Futureproof your generative AI with human intelligence
29 Jan 2024
8 minute read
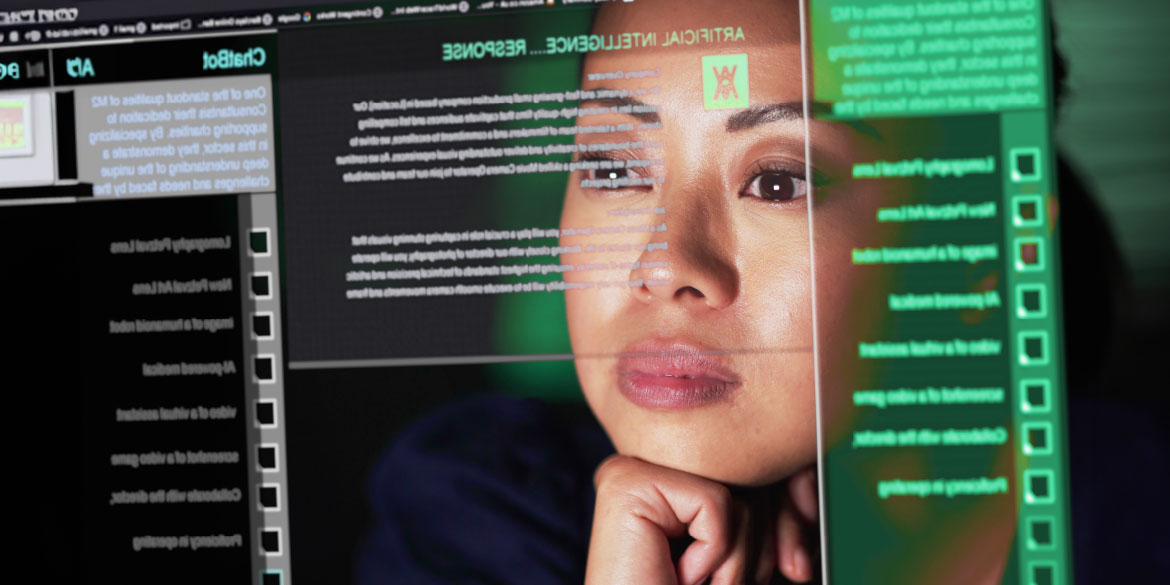
Generative AI models are rapidly evolving into powerful tools for generating ideas and solving problems. However, as we continue to push the boundaries of what AI can do, it’s also important to consider these models’ long-term viability. That's where human intelligence comes into play, ensuring your generative AI technologies offer value for years to come.
Here are six strategies you can implement to help futureproof your generative AI with human intelligence.
Fine-tune using reinforcement learning from human feedback
Many of the generative AI models available today were trained on data from the internet. But in addition to containing useful and factual content, the internet is also a source of fabricated, biased and potentially harmful content.
One way to address potentially flawed AI training data is to employ a model fine-tuning technique known as reinforcement learning from human feedback (RLHF). This process involves using humans to provide feedback and guidance for the AI model, allowing it to continuously improve and adapt based on real-world interactions.
There are many different types of RLHF tasks that humans can complete on a continuous basis to enhance the performance of generative AI models, including:
- Data annotation: Tagging and categorizing data to help the model understand context and improve its prediction capabilities.
- Prompt engineering: Crafting specific instructions and prompt-response pairs to guide the behaviour of the model, helping it generate more accurate and contextually relevant content.
- Quality assessment: Evaluating responses or outputs of the AI model by rating their quality and relevance, feedback that can then be incorporated into the model to help it learn from its mistakes and improve over time.
- Interactive tuning: Engaging in an interactive dialogue with the model, providing real-time human interaction, feedback and corrections, which are then integrated into the model to optimize its performance.
- Red teaming or jailbreaking: Intentionally attempting to find and exploit vulnerabilities in the AI model by, for example, inputting unusual prompts, probing for bias in responses or prompting with the intention of using the system in unintended ways, feedback from which can be used to boost the robustness of the AI.
The integration of these human-centric tasks not only enhances the capabilities of generative AI models but also ensures their long-term sustainability and relevance.
Leverage domain expertise
Another way to futureproof your generative AI is by enhancing it with domain expertise. This involves working with experts in specific fields such as business, law, medicine and more, to fine-tune the model for specialized topic areas and tasks. By incorporating expert knowledge and insights into the training process, you can create a more robust and accurate model tailored to a specific domain or industry. This not only helps improve the overall performance of the AI, but also makes it more adaptable to changes within that domain.
Leveraging domain expertise to fine-tune generative AI models can be accomplished through:
- Curated datasets: Domain experts create carefully curated, domain-specific datasets based on expert knowledge and understanding of the field to provide the AI with highly relevant and accurate training material.
- Knowledge transfer: Experts provide the AI with feedback on its performance for a specific domain, helping it to learn and improve.
- Model validation: Subject matter experts validate the responses from an AI model by reviewing and providing feedback on the AI's conclusions, ensuring that its outputs align with the current understanding in the field and improving its accuracy and reliability.
By effectively applying domain expertise in these ways, you can enhance the ability of your generative AI model to perform specialized tasks, increasing its value and utility in the long run.
Make it global
Locale-specific understanding is a crucial aspect of generative AI. Geographically tailoring your model to different cultures and languages can greatly expand its global reach as well as its potential applications. By incorporating location-dependent knowledge into the training data, your AI model will learn to generate outputs in different languages for different cultural sensitivities, making it more versatile and useful for a global audience.
There are several ways in which human intelligence can be harnessed to infuse generative AI with locale-specific capabilities:
- Data annotation: Native language speakers annotate multilingual datasets to help the model understand the context and nuances of different languages and cultures, enabling it to generate relevant content in those languages, for those cultures.
- Translation and localization: Linguists translate and localize content to be used as multilingual training data, so the model can learn to generate content that’s culturally appropriate and accurate for specific locales.
- Quality assessment: Local experts assess the quality and accuracy of the AI’s output for different regions and languages, providing crucial feedback that can be used to improve the model's performance.
- Interactive tuning: Multilingual speakers engage in an interactive dialogue with the model in various languages, providing real-time feedback and corrections that can be integrated into the model to enhance its outputs for different locations.
By incorporating locale-specific, human-driven tasks into the development of your generative AI, you can ensure its continued relevance and value on a global stage in an increasingly interconnected world.
Prioritize privacy and security
In the era of big data, it’s paramount that we maintain the security and privacy of sensitive enterprise and client information. For generative AI, it's crucial to incorporate measures that safeguard both the data used in model training and any data the model interacts with post-deployment.
Here are a few strategies you can implement to protect the confidentiality of enterprise and client data:
- Encryption protocols: Employ robust encryption protocols for data at rest and in transit, ensure secure access control and conduct regular security audits to protect your AI system from potential threats and vulnerabilities.
- Differential privacy: Introduce a controlled amount of noise to the data, making it statistically impossible to reverse-engineer the original inputs, which preserves privacy while allowing the model to learn from the data.
- Federated learning: Enable the model to learn from decentralized data sources, ensuring that the raw data never leaves its original location, thus enhancing data privacy.
- Security protocol assessments: Regularly review and update your security practices in response to evolving regulations to ensure your generative AI model remains compliant and secure, promising its long-term viability.
By taking a privacy- and security-first approach, you not only safeguard your generative AI from potential threats but also ensure its long-term viability and trustworthiness.
Adapt to AI regulations
With the rapid development of AI technology, governments are introducing regulations and guidelines to ensure ethical and responsible use of AI. It’s important for generative AI developers to anticipate and adapt to these regulations to ensure the long-term viability of their models. This may involve adjusting or modifying the training process or output generation techniques to ensure compliance.
Below are some key regulations and guidelines that should be on your radar:
- GDPR (General Data Protection Regulation): This European Union law regulates how personal data can be collected, stored and used. To comply, ensure your training data is anonymized, and that you obtain necessary copyright and permissions for data usage.
- The EU AI Act: This act regulates AI systems according to their level of risk to fundamental rights. To comply, ensure transparency, provide clear user information and address safety risks in your generative AI. You must also implement safeguards, conduct risk assessments and follow specific requirements outlined in the act to protect fundamental human rights.
- Children's Online Privacy Protection Act (COPPA): If your generative AI interacts with children under the age of 13, you will need to comply with COPPA by obtaining parental consent and protecting children's privacy.
- Biometric Information Privacy Act (BIPA): If your AI involves biometric data, ensure you comply with BIPA by obtaining explicit consent and securely storing such data.
- Federal Trade Commission (FTC) Guidelines for AI: These guidelines suggest fairness and non-discrimination in using AI. Make sure your generative AI model does not exhibit bias or discrimination by defining ethical guidelines and oversight, implementing and monitoring fairness metrics and using diverse and representative training data during model development. You should perform regular evaluations for bias and employ a system to collect user feedback and perform continuous improvement.
By staying abreast of these regulations and future regulatory changes, you can make necessary adjustments to your generative AI to ensure its continued viability.
Continually source training data
As with any AI model, a reliable source of training data is crucial for its success. However, as your generative AI continues to evolve and generate outputs, you may face the challenge of running out of high-quality training data to enhance its performance. Therefore, it’s important to have a strategy for sourcing data to ensure that your model can continue to learn and improve over time.
Here are some strategies for acquiring reliable training data on an ongoing basis:
- Collaborate with subject matter experts: Partnering with experts in the field related to your generative AI can provide you with access to high-quality, specialized data that’s relevant and valuable for your model.
- Obtain user-generated data: Encourage users of your generative AI to contribute their own data or content, which can then be used as training data for future iterations of the model.
- Leverage public, open-source or commercial datasets: There are numerous AI training datasets and data marketplaces powered by human intelligence that can supplement your training data and enhance the diversity and accuracy of your generative AI.
- Use data augmentation techniques: By applying data augmentation techniques such as mirroring, scaling or cropping, you can generate new variations of existing human-generated training data which can add more depth and variety to your model's knowledge base.
By employing strategic data sourcing methods, you can ensure that your generative AI has a continuous supply of quality training data from which it can learn and improve.
As the field of generative AI continues to grow and evolve, it’s important for creators and developers to take specific steps such as these to future-proof generative AI models. Keeping human intelligence at the core of your generative AI strategy will ensure its continued success and adaptability in today's rapidly evolving world.
Planning a generative AI project? Download our generative AI decision roadmap to understand key decisions you should make upfront to ensure project success.