Navigating a single or multi-vendor AI data services partner strategy
23 Feb 2024
9 minute read
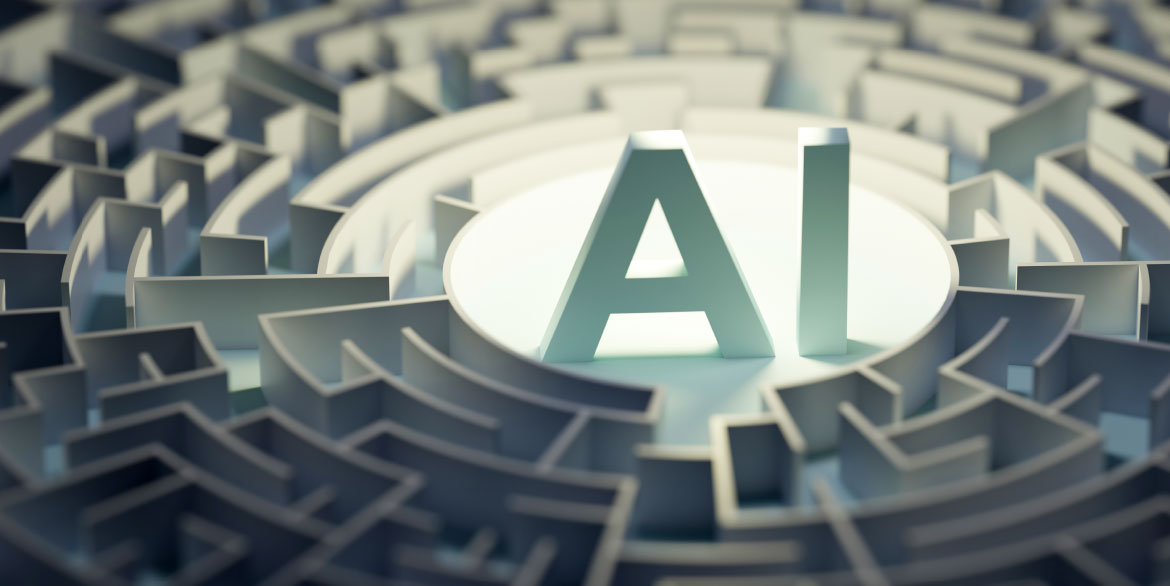
The first step towards building a successful AI data services partnership with one or more vendors is clearly defining your goals and needs. Different model types and use cases require different capabilities – which will impact your choice of partner or partners, and how you ultimately work together.
The more you can define upfront, the better positioned you’ll be to navigate a long and successful partnership with your chosen vendor – or vendors.
In this blog I’ll walk you through some of the key considerations your teams should make when deciding between a single or multi-vendor AI data services partner strategy to support your use cases.
Considerations for a single-vendor AI data services partner strategy
Before selection: Defining your needs
Before pursuing a single-vendor partner strategy, consider the following variables to ensure you make the most appropriate selection for your business and your needs:
Who’s going to be involved?
Consider who will be involved in your AI projects, what’s important to them and how they measure success. If you’re going to have multiple teams building and implementing AI models, look for ways to connect those teams. One way to do this is to create an AI council or steering group that includes key stakeholders not just in your own division, but across your organization, so everyone can make their needs known before a training data vendor is chosen.
Plus, because the AI field is evolving so rapidly, AI councils and steering groups can help you make sure your partner strategy remains aligned with your needs as they change.
Terminology and communication
Clear and consistent communication is key to the success of any AI data services partnership. By establishing the terminology your team will use when discussing AI and your data needs, you can ensure your communication is as clear as possible from day one.
As you evaluate potential partners, clear communication and consistent terminology will also enable you to compare apples to apples when evaluating potential AI data partners. If you’re on the same page with communication and terminology, that’s a good sign for your wider partnership moving forward.
What are your most pressing training data needs?
Training makes up around 80% of the time required to build an operational AI model. If your teams are eager to get moving as quickly as possible, work to determine exactly what they need to do that. With their current needs defined, you can start looking for a partner that’s able to meet those needs at the scale and speed they need.
What will your data needs look like in 3 months, 6 months, 1 year or 3 years?
The journey to AI success is rarely linear. It’s very likely that your data needs will evolve over time. So, for each AI stakeholder group within your organization, look ahead and consider how their needs are most likely to evolve in the short and long term. Then, select a vendor that’s capable of meeting both your current and projected future needs.
After selection: Working with a single vendor
Once you’ve found a suitable vendor that you believe you can build a successful long-term AI training data partnership with, it’s time to establish some ground rules to help make your partnership as streamlined and effective as possible.
Here are some important considerations to make across the different stages of you and your partner’s new connected workflows.
Stage one: Onboarding
To get your working relationship off to the right start:
- Share preferred terminology with your vendor so you can both speak the same language and communicate clearly.
- Find out who you’ll be working with on your vendor’s side, and if you don’t feel their skills and expertise are well-aligned with your AI needs, suggest changes to ensure you’ve got the right people on your projects.
- Take a close look at your vendor’s pricing model – understand what levers affect overall cost and how you can influence them.
- Explore the potential for volume-based discounts and make them practically attainable for your teams.
- Establish regular update meetings and clear lines of communication between all relevant stakeholders and groups.
Stage two: In-process
Once you start working with your vendor it’s important to have effective checks and balances in place to ensure that all stakeholders get what they need from your partnership. By applying the following practices, you can ensure your workflows remain strong following a successful start:
Pilots and testing: Giving your vendor the time they need to perform pilot projects and testing enables them to validate any assumptions made throughout your vendor selection process. Not only will this help to ensure both parties’ expectations are aligned, but will also potentially allow for simplified and more predictable pricing. During the pilot phase, don’t focus on items such as driving average handling times down or decreasing the number of resources to cut costs – instead focus on getting an accurate depiction of all the steps and effort involved.
Evaluation and measurement: Don’t rely on your vendor to tell you how they’re doing. Build your own scale and metrics for performance measurement. Create a consistent approach that’s aligned with your stakeholders’ needs, and remember to build benchmarks first, so you have a clear view of what good performance looks like.
Escalation: Mistakes happen. Your vendor will make missteps as a result of miscommunication, improper planning, administrative errors, or simply bad luck – they will fall down. So, it’s a good idea to have proper escalation plans in place for when your vendor gets something wrong. This should include who will be involved in the resolution process, how that process will work, how any extra costs incurred because of the issue will be handled and what the people and teams involved should do to prevent the issue from happening again.
Create your escalation plan but be open to changing it. If you determine that a vendor has to do a full root cause analysis (RCA) for every minor mistake, they will end up spending more time talking about how problems will be resolved instead of resolving them. Regardless of how a mistake happened, take time to understand what could have been done differently to prevent it from happening. Don’t feel the need to overcome vendor deficiencies, but be open to the idea that what may seem clear and well-defined to you may not be for your vendor.
Considerations for a multi-vendor AI data services partner strategy
If you’re planning a diverse range of AI use cases, or your needs demand a particularly high volume of reliable training data, you may find that you have to work with multiple AI data services vendors. This approach allows for greater scalability while keeping checks and balances within your training data process. However, having more parties involved can also introduce consistency and management challenges.
To keep your multi-vendor partner strategy on track, pay special attention to the following:
Consistency
When you’ve got multiple vendors to manage, consistent terminology and communications become even more important. If your internal stakeholders have to use different terms when communicating with different vendors, that’s going to create issues and misunderstandings fast.
The other factor to consider here is pricing. Pricing needs to be consistent between vendors so that everyone can budget with confidence, and predict their future costs. To do that, you’ll need to create a consistent pricing model that all of the vendors you work with must adapt to.
Volumes
The volume of data and support you need from each vendor may impact the level of service they provide to you. A single-vendor partnership fosters deep loyalty between you and your chosen partner, but in multi-vendor partnerships, that’s more difficult to achieve.
Be upfront with all your vendors about the realistic volume of work you’ll need from them. Then, use their response to those projections to determine how strong of a fit they are for your multi-vendor strategy.
Volume discounts
Similarly, different vendors may offer different volume discounts. Take the time to look at when those discounts are activated and calculate the best way to allocate work in order to hit as many discount thresholds as possible.
In some cases, working with fewer vendors and achieving higher volume discounts is a better option than working with a wide range of vendors. There may even be cases where some vendors already provide different services to your business – making combined volume discounts across service sets even more appealing for your organization.
Communications and management
In an ideal world, your vendors should be able to adapt to your processes and communicate with your stakeholders in a consistent manner. However, things don’t always work out that way.
Carefully consider how work is distributed across your chosen partners to avoid situations where daily communication from multiple vendors and vendor contacts overwhelm your stakeholders
Technology tooling
Each one of your vendors will likely have their own tooling that they prefer to use to deliver their services. However, if you engage with one vendor’s tool but not any other, that can decrease the value you see from working with the other vendors.
Try and find a tool that all vendors are happy working with. If the tool selected is a requirement, consider this when selecting vendors. Some tools are first-come-first-serve in that they deliver a stream of available tasks that vendors can then claim. If your tools offer active vendors the chance to win more work, there’s a high chance they’ll happily engage with them.
Evaluation and measurement
Build your own scale for measuring performance that’s consistent across all vendors. That will enable you to objectively evaluate them against one another and continuously optimize how your vendor portfolio is balanced.
Understand what you need and the right partners will present themselves
Whether you opt for a single or multi-vendor AI data services partner strategy, success is simply a matter of finding the right partner(s) to meet your needs. The more clearly you can define those needs upfront – from your core data requirements, through to communication preferences – the better positioned you’ll be to build the right supplier portfolio. Then, all you need to do is stay on top of changes and make sure you and your suppliers remain aligned in the areas that matter.
Evaluating AI data vendors to train or fine-tune your generative AI? Download our checklist to evaluate AI data services providers and get your project off to the right start.