Driving powerful search with knowledge graphs

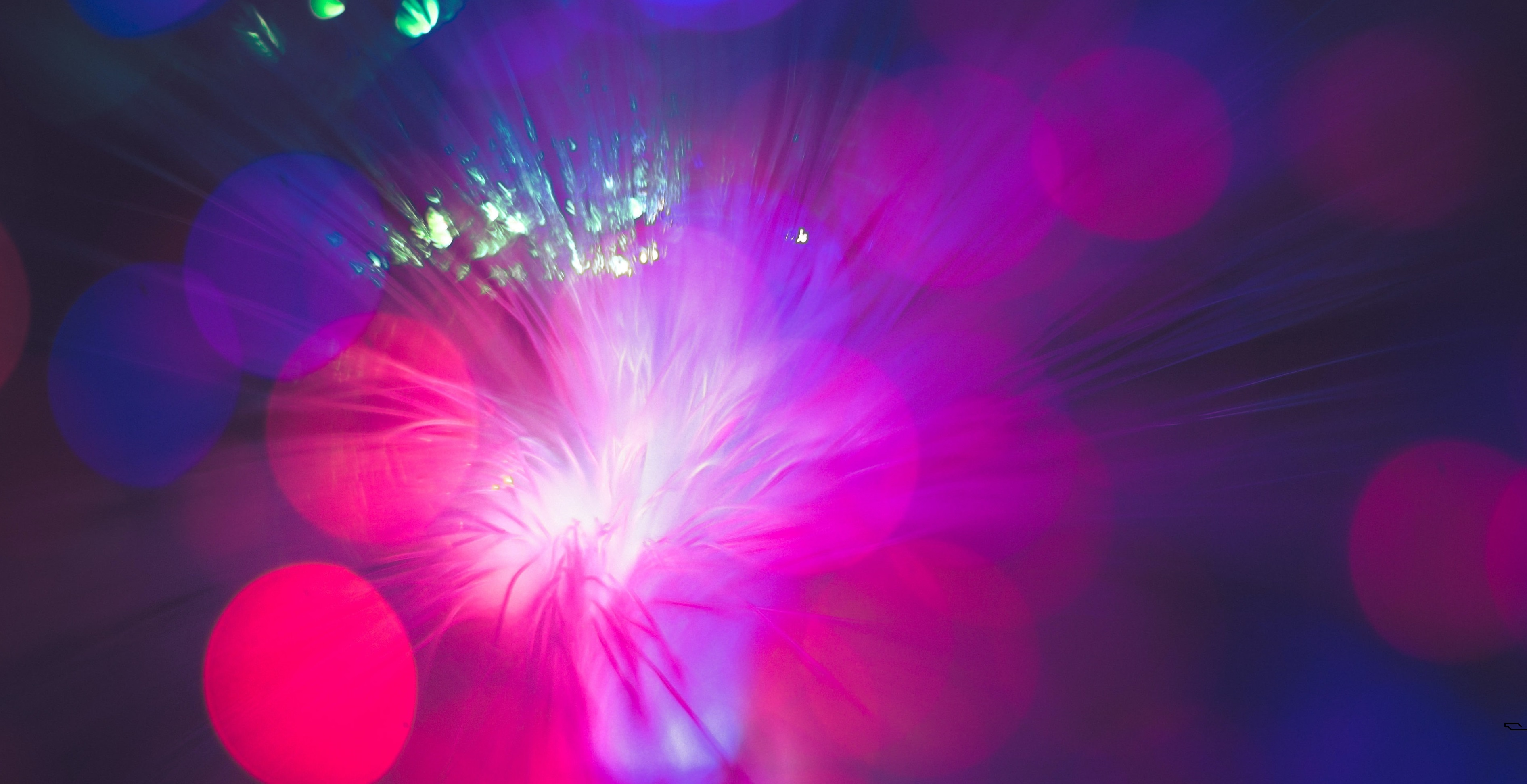
In today’s fast-paced digital world, users expect relevant information at their fingertips. Those who provide the most relevant information in as less time as possible excel, while others continue to languish and struggle to stay afloat.
Today’s search is all about helping people get things done in a few seconds. And it is not just about providing the relevant information but also working as an expert to guide the user to various options or possibilities given their interests or search objective/s.
Further, search is not just limited to text but extends to more advanced formats such as voice, AR/VR, or chatbots. We will discuss the various stages in which search has evolved over time to become much more intelligent and effective in serving users’ objectives.
Evolution of search
As we know, it all started with the basic keyword search that provided us access to large amount of results, some relevant and some irrelevant. To refine the results, the search matured into a “full-text search”, which worked to an extent but not necessarily served the user objective.
The search then evolved to faceted search, allowing users to apply several filters to weed out irrelevant or least relevant results. This worked well, however, it lacked context because it didn’t track the user behavior.
To overcome this action-oriented search was introduced, in which the user clicks were traced to further refine the results. This still had limitations because the starting point is always the exact text entered by the user. The search technology didn’t account for the context or objective behind the search.
This limitation gave birth to the concept of semantic AI, which aims to understand the context in which the user is carrying out the search. In the simplest form, two different users are searching for flight details. One is performing the search to book a flight, while the other is to pick someone up from the airport or to coordinate or plan onward transport at the destination.
A simple text-based search won’t be able to distinguish between these two users and help them meet their objectives. But semantic AI can because it draws linkages between various information assets and users and vice versa to define all potential relationships between them.
Search maturity roadmap:
Let’s understand at a more granular level the way in which search has evolved at each stage and the way in which its applications have matured over time:
1. Basic keyword search
A keyword search as the name suggests looks for words anywhere in the record. Keywords may also substitute for a title or author search when you have an incomplete title or author information.
In the case of keyword searches and metadata searches, an organization must assign specific information to each artifact that will be used to locate information for a particular use. Many of today's vendors have been creating scanners and other programs that will scan through a document and simultaneously assign keywords or try to classify artifacts according to a user-defined metadata structure (metamodel).
2. Full-text search
Full-text searches scan documents from top to down, finding the words or parts of words that match the user's selection. This type of search returns and recognizes textual artifacts comprising the selected terms and ranks them by the level of appropriateness according to the word frequency or how the words are combined in the relevant context.
3. Faceted search
Also known as faceted navigation or faceted browsing, it is a technique used by eCommerce organizations to help their users analyze, organize, and filter large sets of product offerings based on filters such as size, color, price, and brand. Faceted search based on taxonomies enables users to filter irrelevant search results to improve findability quickly.
Ideal applications for such search are the instances that vary by breadth vs depth of information. And hence, e-commerce is one of the best use cases for faceted search. However, this has limitations, as the search results don’t change based on user behavior.
4. Action-oriented Search
The focus here is on the user – what does the user want to do or what do they want to find? In other words, how can search results be used for measuring the success of an intended action?
Action-oriented search shouldn't be just a link to a vast data dump in a document. If the user must find his way through the information he needs, an action-oriented search isn't successful.
5. Knowledge Graph-based search
A knowledge graph connects various information assets to people and people to other objects and defines the relationship between them. Over the period, based on the search experience, the search results improve. Also, a knowledge graph improves search by capturing the meaning of the search terms. That's why Knowledge graph-based search is also called ‘semantic search’. They rely upon defined relationships between various objects to narrow down possible search results. These objects can be varied types of data sources.
A knowledge graph combined with the power of metadata and taxonomies powers semantic search, and the search results improve over time based upon the user feedback – which can be collected in various forms such as a thumbs-up/down, question at the end of the information and rating.
Building intelligent search
In a nutshell, search has evolved from mere keywords to having a conversation. We expect search to become an information exchange and instead of the traditional one-way interaction. The goal is to make information more easily accessible and relevant to serve the user’s objective.
3 Key considerations for a successful search:
1. Search is about ‘things’, not ‘strings’
What action are you enabling based on the user’s search or activity? When searching for an expert: the search is carried out to identify relevant experts, understand their level of expertise, find where they are located, and whom they work with or are associated with. Hence, an ideal search for finding an expert should not only return a few links but all the relevant information about the experts to complete a user's journey or objective.
2. Knowledge graph: a map of information across an organization and how it relates to each other
Knowledge graphs connect information across the organization and draws relations. As a result, it delivers several benefits such as:
- Understands context: How things relate to each other?
- Enables natural language search: Stores information on how people think, making search natural and easier
- Structured and unstructured information: integrates various forms of content to enable simultaneous multiple-format search
- Aggregates information from multiple repositories in various formats
3. AI and ML can be either rule-based or statistical
A good search shouldn't be either or it can be both since both have their respective right use cases and time.
How Tridion helps you build powerful search experiences?
If you want your organization to offer customers a better search experience – more like Google or Amazon— you need to build in semantics. This is equally applicable if you focus on helping your employees find what they need.
Tridion has built-in semantic AI. Semantic AI is a SaaS-based offering available for the various components of Tridion: Tridion Sites for Web Content Management, Tridion Docs for Structured Content Management, and Dynamic Experience Delivery for search and headless content publishing. It can be used with both: on-premises and cloud-based deployments of Tridion.
A new module of Tridion, ‘Taxonomy Space’, allows companies to manage their underlying knowledge models based on open standards such as SKOS and EuroVoc.
There are multiple ways in which semantic AI will benefit organizations, and it can be used across several different use cases, such as:
- Self-service experience optimization
- Enterprise search-enabled workplaces
- Digital experience enhancements
- Recommender systems
- Conversational interfaces
If you would like to learn more about how Tridion can help your business, click here.