10 best practices of reinforcement learning from human feedback (RLHF)

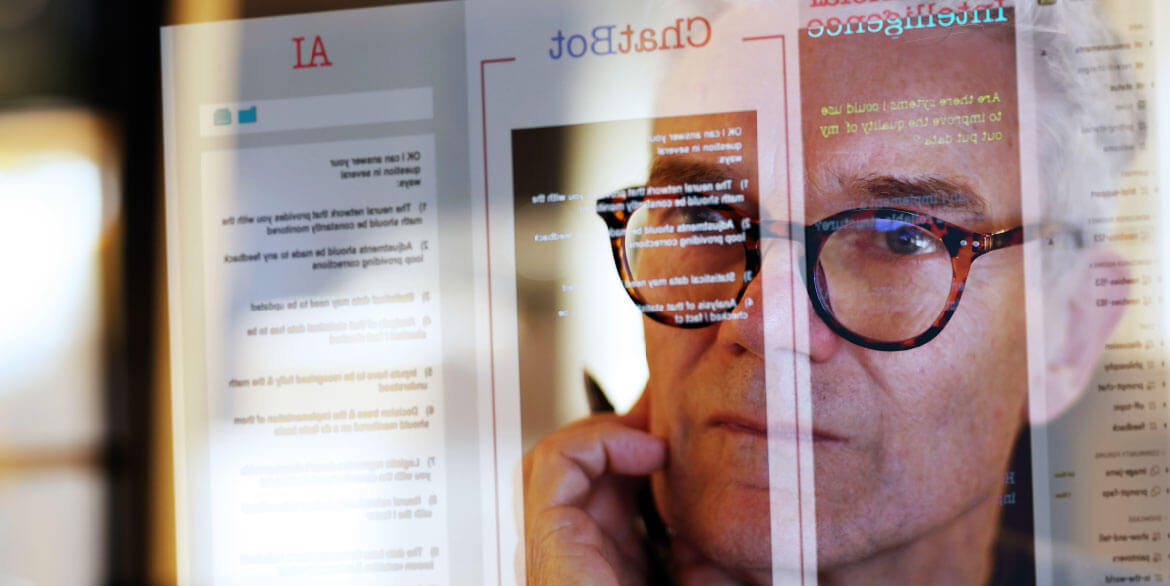
Generative AI models are great at detecting patterns across vast datasets and rapidly generating valuable insights and outputs. But across most use cases, there’s still no replacement for the nuanced expertise and context that humans bring to the table.
Often, the best results come about when generative AI and humans work alongside one another and augment each other’s strengths. That’s where practices like reinforcement learning from human feedback (RLHF) can make all the difference.
RLHF is an approach where generative AI models learn from human feedback on their outputs. Humans validate everything that the model is doing well (or not so well), and the model uses that feedback to continuously generate stronger and more relevant outputs.
However, there are still some key pitfalls to avoid when applying RLHF to fine-tune generative AI. Here are 10 best practices that we follow – and encourage our clients to adhere to – to help generative AI models and human teams get the most from one another:
- Start with the right data: The quality and quantity of data used to train or fine-tune your generative AI model directly impacts its performance. A diverse, representative, high-quality set of training or fine-tuning data can give your model the greatest chance of generating valuable outputs.
- Watch out for bias: The data you use to train and fine-tune your generative AI model can introduce issues such as bias into the model. If the data used to train and fine-tune your model isn’t representative of the users its outputs will serve, the model may exhibit biased behavior, which can lead to unfair or discriminatory outcomes. Remember, biased data going in means biased outputs coming out.
- Take the time to verify data quality: Data that isn’t vetted or sourced responsibly can introduce errors into your model's outcomes. Data preprocessing and cleaning are necessary to ensure data quality. This is also one of your first chances to bring human perspectives and validation into your AI project. Have your data experts take the time to ensure that training or fine-tuning data is of a high enough quality to deliver the accurate and useful outputs and outcomes you’re looking for.
- Augment your data: Augmenting training data by adding variations or synthetic examples can improve model performance and robustness. Techniques like data augmentation can help the model learn from a broader range of scenarios. This works best when you augment natural data collection of real-world, unaltered information with data creation to ensure a robust range of AI training data.
- Right-size your training datasets: In general, larger datasets tend to lead to better model performance – up to a certain point. Beyond that threshold, the benefits of adding more data may diminish, while still increasing your costs. So it’s worth taking the time to consider how much RLHF data your model really needs upfront.
- Manage data distribution: The distribution of generative AI training or fine-tuning data determines the variety and quality of experiences the model will learn from. The distribution of human-provided feedback should match the distribution of the data that the model will encounter in the real world. Mismatches can lead to poor generalization across diverse scenarios. This tends to be one of the hardest practices to implement because you must understand your data to understand if it has the distribution that you need.
- Maximize domain specificity: Models trained on domain-specific data will almost always outperform more general models. If you’re using your model for a domain-specific use case, make sure your training data is highly relevant to the context of that domain.
- Put the right people on the job: When the success of your AI model hinges on human feedback, aligning the right humans with the right expertise to the right tasks is essential. This includes having skilled data collectors, data annotators and domain experts who can effectively contribute to the data preparation and curation process. Misallocation of human resources can negatively impact the quality of generative AI training and fine-tuning data.
- Train the trainers: Training human annotators and data collectors so that they can support others is crucial for achieving high-quality generative AI outputs. Providing timely feedback on the quality of their work and helping them understand inaccuracies or biases in the data they’re producing can lead to continuous improvement in data quality.
- Establish data labeling standards: Establishing clear and consistent data labeling standards is crucial to ensure the accuracy and reliability of your training data. Inconsistent or vague labeling can lead to model errors and misinterpretations of data.