Diary of an AI data annotator: Daily challenges and triumphs
08 Mar 2024
6 minute read
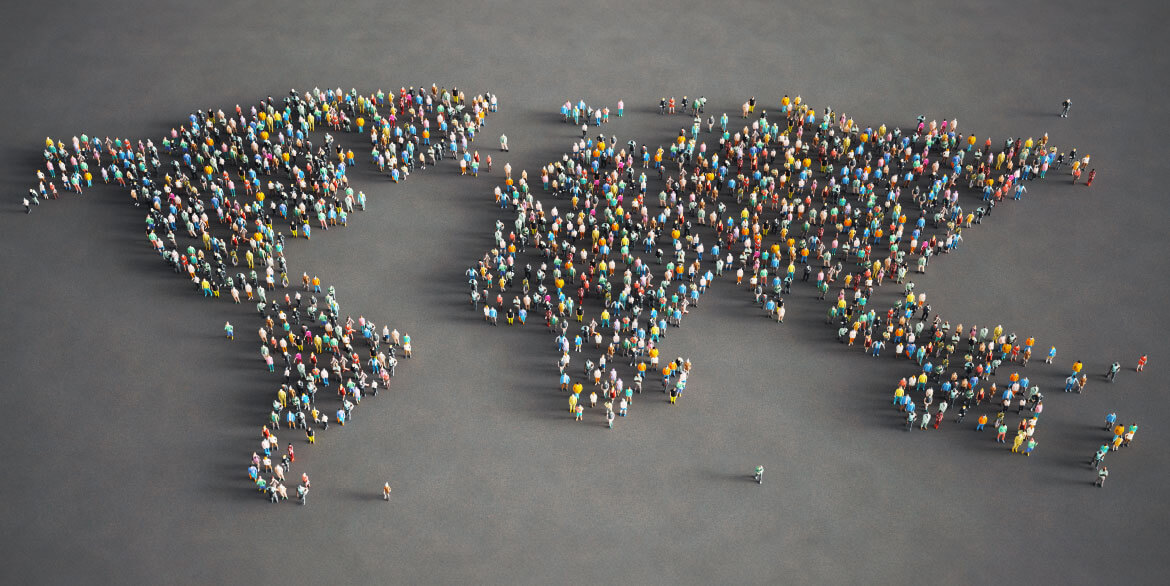
Growing up in rainy Oregon, I was never too far from the technology industry. That, along with a natural interest in the field, is what led me to complete a bachelor’s degree in physics and a master’s degree in computer science.
However, despite all my connections to Silicon Valley, I am still a big fan of being in nature and taking advantage of all the outdoor activities Oregon has to offer. When I’m not outdoors, a lot of my time is spent working as an AI data annotator! During work I spend most of my time checking and working on the content generated by AI models, communicating with my team, helping teammates and managing the tracking and analysis of jobs that my team is working on which can have different types of issues.
Getting started in the role
When I first started working in this role, I was fortunate to have a smooth and well-organized onboarding experience. The hardest part was installing some of the applications! But I received plenty of instruction documents and help to get all the administrative pieces done. And then I went through a great training experience with other AI data annotators which included thorough presentations, formal guidelines and practice work that helped us feel prepared to start working.
My daily routine as an AI data annotator
A typical day in the life of an AI data annotator can be quite eventful. The first step is always checking emails and team chats to see if there have been any updates from the client about new tasks coming in, or if the requirements for the current work we’re doing have changed.
If something new has come in, it means it’s time for a deep dive into the new guidelines. The instructions are often lengthy, so it can take a lot of time to sort through all the details. And they always require multiple reads until you feel like you understand what the client wants done and how to do it. However, just like in school when sometimes a new concept seems simple when explained in the textbook but ends up being difficult once you start doing it, the same is true for data annotation tasks. They might seem straightforward at first, but once you actually start the work, the questions and ambiguities start rolling in!
If nothing new has come in, the next step of the day is to review all the team chats to see if any new issues or questions from my teammates that I need to know about have popped up. It can be challenging when questions come up because there’s often a delay between the question being asked and actually getting a response from the client. So, it’s important to keep track of the questions while waiting for clarification. And if I know the answer to any questions or have suggestions, I make sure to reply in the chats to help my teammates out.
Once I’m up to speed on any updates or questions that have come up, it’s time to get going on the tasks themselves. As soon as I get a task, I go over the data with a fine-toothed comb, making sure it meets every requirement the client has specified. Maintaining a balance between avoiding being overly granular while still meeting all the client’s requirements is a constant tightrope walk. This can certainly be difficult with so many tasks, but once you get going and get into the zone it gets much easier.
Typical tasks and team support
The types of tasks I’ve worked on have varied, but one example would be doing quality analysis on the text generated by AI models. This requires making sure the text makes sense to a human reader, has the appropriate style and format, matches any client-provided requirements and has proper grammar, punctuation and so on. If any issues or ambiguities pop up, I flag the task to make sure everything is cleared up before sending it along to the next stage in the data preparation process. And throughout the day I periodically check team chats to stay up to date.
When issues do come up during work, I have the benefit of being supported by the TrainAI team. They are great at quickly responding to our questions or thoughts. And if they don’t have the answer, they make sure to pass the question along to the client to get an answer for us. Also, they lighten up our work atmosphere by having fun in the chat when something entertaining happens at work. And when issues pop up in our personal lives, they are extremely understanding and do everything they can to help.
Client wish list
When it comes to working with clients, my experience has been extremely positive. But if I were offered a wish list of things clients could do differently, there would be a few things I would ask. For example, it would be wonderful to have some more time to meet with clients to discuss difficult tasks or edge cases. And although the applications we use to do our work are excellent, some modifications to make them a little more user-friendly would help make everything go even smoother. And finally, in our line of work, there’s no such thing as too much feedback – the more the better!
Final thoughts
As someone who has a major interest in AI and studied it in school, working as an AI data annotator has been so much fun! It’s been amazing to see this process of the AI pipeline, which I hadn’t seen before, and it has given me a new understanding of how AI models behave in practice. And all my wonderful teammates make it even more enjoyable – we’re never short on shared laughs! Lastly, having a flexible schedule combined with working remotely has been great as well – it has allowed me to spend more time with family and friends.
Despite all the challenges that arise in the daily life of an AI data annotator, it’s exciting to be part of work that’s driving the future of AI forward. Although it may not be as flashy as designing or training new AI models, it’s the important behind-the-scenes work that must be done before we can achieve the progress that we’re striving for.
Planning a generative AI project? Download our generative AI decision roadmap to understand key decisions you should make upfront to ensure project success.