Strategic spending: Optimizing your AI data budget for generative AI success
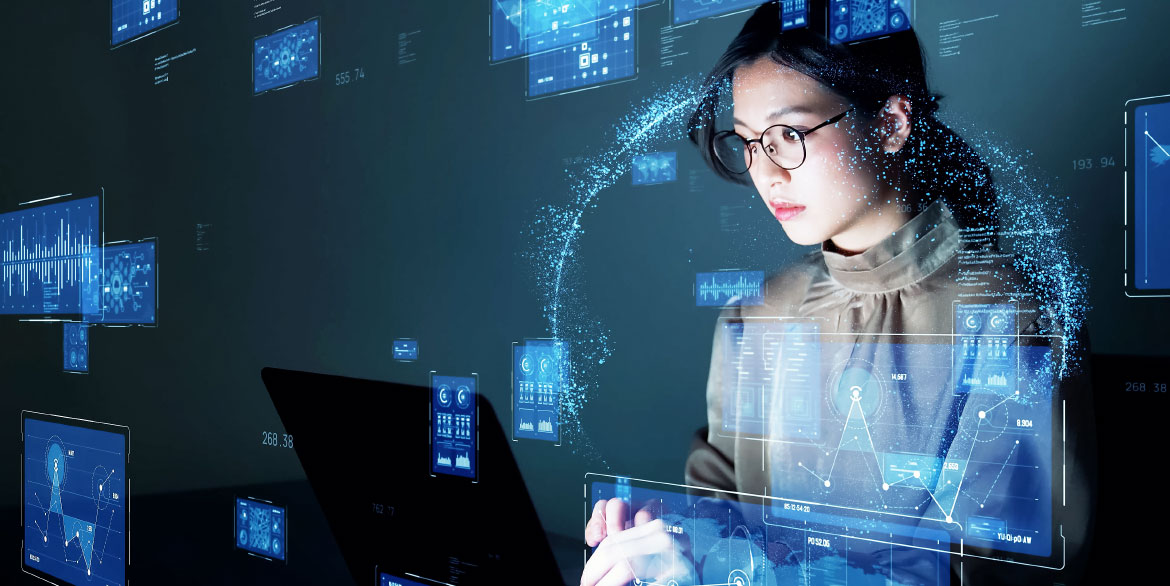
When shopping for AI data services, you might ask yourself the following questions: How much does the data, content or feedback required to train or fine-tune generative AI cost? What issues can cause costs to increase? And how can those issues be overcome?
It can be difficult to understand the costs associated with training and fine-tuning generative AI. Pricing models often vary wildly with some fees baked in, and others completely hidden. Furthermore, required spends and volumes are often completely unknown upfront, making it difficult to get the best price possible amidst the uncertainty.
Generative AI training and fine-tuning demand different resources with greater specialization. The power it brings is immense, as are the risks. Here are four key factors to consider when building your generative AI training budget.
Resource compensation
- Where do resources need to be located?
- What language(s) should they speak?
- Do they need to specialize or have expertise in a particular domain?
- How many total resources will be needed?