Issue #10 - Evaluating Neural MT post-editing

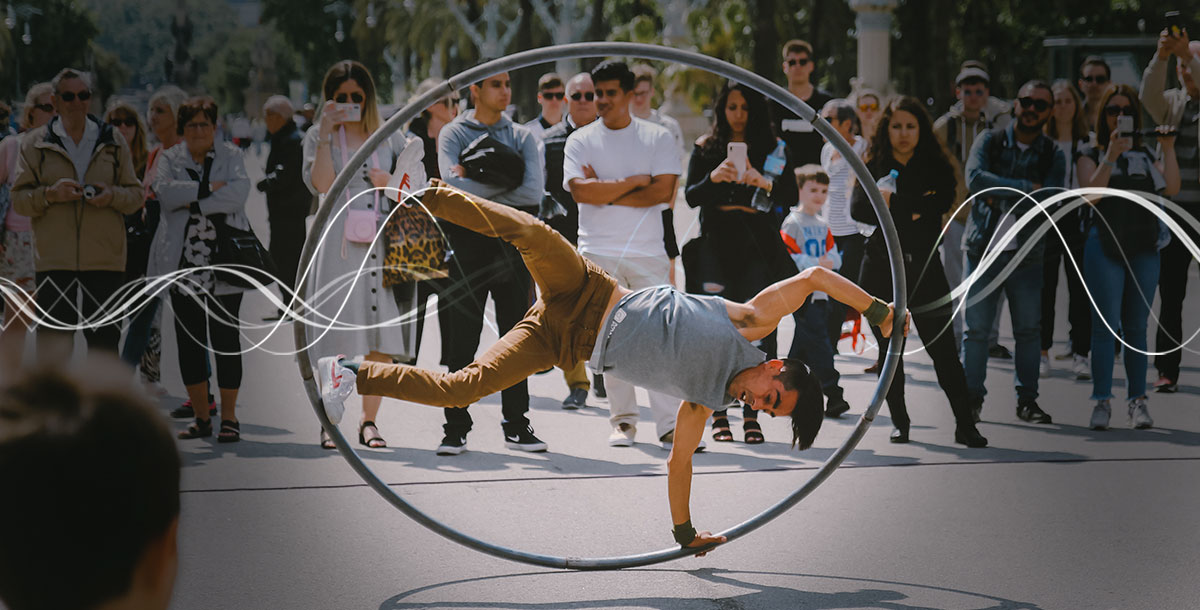
Dissemination vs. Assimilation
The previous articles in the Neural MT Weekly have looked at various aspects of NMT training, data preparation, and evaluation. Once you’ve produced the NMT output, what happens next? MT for assimilation means that raw MT is the end product, giving a gist translation; MT for dissemination means that MT output is “an intermediate step in the production” of the final text, usually followed by post-editing (there’s a good definition of this in Forcada 2010), and it’s that latter use of MT that’s the topic of this article.Is NMT post-editing faster than SMT post-editing?
When we carried out a comparative evaluation of statistical and neural MT as part of the TraMOOC EU project (published as Castilho et al. 2018), one of our measures of quality was post-editing effort, measured as temporal effort (i.e. productivity, or the amount of time spent post-editing) and technical effort (the actual number of edits, often measured in keystrokes/segment). Language pairs were English to German, Greek, Portuguese, and Russian, and the systems were trained on educational data as detailed in the paper. Where we might have expected NMT post-editing to be far faster than SMT post-editing (as NMT showed improved word order, fewer morphological errors, and rated highly for fluency), measurements of temporal post-editing effort did not reflect our expectations. Production speed for NMT post-editing was roughly the same as for SMT, despite the fact that far fewer segments required editing, and the number of errors found in our annotation exercise was also fewer for the NMT output.
Technical post-editing effort was lower for NMT, however, with fewer keystrokes required for NMT segments in all language pairs. Where other researchers had found a deterioration in NMT quality for longer sentences, we did not. When our experiment participants (all professional translators) were asked about this, they said that the errors were fewer in NMT output, but they were more difficult to spot. SMT errors were familiar and usually quite obvious, but the fluency of NMT was deceptive.
In order to investigate further how translators/post-editors interact with NMT, a group of us decided to co-edit a special issue of Machine Translation journal. The articles submitted are currently under review, but we can see the same pattern reappearing in different language pairs (EN-ES, ES-DE, EN-NL…) and in different domains. NMT systems are rated highly for adequacy and fluency (at segment and document level), automatic metrics show positive results for NMT, and technical effort is less for NMT post-editing. Nonetheless, if there are improvements in post-editing productivity for NMT output (and results here are mixed), they are minor, and not statistically significant. Most show a productivity disimprovement in longer sentences.
Of course, post-editing isn’t the only option for post-production of MT output. Some tools incorporate interactive or adaptive MT, whereby the whole MT suggestion updates in real time as the user makes changes to words in the text. One of the studies in press tests an adaptive NMT tool, finds that editing time is not improved when compared with adaptive SMT, with the caveat that the number of participants is small. An important addition here is that users tend to prefer working with the NMT output. The translation industry in general does not yet appear to put great store in usability for translators or post-editors as it’s difficult to make a strong link to cost, despite the suggestion from scholars such as Abdallah (2017) that quality should be measured in three dimensions: product, process, and social.
Finally, studies such as Skadina and Pinnis (2017) show that using NMT can lead to slower post-editing speeds for minority languages, especially in narrow domains, due to the small amount of training data available. They find similar productivity rates for translation from scratch and SMT post-editing in English to Latvian, with poorer results for NMT post-editing. The automatic evaluations published by Dowling et al. (2018) suggest that post-editing throughput for English-Irish translation is likely to be similar.