Issue # 33 - Neural MT for Spoken Language Translation

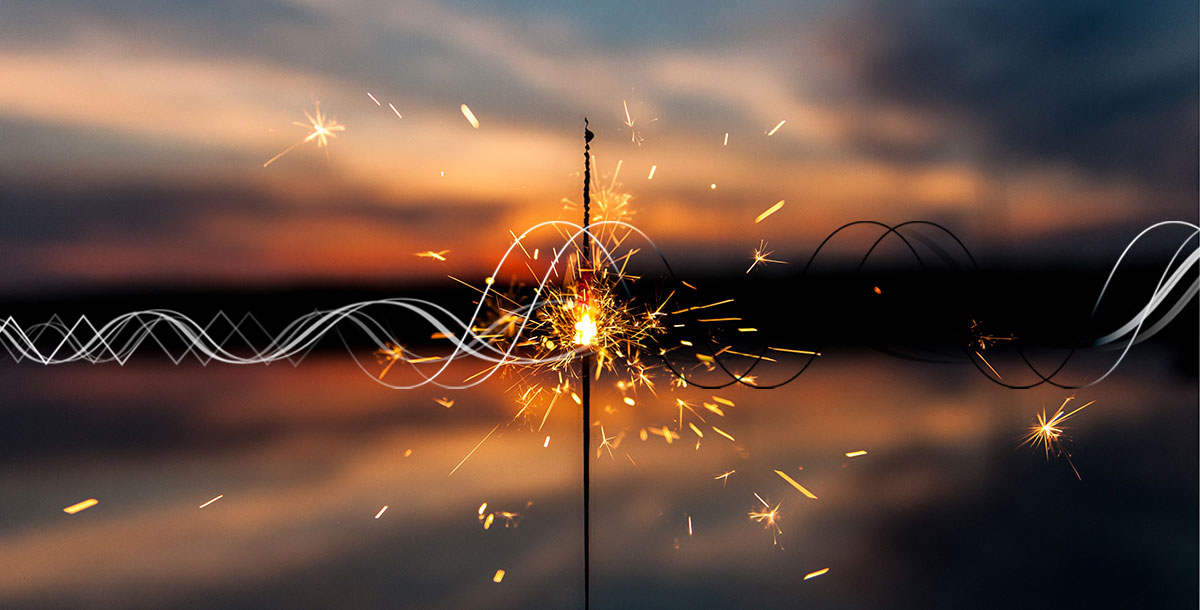
Introduction
This week, we have a guest post from Dr. Marco Turchi, head of the Machine Translation Group at Fondazione Bruno Kessler (FBK) in Italy. Marco and his team have a very strong pedigree in the field, and have been at the forefront of academic research into Neural MT. In this issue, Marco describes his recent work on spoken-language translation.
Overview
In this post, we take a look at spoken-language translation (SLT), a natural language processing (NLP) topic that aims at translating an audio speech in a source language into text in a different target language. A widely investigated topic, it is again receiving attention from the research community and big industrial players, thanks to the artificial intelligence (AI) revolution.Why Spoken-Language Translation?
Nowadays, the amount of audiovisual content produced and consumed daily by users has reached unprecedented volumes. This steady growth is mainly due to two factors. The first one is the continuous request for new content from the users, which has generated an unprecedented flow of videos and an increment in the time spent on watching them, mainly in “silent mode”. The second factor is the growing number of companies that focus their business on hosting and distributing digital videos (e.g. YouTube or Netflix). Nonetheless, almost all of the world's video content is in English, limiting the circulation of knowledge. This limitation calls for solutions that allow audiovisual content in English to be comprehensible in different languages.The deep revolution
Spoken-Language translation is a research area that has been addressed for years by cascading an automatic speech recognition (ASR) and a machine translation (MT) system. On the one hand, this approach has shown high potential and it has been widely used in the market. Its main advantage is the possibility of leveraging large quantities of ASR and MT data. On the other hand, cascade systems need to deal with several issues, in particular, the engineering required to maintain separate modules, the cumulative error propagation between the ASR and MT components and the lack of direct use of speech prosodic cues to improve the translation.
The ongoing AI revolution has created new research opportunities on SLT, where the cascade models are challenged by more efficient end-to-end deep models that directly translate speech signal from one language into text in another language without intermediate steps. Although neural end-to-end models are yet to confirm their worth in many application scenarios, new approaches are being presented at top conferences and in academic journals, showing the level of interest of the research community on this topic.
The Google recipe
The crucial aspect that is limiting research in SLT is the lack of training data to build a data-hungry end-to-end model. Recently, Googlers have published an inspiring paper that provides a recipe on how to build a neural end-to-end model:- leveraging large quantities of ASR and MT data, and
- being able to outperform cascade systems.