Issue #27 - Use case: Neural MT for the Life Sciences
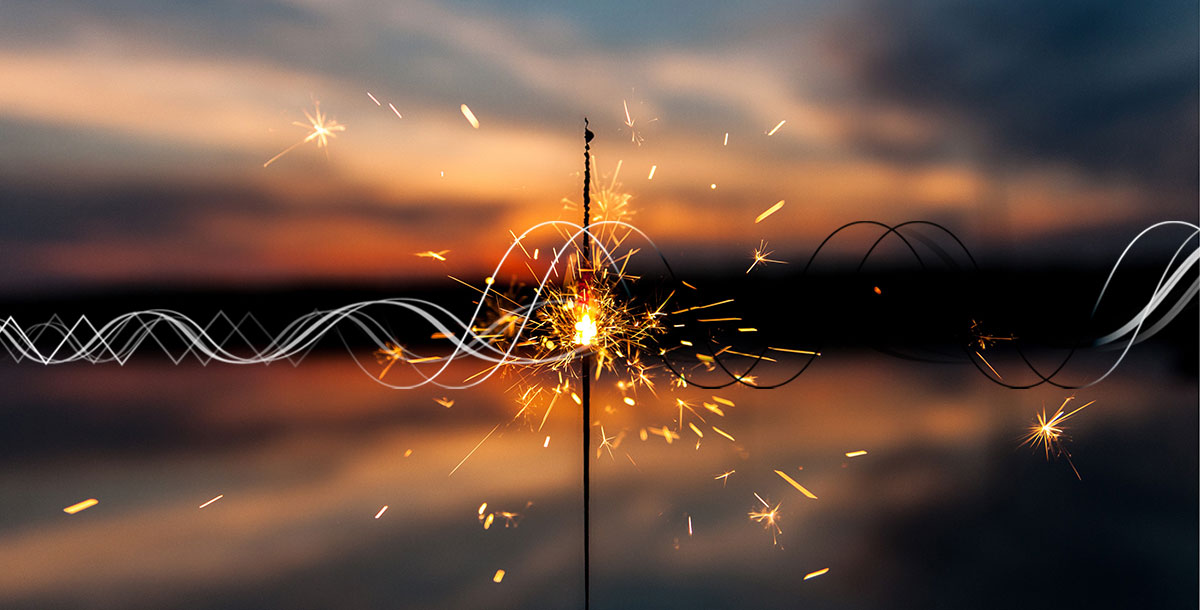
Introduction
Neural MT has had quite a significant impact on how global enterprises are looking at translation automation to improve existing workflows. Above and beyond that, however, organisations are considering how machine translation can transform key areas of their business.
The reasons for this are clear - when adapted effectively for a particular use case, Neural MT can produce significantly better, more reliable results. Many enterprises dipped their toes into the world of MT in the past, found it inadequate, and the concept was abandoned. Now, it’s being revisited and adopted at scale, and the results are just that - transformative.
A prime example of this is the life sciences. Pressure to go global faster, get drugs to market around the world, run clinical trials in multiple countries, and assess adverse event reports, means that Neural MT is increasingly not just the best option, it’s the only option. In this article, we take a look at one particular use case in the field of life sciences where Neural MT is gaining a lot of traction: pharmacovigilance.
Use case: Pharmacovigilance
Pharmacovigilance, also known as drug safety, is the process by which pharmaceutical and related companies collect, detect, assess, monitor, and act on adverse events with their pharmaceutical products (source: wikipedia). These adverse events, or side effects, can be reported by any users of the products - medical practitioners, or individual consumers - across a variety of channels.
Internal procedures, as well as various regulatory requirements mean that organisations need to act on these reports often in a very short timeframe, and that presents a challenge.
What is the challenge?
Volume, language, format - you name it, it’s a challenge!
Year on year, the number of cases being reported is rising, particularly as more products are launched in the market. We’re talking in the order of millions. With the growth in numbers of adverse event reports, comes the growth in the proportion of multilingual content that needs to be interpreted and acted upon. In 2018, more that 60% of such reports were non-English.
Existing processes for case intake are heavily manual and effort intensive, and are unsustainable in the long term. They rely on external support at multiple stages, particularly translation, and the quality of the service varies greatly from country to country.
We’ve reached a tipping point, from which the only practicable solution is centralising the entire workflow and introducing automation wherever possible, and that’s where Neural MT plays a role.
How is Neural MT helping?
Before MT can be effective, engines need to be heavily tailored to the specifics of the domain, and a bespoke solution in place for each client. Pharmaceutical companies have particular ways their products are referred to across markets. On top of that, different health organisations have introduced standards on how to refer to specific adverse events.
At Iconic, we’ve been working with a number of pharmaceutical companies and CROs (Contract Research Organisations) over the past 18 months to develop and assess such Neural MT solutions. The image below illustrates some of our very positive findings in terms of the quality of bespoke Neural MT.
As a company, this was something we tried 4-5 years ago with little success. As we suggested when we discussed another case study in Issue #14, Neural MT is opening doors to new applications that simply weren’t viable before. [caption id="attachment_8033" align="aligncenter" width="618"] Usability of Neural MT output across various cases[/caption]
In summary
Neural MT is being rolled out rapidly across the life sciences. When it comes to pharmacovigilance, it is allowing organisations to centralise the case intake process, which reduces the backlog of adverse event reports, and ultimately allows them to provide better care for patients.
In the coming months, we’ll be publishing a number of detailed case studies on further applications of Neural MT in the life sciences, so stay tuned!