Issue #119 - Machine Translationese: Effects of Algorithmic Bias on Linguistic Complexity in MT

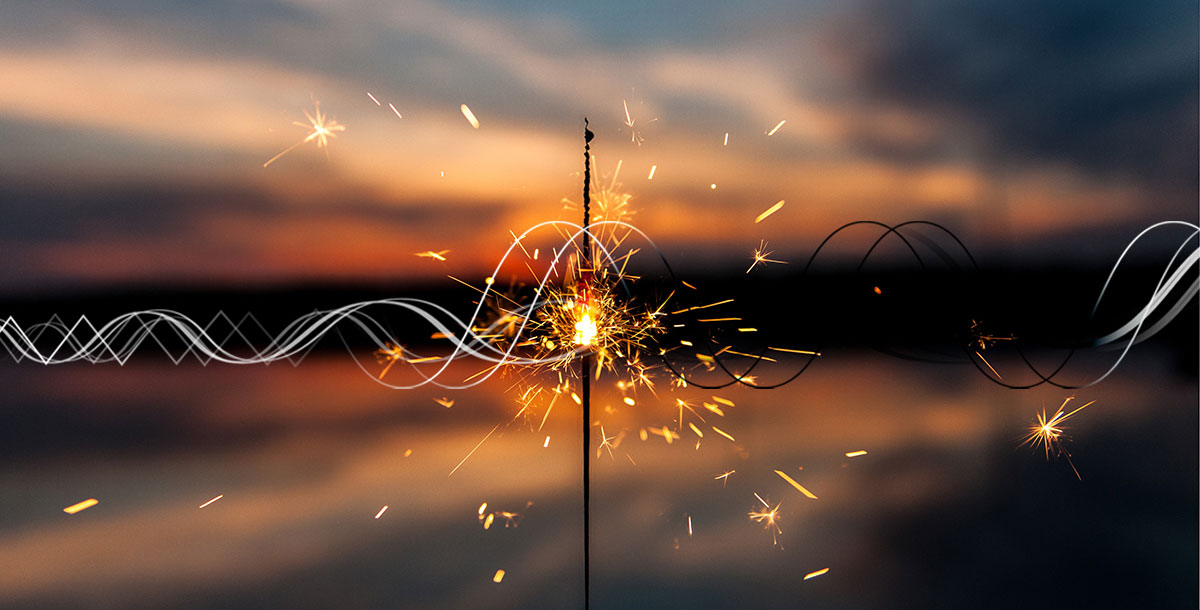
This week we have a guest post from Eva Vanmassenhove, Assistant Professor at Tilburg University, Dimitar Shterionov, Assistant Professor at Tilburg University, and Matt Gwilliam, from the University of Maryland.
In Translation Studies, it is common to refer to a term called "translationese" that encapsulates a set of linguistic features commonly present in human translations as opposed to originally written texts. Researchers in the Machine Translation field have explored how this could be transposed to post-editing tasks and hence talk about "post-editese" (if you are curious, this paper by Joke Daems et al. may be a good starting point). Eva and her colleagues go one step further and investigate "Machine Translationese". In the paper they summarize for the Iconic Neural MT weekly, Eva and her colleagues explore the difference between the richness of the data used to train Machine Translation (MT) systems and the output they produce. Could this be attributed to some sort of statistical/algorithmic bias? Read below to learn what they found out!
Introduction
Recent studies in the field of Machine Translation (MT) and Natural Language Processing (NLP) have shown that existing models amplify biases observed in the training data. These biases have been primarily examined with respect to specific phenomena (e.g. gender bias (Vanmassenhove et al., 2018)). In the paper “Machine Translationese: Effects of Algorithmic Bias on Linguistic Complexity in Machine Translation” we examine the effects of statistical or algorithmic bias on language in a broader sense. We hypothesize that the exacerbation of frequent patterns observed during training in combination with a loss of the less frequent ones leads to an artificially impoverished language: ‘machine translationese’. In order to investigate this hypothesis, we assessed the linguistic richness of 12 different flavors of machine translationese on a lexical and morphological level: two language pairs, English (EN) <=> French (FR) and English (EN) <=> Spanish (ES), and three types of MT systems, PB-SMT, Transformer NMT and RNN NMT.Translation as Transformation
The idea of (human) translation entailing a transformation is widely accepted in research in Translation Studies (Ippolito, 2014). It has been empirically established that the translation process leaves fingerprints resulting in a language with specific characteristics, commonly referred to as ‘translationese’ (Gellerstam, 1986).
So far, in the field of MT, the main objective has been the generation of accurate and fluent translation. Maintaining the richness and diversity has understandably not been a priority (Vanmassenhove, 2020). However, MT systems are currently used widely on a daily basis and have reached a quality that is (arguably) close to that of human translations (Laubli et al., 2018; Toral et al., 2018). As such, it is time to look into the potential effects of MT algorithms on language itself.
‘Human Translationese’ vs ‘Machine Translationese’
While human translationese is characterised by features such as ‘explicitation’, ‘normalization’, ‘leveling out’ and ‘simplification’ and can be partially due to intentional choices made by the translator, machine translationese is a by-product of our algorithms and their application on specific training data. Statistically biased MT (preferring frequently occurring (sub)words over less frequent ones) might not only lead to a decrease in lexical richness (e.g. loss of synonyms) but could also interfere with the correct generation of appropriate morphological variants. Furthermore, given that language contact (e.g. via translationese) can entail language changes (Kranich, 2014) a sociolinguistic perspective comes into play. If machine translationese (and other types of ‘NLPese’) are indeed a simplified version of their training data, what would that imply from a sociolinguistic perspective and could this affect language in the longer term?Assessing the richness of Machine Translationese
Given that this study is the first attempt to quantify both the lexical and the morphological richness of machine translationese, experiments were conducted with the three state-of-the-art data-driven MT paradigms: Neural MT (RNN and Transformer) and Phrase-based SMT. We trained English-French and English-Spanish MT systems (in both directions) on the commonly used Europarl (Koehn, 2005) corpus. The trained MT systems were then used to translate the source side of the training data, i.e. completely observed data. Data that has been fully observed during training is the most suitable to research the effects of the algorithm on language itself. Translating observed data is also the most favourable translation (and evaluation) scenario for the MT systems.
Assessing the richness of language is a difficult, multi-aspectual task. To quantitatively measure the difference between the linguistic richness of an MT system’s training data and its output, an in-depth analysis of the lexical richness and morphological richness was conducted. The lexical richness is assessed using: (a) three standard metrics (TTR, Yule’s I and MTLD), (b) an adaption of metrics used to assess lexical sophistication of language learners (Lexical Frequency Profile and Beyond 2000), and (c) three new metrics that look at synonyms/translation alternatives. For morphological richness, metrics originating from Information Theory that are currently mainly used to assess the evenness and richness aspect of diversity in biology, economy and ecology (Shannon Entropy and Simpson Diversity) were exploited. With the Shannon Entropy and Simpson Diversity, we measured the entropy and diversity of inflectional paradigms per lemma by looking at the distribution of word form counts per lemma.
Less is not always more
All 9 metrics employed indicate that the MT output of all systems, in both language directions, is less lexically and morphologically diverse than the training data it was originally trained on – a product of algorithmic bias. The findings with respect to lexical diversity are in line with the work described in Vanmassenhove et al. (2019). As an illustration of the consequences of morphological diversity, Table 1 shows the word counts for specific word forms of the French lemma président (EN: president) over the original data and the different MT systems. It can be observed that the word présidentes (female plural form of the word président), an already rare word form in the training data (8 occurrences), has completely disappeared from the output of the LSTM and Transformer model (n/a).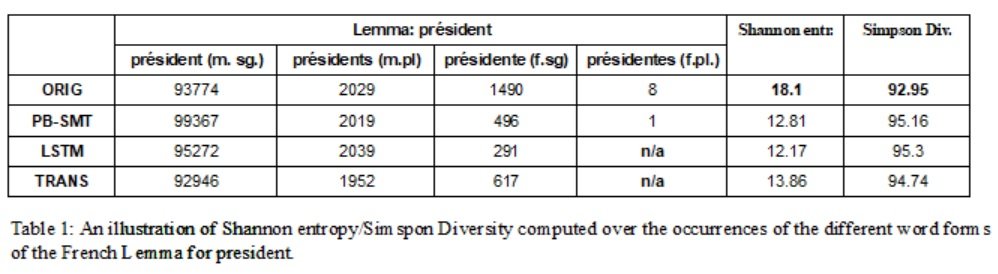