Scoping your AI training data project
06 May 2024
5 minute read

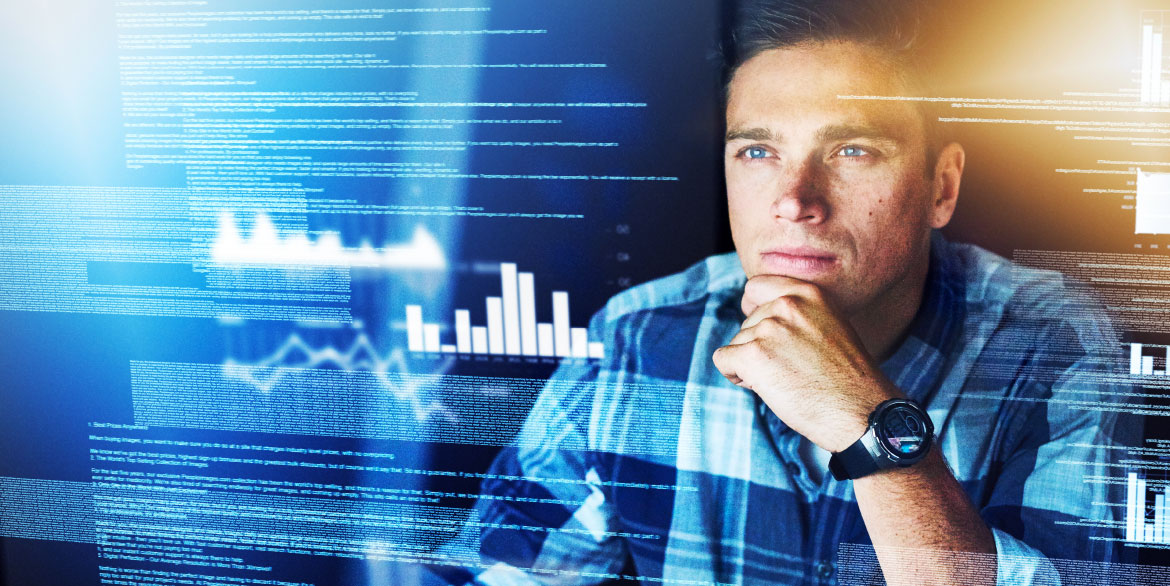
When you're planning to outsource the annotation of your data for machine learning (ML) training purposes, it’s crucial to scope your AI training data project carefully. Without a clear definition and documentation of your needs, you may end up doing more work than expected, or worse, with inaccurate data to train your AI model.
In this article, I'll walk you through the process of scoping your AI training data project, from understanding your data requirements to preparing your project scope document.
Step one: Define your AI data requirements
Gather project information
Before starting work on your data project, it’s important to understand exactly what you need, and what you want to achieve. That means gathering all the necessary information from relevant internal stakeholders, including ML engineers, data scientists and business owners. They'll be able to tell you about the model they're trying to build, their AI project objectives, the specific data they need to train it and the level of data accuracy they expect. Once you have this information, you can begin to map out your AI training data project.
Consult with your AI data services provider
If possible, this is when you should start engaging with your AI data services provider, since a reputable provider will be able to provide recommendations to help shape your project based on their expertise in terms of data collection, annotation and validation best practices, and their experience on similar projects. Don’t be afraid to set up a direct communications channel between your internal stakeholders and your AI data services provider – it can lead to fruitful discussions and improvements implemented even before the project kicks off.
Break complex data projects down into steps
Sometimes you won’t be able to define the full scope of your project from day one because of other team dependencies, or overly ambitious timelines. In such cases, you should work with internal stakeholders to break the project down into smaller, independent, more manageable steps. That way, you’ll be able to focus on one step at a time without impacting other steps. Regular check-ins and open communication are key to keep the progress of the project on track and avoid potential scope creep.
Step two: Set your quality expectations
Define quality KPIs
The crux of all data collection and annotation projects is the quality of the training data delivered, which is critical for your models to learn effectively and produce accurate results. The tricky part of managing data quality is that the concept of quality can mean different things to different people.
Therefore, you should always strive to quantify your quality expectations in the form of measurable targets or key performance indicators (KPIs).
Furthermore, if there are different dimensions to your quality expectations such as closeness to ground truth, variance or diversity, create separate KPIs for each quality dimension to keep tracking and monitoring transparent.
Share your AI data quality audit plan
Be transparent about any quality audits you plan to conduct on your end. Contrary to what many think, data quality auditing transparency doesn’t allow your AI data services providers to relax their requirements and let quality slip. Rather, it sets the right expectations on both sides from the start.
Step three: Incorporate responsible AI
Don’t forget to take AI ethics and the principles of responsible AI into consideration when scoping your AI data project, acquiring training data and training your ML model. This means ensuring that your data is legally sourced and data workers are treated and compensated fairly and ethically. It also means ensuring data diversity, inclusivity and transparency to minimize the potential for bias in your AI training data and model.
This is yet another area where great AI data services providers really shine. They’ll be able to advise on responsible AI pitfalls and propose mitigating actions to reduce bias in your AI training data and discriminatory outputs from your AI model.
Step four: Establish your project budget
Budgeting is a key aspect of planning your AI training data project. It's essential to balance the financial resources available with the quality and quantity of data you require.
It’s important that data science and R&D teams work with AI data services providers to understand every factor that can influence project cost. Key factors to consider include the volume of training data required, the number, demographics and skillset of workers needed to prepare the data, the estimated time it will take to perform the data collection, annotation, validation or fine-tuning tasks needed and the resources required to complete quality assurance checks. Remember that the higher the quality of data needed, the more expensive the process is likely to be due to additional investments in QA time. You should also add a contingency for any unforeseen changes that may arise.
Once you've estimated these costs, you can match them against your available funds to establish a realistic budget for your project. This is another area where your AI data services provider can provide invaluable insights – be sure to leverage their knowledge and expertise to set a realistic budget for your AI training data project.
The final step: Document your project scope
Now that you've gathered and considered all of the essential information, the final step is to compile what you’ve learned into a structured project scope document. The scope document should describe the overall project objectives and desired outcomes for your AI model, the different types of data needed and the roles and responsibilities of all parties involved. This should be followed by a detailed breakdown of tasks and deliverables, including expected timelines and critical milestones.
The scope document should also clearly outline your quality expectations, including defined KPIs and the plan for any data quality audits. Finally, include your project budget to provide a clear picture of the project's financial requirements and constraints.
Remember, a well-documented project scope serves as a roadmap, guiding all stakeholders through the project's journey, helping to set project expectations and manage risks. It's crucial to ensuring the successful delivery of your AI training data project.
Planning a generative AI project? Download our generative AI decision roadmap to understand key decisions you should make upfront to ensure project success.