Dos and don’ts when fine-tuning generative AI models
29 Jan 2024
4 minute read

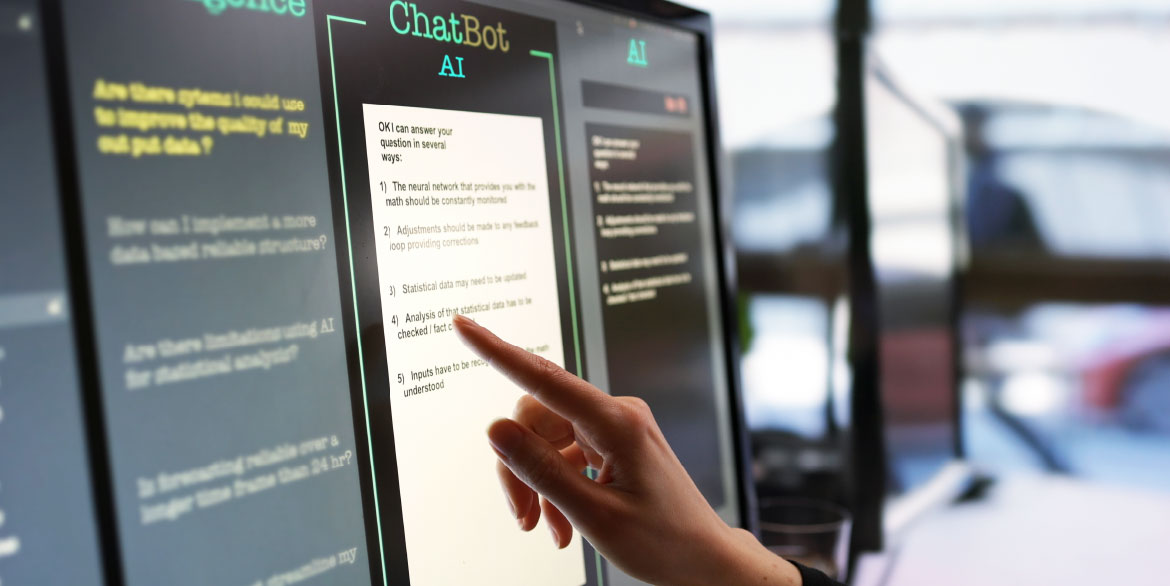
Fine-tuning generative AI models presents a unique set of complexities and challenges. Throughout the process teams must delicately adjust parameters, tweak architectures, curate AI training data and optimize models based on user feedback, all while staying focused on the ultimate goal – generating useful and meaningful outputs.
TrainAI works with many different organizations and content types, which enables us to anticipate potential challenges across the fine-tuning process before they happen and advise our clients on how best to avoid them. In this blog post, we'll explore some important dos and don’ts when training and fine-tuning generative AI models, drawn from TrainAI’s own diverse project experience.
The dos
1. Understand your data
DO: Start by gaining a deep understanding of your AI training data and where it comes from. Be confident it was collected or created in an ethical and secure manner.
DO: Invest time in AI data preprocessing and cleaning. This will ensure that your model doesn’t inadvertently learn and propagate harmful or incorrect information.
2. Spend time developing thorough content guidelines
DO: Develop and adhere to ethical guidelines for content generation. Clearly define what is acceptable and unacceptable content for your specific generative AI project.
DO: Regularly review and update your content guidelines in line with today’s ever-evolving AI environment as well as the latest data- and AI-related regulations such as the EU AI Act and GDPR (General Data Protection Regulation).
3. Continuously evaluate model outputs
DO: Implement a feedback loop to evaluate the quality and appropriateness of generated content. Regularly involve human reviewers to provide your generative AI model with guidance and feedback that it can learn from.
DO: Use metrics that are specific to your generative AI project goals, such as relevance and coherence.
4. Mitigate bias
DO: Prioritize fairness and inclusivity in content generation, and strive to minimize any potential type of discrimination or harm.
5. Maintain documentation and transparency
DO: Maintain up-to-date documentation about your generative AI model and its fine-tuning processes, including its model architecture and training data sources.
DO: Be transparent about your AI system's limitations, and clearly communicate that its outputs are machine-generated.
The don'ts
1. Overlook data biases
DON'T: Assume that your training data is bias-free. Failing to address biases can result in discriminatory or offensive content generation.
2. Rely solely on automated metrics
DON'T: Depend solely on automated metrics for evaluating generative AI model performance. They may not capture the nuances of content quality and ethical considerations. Consider ways that you can independently assess the quality of your outputs.
3. Skip regular human reviews
DON'T: Neglect the importance of regular human reviews. Human reviewers play a vital role in fine-tuning generative AI systems and ensuring that they generate ethical content outputs.
DON’T: Miss the opportunity to talk to your AI training data team about any trends they’re seeing in data collection, content generation and the training or fine-tuning of generative AI models. These easy conversations can lead to early engineering process modifications that help you avoid potential issues before they arise.
4. Provide overcomplicated guidelines
DON’T: Create overly complex or excessively lengthy project or task guidelines that increase the risks of misinterpretation and inconsistencies in model fine-tuning. Doing so can add unnecessary time and complexity to your fine-tuning project, since your project guidelines must first be converted into a format that can be easily understood by human raters. To address this you should:
- Simplify and clarify: Condense project or task guidelines into concise, easy-to-follow instructions. Use clear language and provide illustrative examples where necessary.
- Train and solicit feedback: Train raters effectively, providing them with ample opportunities to ask questions and seek clarification on your guidelines. Encourage feedback and adapt your guidelines based on raters' input.
- Iterate: Continuously refine project or task guidelines based on feedback and learnings from the generative AI fine-tuning process.
In conclusion, fine-tuning generative AI models is a delicate balancing act. Adhering to the dos and avoiding the don'ts, while also ensuring that project guidelines are easily understandable, is crucial to harnessing the full potential of your generative AI in a responsible manner.
Planning a generative AI project? Download our generative AI decision roadmap to understand key decisions you should make upfront to ensure project success.