AI data services: Providers, pros and pitfalls
23 Feb 2024
5 minute read
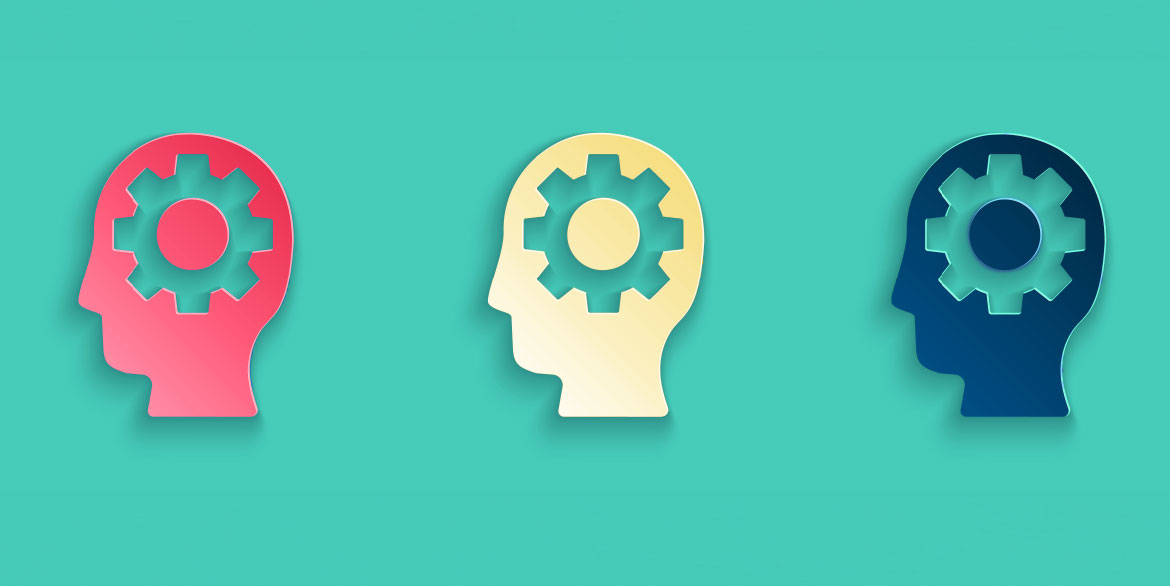
In today's AI-driven world, AI data services have become the lifeblood of successful machine learning projects. Access to high-quality, well-annotated data is essential for training machine learning models and fine-tuning generative AI.
However, not all organizations have the resources or expertise in-house to effectively curate and prepare the data they need to train their AI models. This is where AI data services providers come into play.
In this blog post, we'll explore the different types of AI data services providers in the market today, and discuss the pros and pitfalls of working with each type.
The landscape of AI data services providers
There are several different types of AI data services providers, each with their own unique set of strengths and weaknesses. Here are some of the most common types:
Provider type: Crowdsourcing platforms
These platforms crowdsource small data labelling and annotation tasks to a large, distributed online workforce. They enable organizations to source labour on demand for various data-related tasks to help train and fine-tune AI and machine learning models.
Pros:
- Scalable: Large volumes of data can be annotated quickly, providing scalability for simple data tasks.
- Cost-efficient: Enables basic tasks for small projects to be completed cost-effectively.
Pitfalls:
- Lack of quality control: Little to no vetting or training of workers in the crowd makes it difficult to ensure high-quality data annotations. Also, expertise for more complex data projects is rare.
- Complex project management: Managing a large crowd of workers on a project can be time-consuming, requiring community management expertise that may not be readily available.
Provider type: Data marketplaces
Data marketplaces act as intermediaries between companies in need of training data and individuals or organizations that have datasets to sell. They often offer a curated selection of data compared to crowdsourcing platforms, and they have some quality control measures in place.
Pros:
- Convenient: They provide ready access to pre-existing, curated datasets to train AI models.
- Fast: This approach saves time compared to collecting and annotating data from scratch.
- Cost-effective: Marketplace datasets may be a cost-effective approach to take for some AI data use cases.
Pitfalls:
- Lack of control: With this approach, companies have no control over data quality and no option to customize the data to the specific needs of their AI application.
- Lack of transparency: Marketplace datasets may not provide full visibility into how the data was sourced, which in turn could potentially expose models to flawed data and companies to legal risks.
Provider type: Specialized data labelling companies
Specialized data labelling companies focus exclusively on data labelling and annotation services, often for specific data types (e.g., image, text, audio) and domains (e.g. medical imaging or autonomous vehicles).
Pros:
- Domain expertise: They can provide specialized data annotations for specific domains.
- Quality assurance: They often deliver high-quality annotations with robust quality control processes for complex data types.
Pitfalls:
- Higher cost: Specialized data is often pricier, compared to other options like crowdsourcing.
- Extended turnaround times: Specialized tasks typically take longer to complete.
- Limited service scope: These providers may not offer the full range of AI data services required on an AI data project.
Provider type: Full-service AI data providers
Full-service AI data providers, like TrainAI from RWS, offer complete, end-to-end AI data solutions, encompassing data collection, data annotation or labelling, data validation and project management services.
They typically have dedicated teams of data and quality assurance experts to ensure the accuracy of the AI training data they deliver. This makes them an ideal choice for preparing the data needed to train machine learning models and fine-tune generative AI.
Pros:
- End-to-end support: Complete AI data services – from data collection and annotation to data cleaning and project management – streamline the data preparation process, ensuring consistency and quality of AI training data.
- Bespoke solutions: These providers are often flexible and can tailor their services to meet your unique AI data project requirements, whether you need image recognition, natural language processing (NLP) or other AI data capabilities.
- Expertise and quality control: They typically employ data services experts with relevant industry experience who understand the nuances of AI data and have dedicated quality assurance teams who apply rigorous quality control processes on AI training data projects.
- Scalable: They can often handle large-scale, complex, mission-critical projects.
- Procurement and cost efficiency: Some full-service AI data providers, like TrainAI, provide complementary services such as language support, domain expertise and AI data strategy consulting, enabling companies to leverage volume discounts and optimize vendor spend across services.
Pitfalls:
- Higher up-front investment: Working with a full-service provider may require a higher initial investment due to the comprehensive range of services offered – but it’s a valuable investment in long-term AI project success. Their expertise ensures the quality, consistency and dependability of AI training data from the beginning, eliminating the need to redo AI data at an additional cost due to quality issues.
Find the right AI data services provider today
AI data services are a critical component of machine learning projects and choosing the right provider is essential to project success. While there are various types of providers available, full-service providers like TrainAI offer a one-stop solution that ensures training data quality, expertise and cost-efficiency.
By partnering with a full-service data provider, you can navigate the complexities of AI training data preparation with confidence, ultimately accelerating the development and deployment of your AI solutions.
Evaluating AI data vendors to train or fine-tune your generative AI? Download our checklist to evaluate AI data services providers and get your project off to the right start.