Mitigating generative AI risks: The strategic role of data services providers
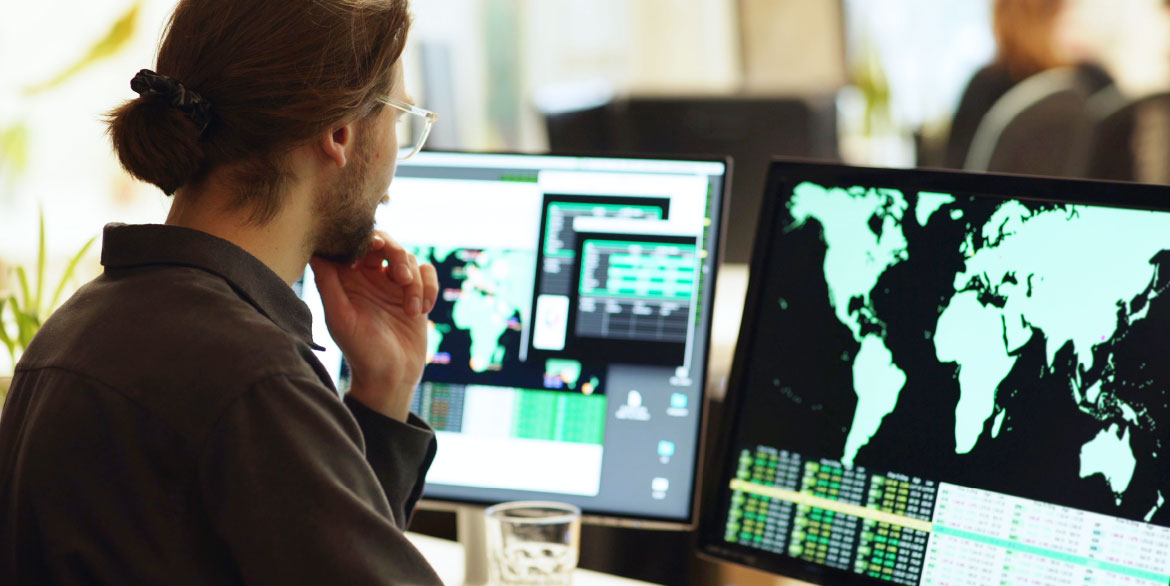
Generative AI applications are being rolled out to consumers at such a rapid pace that many fail to realize the potential risks they come with. Risks such as bias, hallucinations, misinformation, factual inaccuracies, toxic language and more all frequently appear in one form or another in today’s generative AI systems.
To avoid these risks you need a complete understanding of the data used to train generative AI. It isn’t enough to simply know the source of training data. You also need a clear understanding of what’s been done to the data to prepare it for training, who has touched it, the work they’ve done on it, inherent biases they might have, how they were compensated and how quickly any risks you identify can be resolved.
Not considering the potential risks that can be introduced at each step of the AI building process can lead to disastrous results down the road. Here are just a few ways that your AI data services provider can help mitigate potential risks as you build, implement and optimize your generative AI.
Ensuring AI data explainability
Making AI explainable starts with its training data. At the root of the data, and sprinkled throughout its journey to your model, are humans – with all their flaws and biases. Your AI data services provider should not only recognize these flaws and biases, but also understand what strategies can be implemented to overcome them.
As their client, it’s important that you also understand how the data services process works. If you require data to be collected, you should know exactly where the data will come from and who will provide it. You should feel comfortable that the workers preparing your data will be paid fairly and treated well, not only because it’s the ethical and right thing to do, but also because compensation and treatment impacts work quality. Finally, you should understand how they will perform their tasks to help identify and minimize the potential for risks to be introduced. This knowledge will contribute significantly to ensuring that your generative AI model is explainable.
Recruiting with diversity and inclusion in mind
- Demographic factors such as age, gender and occupation
- Geographic factors such as location, culture and language
- Psychographic factors such as lifestyle (e.g. parent, student or retiree), interests and domain speciality or expertise
Providing scalability of resources
Uncovering and addressing hallucinations or bias in your generative AI model requires the ability to pull together communities of resources to solve these problems quickly. If you discover that your model fails to support a given region of the world, you’ll need people from that region assembled, trained and ready to help you resolve that issue. It’s important to understand what resources your AI data services provider has available today to ensure they can meet your needs.
Training and fine-tuning generative AI applications often require resources with increasingly specific areas of expertise. Understanding how quickly your data services provider can source, recruit and scale new communities is equally as important (and in some cases more important) as the resources they have available in their community today.
Offering ongoing resource training and support
Recruiting and sourcing the appropriate resources is one challenge, but getting those resources up to speed and performing at a high level is another. As a client, it’s important to remember that on the receiving end of any instructions or guidelines you provide is a person, sitting at a desk, reading them from start to finish, just trying to understand what you expect of them.
One of the most common mistakes we see clients make when working with an AI data services provider is how instructions and guidelines are communicated to workers. In some cases, instructions and guidelines can be as lengthy as 100 or more pages. If instructions aren’t transformed into a clear format that everyone working on the project can understand, you’ll quickly run into quality issues and costly redos.
Your data services provider’s ability to take lengthy and complex guidelines and transform them into easily digestible training for newly onboarded resources is critical to success. Their ability to provide ongoing, responsive support to the community of workers preparing your AI training data is also important. Make sure you’re satisfied with your AI data services provider’s training and support plan to ensure a successful outcome for your generative AI training and fine-tuning project.
Achieving success in your generative AI training or fine-tuning efforts depends heavily on the quality of your AI training data. Partner with an AI data services provider that values explainability, diversity, scalability and support, so that you’re better positioned to mitigate potential risks and create high-performing generative AI applications that resonate with your users.
Evaluating AI data vendors to train or fine-tune your generative AI? Download our checklist to evaluate AI data services providers and get your project off to the right start.