Determining what AI data you need and how to source it
08 Apr 2024
10 minute read

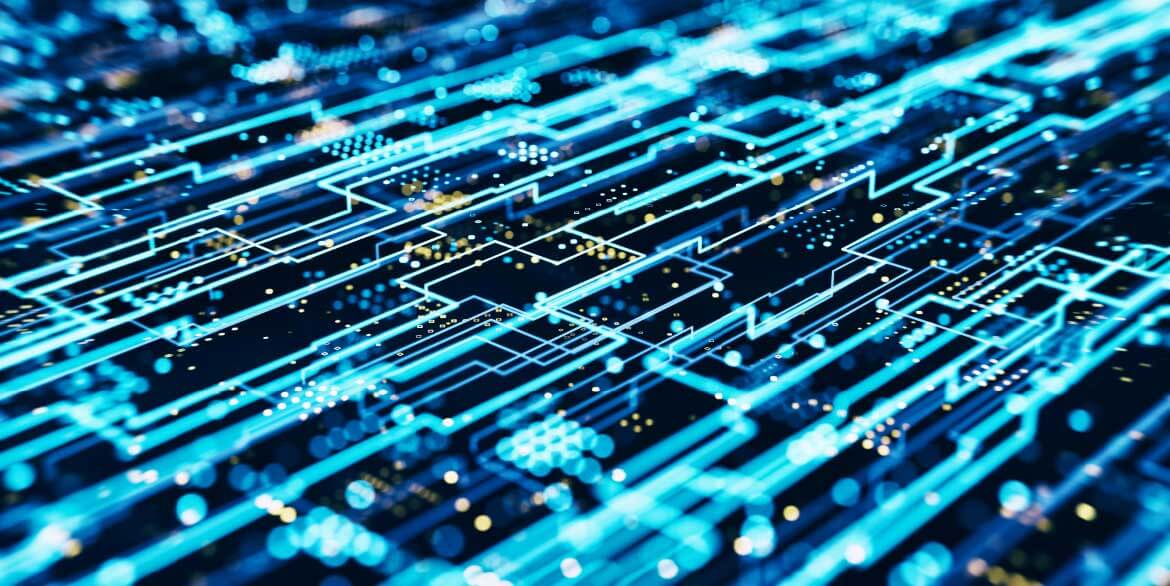
With the evolution of artificial intelligence (AI) technologies, more and more businesses are turning to AI solutions to solve complex problems and optimize their operations. However, one critical factor in successfully implementing AI is having high-quality data. Without it, even the most advanced algorithms will struggle to produce accurate results.
But how do you determine what AI data you need and how to source it?
Getting started: Identifying the AI data you need
Before you can start sourcing data, you first need to clearly understand the type of data your AI project requires. Here are some steps you can take to identify the data you'll need to train or fine-tune your AI model:
- Define your project goals: The first step in any AI project is to clearly define your goals, which includes understanding the challenges you want to solve with your AI model. Your goals will guide the type of model you choose to build, which in turn will determine the type of data you'll need.
- Consider the model's AI data requirements: Think about the type of AI model you're building and the data it needs. This can include structured data (numerical, categorical or tabular data), unstructured data (text, images, audio or video data) or a combination of both.
- Assess your data availability: After defining your data requirements, evaluate if you already have access to the necessary data. This could be data generated internally by your business operations or data from previous projects. If your existing data is insufficient or irrelevant, you’ll need to consider sourcing or collecting new data.
- Consider data quality and quantity: Both data quality and quantity are crucial to the success of your AI project. You'll need data that’s not only relevant, accurate and representative of the problem you're trying to solve and the user groups for your model, but also sufficient in quantity to ensure reliable results.
- Prioritize data security and privacy: In the quest for the right data, never lose sight of data privacy and security. Make sure any data you source is obtained legally and ethically, and that you comply with all applicable data protection and privacy laws. This includes securing the data through encryption or other means to prevent unauthorized access. If you're working with sensitive information – such as medical or financial data – you might need to anonymize it to protect the privacy of individuals. Always consider the implications of data storage and transfer, particularly if your data crosses borders, as different countries have their own data protection laws.
- Factor in your budget: Sourcing high-quality AI training data can be costly. If your budget is low, you might have to opt for freely available datasets which may require more preprocessing to be suitable for your specific use case. On the other hand, if your budget is higher, you might be able to purchase tailored datasets tailored to your specific project needs that save time and resources in the long run. Depending on your budget, you may need to strike a balance between quality, quantity and cost.
Once you've identified what data you need, you can then start to think about how to source it.
Sourcing AI training data
When it comes to sourcing AI data, there are several options available. Here are some of the most common methods for obtaining quality training data:
In-house AI data preparation
This approach involves collecting and labeling your own data. It’ll require a greater investment of time and cost, but taking control of the quality and relevance of data is worthwhile, as it ensures the data meets your specific needs and standards and can lead to more successful outcomes.
The advantages of in-house AI data preparation include:
- Control over quality: Collecting your own data gives you complete control over its quality. You can ensure the data is clean, accurate, and relevant to your specific needs.
- Data exclusivity: The data you collect is exclusive to your organization, giving you a competitive advantage as others wouldn’t have access to the same insights.
- Data protection: By collecting your own data, you avoid the legal and ethical issues that can arise from sourcing data externally.
However, in-house AI data preparation also has its disadvantages:
- Time-consuming: Collecting and preparing your own data can be a lengthy process, particularly when it requires manual labeling for AI training.
- Resource-intensive: This approach requires significant internal resources, including the right tools and skilled personnel, which might not be readily available. It can also be more costly.
- Bias risk: If in-house data preparation isn’t carefully managed, it can produce biased data, which can affect the accuracy of your AI model's predictions.
Publicly available datasets
There are many online repositories that offer free datasets for AI training. However, the quality and relevance of these datasets can vary widely.
The advantages of using publicly available datasets include:
- Cost-effectiveness: Using public datasets is generally free or relatively cheap, reducing the financial burden associated with data collection.
- Time-savings: These datasets are ready to use, eliminating the need for a time-consuming data collection process.
- Diversity: Public datasets often come from a wide range of sources, offering a diverse range of data points that can improve your model's robustness.
However, using publicly available datasets also has its disadvantages:
- Relevance: The available datasets might not align perfectly with your specific project requirements, leading to less accurate results.
- Quality control: The quality of public datasets can vary significantly, and poor-quality data can negatively impact the performance of your AI model.
- Lack of exclusivity: Since these datasets are available to everyone, your competitors might have access to the same data, potentially reducing your competitive advantage.
Web scraping
Web scraping involves extracting large amounts of information from websites, which can then be used for AI model training. Using web scraping tools, you can automate the process and gather data from various web sources relatively quickly.
The advantages of web scraping for AI data sourcing include:
- Access to information: The internet is a vast reservoir of data, potentially providing a huge volume of relevant and diverse data for training your AI models.
- Cost-effectiveness: Web scraping can be a cost-effective method of sourcing data, especially when compared to manual or in-house data collection.
- Flexibility: With web scraping, you can be very specific and creative about where and how you source your data, allowing you to tailor the process to your project's specific needs.
However, web scraping also has its disadvantages:
- Legal and ethical considerations: Not all web data is free to use. Some websites prohibit web scraping in their terms of service, and there are legal and ethical considerations you must be aware of. Always ensure you have permission from the copyright holder to use the data you scrape.
- Quality and relevance issues: Web data can vary greatly in quality and relevance. Cleaning and preparing the data for training can be a significant task.
- Maintenance: Websites change and update frequently, so a web scraping setup may require regular maintenance to ensure it continues to function and provide the data you need.
Crowdsourcing
Crowdsourcing is an approach that involves obtaining data from a large group of people, typically via the internet. Crowdsourcing can be a cost-effective way to gather a large quantity of diverse data, although the quality of the data can vary greatly.
The advantages of crowdsourcing your data include:
- Cost-effectiveness: Crowdsourcing can often be cheaper than other methods of data collection, particularly when you require large volumes of data.
- Timeliness: Speed can be a significant advantage, as many individuals contributing simultaneously can gather data more quickly than a single entity.
However, crowdsourcing also has its disadvantages:
- Managing a crowd: Effectively coordinating a large group of contributors can be complex and time-consuming.
- Quality control: Managing the quality of crowdsourced data can be challenging, as it comes from many sources with varying levels of accuracy and reliability.
- Data privacy and protection: Ensuring that crowdsourced data is collected ethically and legally can be complex, as the data may come from different jurisdictions with different data protection laws.
- Data relevance: While crowdsourcing can provide a large quantity of data, it might not always provide the specific kind of data prepared by the diverse and representative community that your AI project requires.
Data marketplaces
Data marketplaces provide a 'one-stop shop' for your data needs, offering a wide variety of datasets from different providers across areas such as consumer behavior, financial markets, healthcare and more. They allow you to browse and purchase the specific type of data you need for your AI project.
The advantages of using data marketplaces include:
- Variety: A data marketplace offers a broad selection of datasets that cater to a wide array of industries and domains. This allows you to choose the most relevant dataset for your AI project.
- Time-savings: These pre-packaged datasets save you the time and effort involved in collecting and preparing data.
- Quality assurance: Many data marketplaces vet their data providers and provide some level of quality assurance to reduce the risk of inaccurate or unreliable data.
However, using data marketplaces also has its disadvantages:
- Cost: Commercial datasets can be expensive and might not meet your specific AI training data requirements.
- Data relevancy: While there may be a wide range of datasets to choose from, finding the one that fits your specific project needs can still be challenging.
- Data privacy concerns: Depending on the nature and source of the data, there may be privacy and legal implications to consider when purchasing and using these datasets. It's crucial to ensure that the data complies with all relevant data protection and privacy laws.
- Data quality issues: While many reputable data marketplaces vet their data providers and offer certain guarantees of quality, standards can vary depending on the marketplace and the specific vendor or dataset. Therefore, it's crucial to do due diligence before purchasing a dataset.
AI data services providers
Full-service AI data services providers, like TrainAI from RWS, deliver data that has been meticulously collected, cleaned, labeled and validated to match your specific AI training data needs.
The advantages of using all-inclusive AI data services providers include:
- Customization: They often offer tailored datasets tailored to your specific requirements, ensuring the data you receive is relevant to your AI project.
- High quality: As these organizations specialize in data collection and preparation, they usually provide high-quality, clean and accurately labeled data.
- Time-savings: The data is prepared specifically for your AI model and comes ready-to-use, significantly reducing the time you spend collecting and preparing the data.
However, using AI data services providers also has potential disadvantages:
- Cost: This method can cost more since it involves customization and high-quality data preparation to meet your specific project needs. However, this cost may be considered an investment in model success, since spending more upfront to source the right training data for your AI model eliminates any need to redo the data later on, resulting in long-term savings.
- Privacy concerns: Just like data from marketplaces, data from paid sources also comes with potential privacy and legal implications. Explainability and transparency are key. Your AI data services provider should document where and how data is being sourced to ensure compliance with all relevant data protection laws.
The best approach for sourcing AI training data depends on your specific needs, resources and capabilities. A clear understanding of your AI project's requirements – combined with a thoughtful and well-planned data sourcing strategy – can significantly enhance the effectiveness and success of your AI model.
Not sure how to source the AI data you need to train and fine-tune your AI model? Download our AI data sourcing decision tree to get started.