Addressing bias in generative AI starts with training data explainability
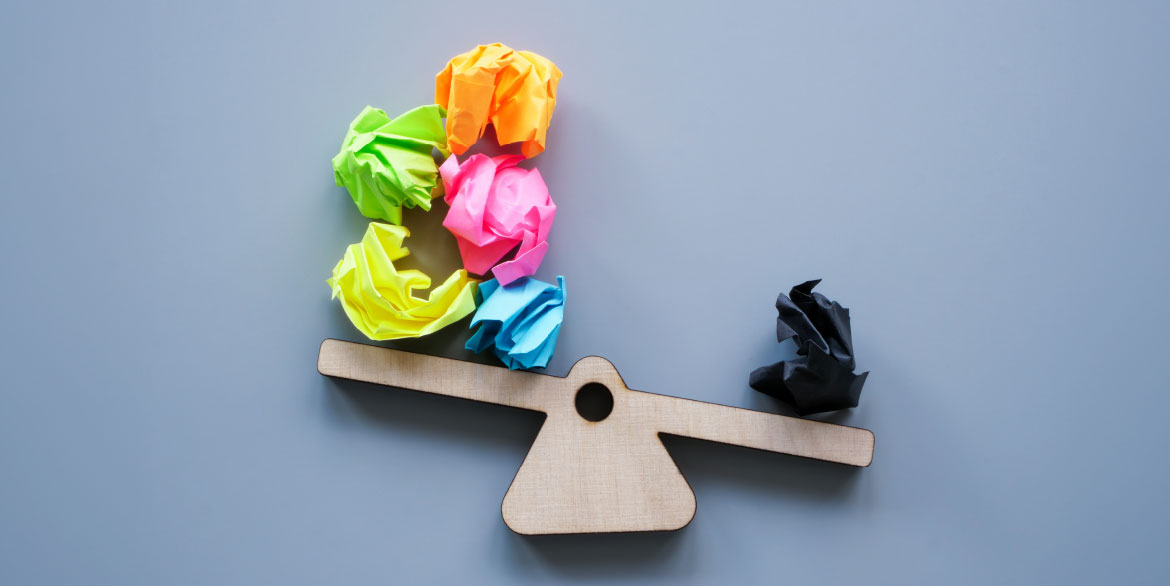
In an age where artificial intelligence (AI) plays an increasingly influential role in our lives, understanding how and why AI models make the decisions they do is paramount. The term "AI explainability" refers to the capacity to unravel the complex inner workings of AI systems and understand and interpret their outputs.
Ensuring the transparency and explainability of the data used to train your generative AI models is the first step toward addressing issues such as AI bias. But understanding how bias can manifest itself in seemingly neutral training data, how biased data can impact users of the model, and how to ensure explainability of AI training data so that issues like bias can be effectively addressed aren’t always easy. Let's dive into these concepts by exploring real-world examples of how bias can creep into generative AI systems undetected.
The power and pitfalls of AI training data
The unintended bias of data workers
Scenario 1: Lack of diversity or representation
Scenario 2: Ambiguous labelling
Scenario 3: Limited perspective
Scenario 4: Reinforcing stereotypes
The ripple effect: How bias trickles down
- Learning biased associations: During model training, the biased training data informs the AI model's understanding of the world. It learns patterns, associations and biases present in the data. This means the model will be more likely to make predictions and produce outputs that align with the biases present in the training data.
- Amplified stereotypes: Subtle biases present in the training data can be amplified during the generative process. The model may not only replicate existing biases but also exaggerate them in generated outputs. This occurs when the model, based on its learned biases, produces outputs that are more extreme or skewed than the input data.
- New bias creation: Bias in training data can lead to the emergence of new, unintended biases in generative AI outputs. As the model learns patterns from the data, it might create associations or infer relationships that were not explicitly present in the training data, but align with the learned biases. This can result in the generation of content with novel biases that were inadvertently introduced during the learning process, further complicating efforts to identify and mitigate bias in the outputs.
Addressing bias through explainability
- Know the source: Make sure you understand where your AI training data comes from and who prepared it. You should know where workers are located and how they will be hired, compensated and trained to minimize the potential for biases to be introduced to training data from the start.
- Ensure diversity and representation: Make sure the users of your generative AI are fully represented in the training data. Hire data workers from diverse backgrounds, locations and perspectives, and train them to recognize and mitigate bias.
- Promote transparency and document everything: Document data curation and labelling processes to identify potential sources of bias. Maintain proper documentation about the data used for AI training and any decisions made during the process to provide transparency into how the data was selected and labelled.
- Continuously monitor and update: Implement continuous monitoring and auditing of AI model outputs for bias and fairness, even after deployment. This allows for the detection of any biases that may have been missed during the training process, enabling updates and improvements to be made as needed.