Issue #2 - Data Cleaning for Neural MT
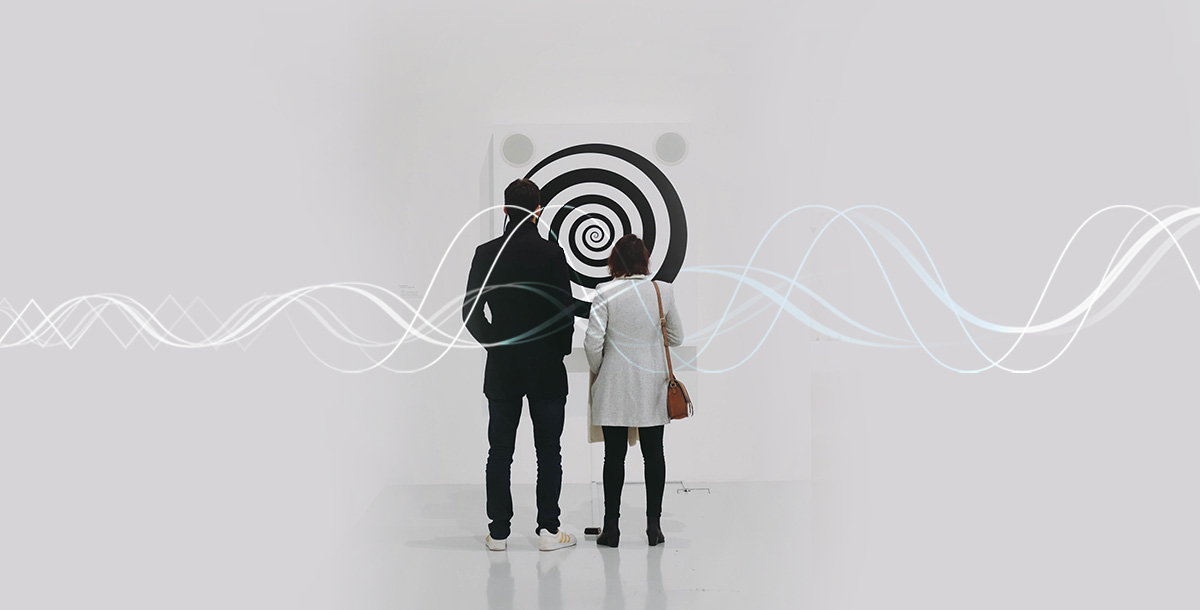
Introduction
“Garbage in, Garbage out” - noisy data is a big problem for all machine learning tasks, and MT is no different. By noisy data, we mean bad alignments, poor translations, misspellings, and other inconsistencies in the data used to train the systems. Statistical MT systems are more robust, and can cope with up to 10% noise in the training data without significant impact on translation quality. Thus in many cases more data is better, even if a bit noisy. According to a recent paper (Khayrallah and Koehn. 2018) the same can not be said for Neural MT, which is much more sensitive to noise.
Where is the problem?
Let’s look at their comparison of the impact on the BLEU score of several types of noise in the training data for German into English machine translation.
The most harmful type of noise are segments of the source language copied untranslated into the target, e.g. German aligned with German. With only 5% of this type of noise, the BLEU score drops from 27 points to less than 18, and with 10% of such noise, it drops even further down to 11 points. This issue had also been observed by Ott et al. (2018) in their paper on “Analyzing Uncertainty in Neural Machine Translation”. Other types of noise which are particularly harmful for Neural MT are misaligned sentences (training sentence pairs in which source and target segments do not match), wrong language in the target side of the training sentence pairs (French when it should be English, it’s surprisingly common!), very short segments (2 words), and misordered words. The impact on the Neural MT BLEU score of these types of error is between 0.5 and 0.7 up to 10% of noise, and up to 1 point for 50% of noise.
How can we fix it?
As a consequence, the field of parallel data filtering has regained activity recently. So much so that it will be the subject of its own shared task in the Third Conference on Machine Translation (WMT 2018) and there are several research groups and companies dedicating significant resources to it. For example, the eBay labs recently gave a tutorial on the importance of parallel data filtering and their best practices. They described two methods to filter out misaligned sentences. A simple and quick method based on the score of simple word alignment models, and a more accurate method based on translating the source side of the corpus and comparing this translation to the target side.
As for the other types of noise, filtering very short segment and source segments appearing untranslated in target is relatively straightforward as we can simply match strings of a certain length. Sentences in the wrong language can be detected by a combination of two approaches: language recognition based on machine learning, and detection of the character set, e.g. if we’re expecting English, there shouldn’t be many/any accented characters, Cyrillic, etc. Misordered words can be detected with machine learning approaches looking at features such as n-grams or language model scores. Recently, some approaches using neural networks themselves were proposed. For example, Tezcan et al. (2017) model n-grams with morpho-syntactic representations of words to detect several types of grammatical errors.