Issue #73 - Mixed Multi-Head Self-Attention for Neural MT
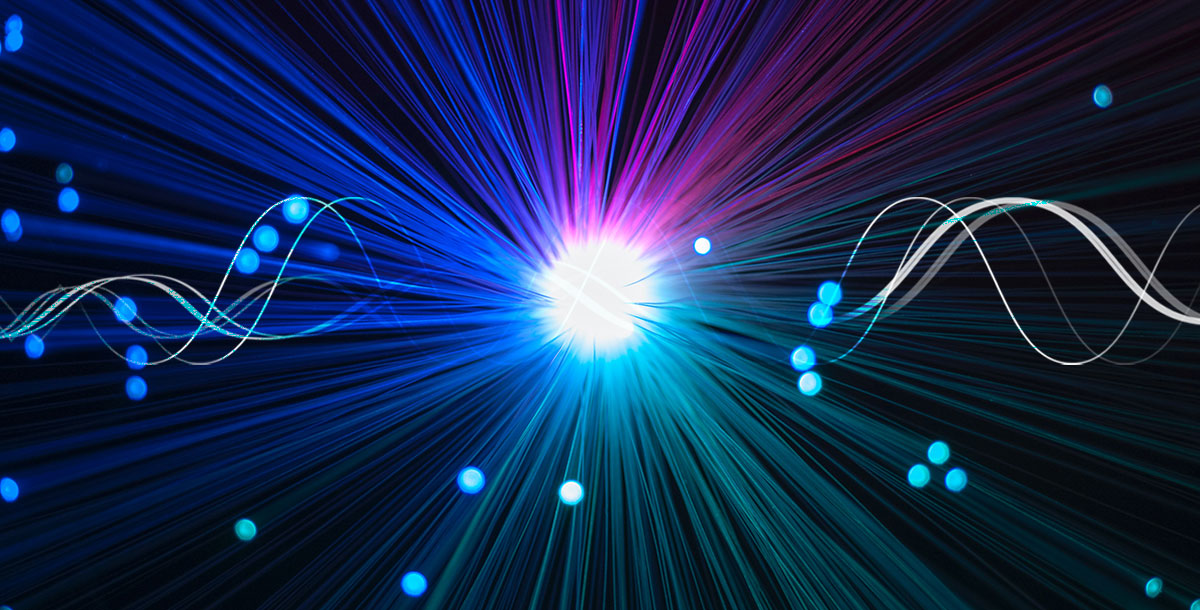
Introduction
Self-attention is a key component of the Transformer, a state-of-the-art neural machine translation architecture. In the Transformer, self-attention is divided into multiple heads to allow the system to independently attend to information from different representation subspaces. Recently it has been shown that some redundancy occurs in the multiple heads. In this post, we take a look at approaches which ensure that different heads can capture distinct features.
Multi-head Self-attention
Attention mechanisms selectively focus on specific parts of the sentence during translation. In self-attention networks (like the Transformer), the hidden states of each word are calculated by attending to every other word in the sentence. Self-attention thus relies on global information. Furthermore, the Transformer was designed with multiple attention heads to give the model the ability to attend to different parts of the word representation vectors in parallel. These different subspaces of the representation vectors contain, in principle, information on different word characteristics. Thus, in theory, by attending to different types of information, multi-head attention can capture different features. The multiple heads significantly improve the Transformer performance (in terms of BLEU score, about 1 point in the original paper about Transformers). However, no explicit mechanism ensures that different heads capture distinct features. There is actually recent evidence that some redundancy occurs in the multiple heads (for example Voita et al., 2019). Thus the majority of attention heads can be pruned without seriously hurting the model’s performance. Furthermore, multi-head self-attention has been observed to have difficulties to capture local information and sequential information.Mixed Multi-Head Self-Attention
Xu et al. (2019) propose an approach to leverage local and global patterns for Self-Attention Networks. This approach is extended by Cui et al. (2019), who add patterns to model forward and backward information. Concretely, the Mixed Multi-head Self-Attention (MMA) model proposed by Cui et al. is composed of four attention functions (see figure):- Global attention, which models the dependency of arbitrary words directly, as in the baseline transformer.
- Local attention, where the attention scope is restricted to the neighbouring words.
- Forward and backward attention, which attend to words from the future and from the past respectively, and thus helps the network to model sequence order.
[caption id="attachment_16585" align="aligncenter" width="1024"] The architecture of Transformer with Mixed Multi-Head Self-Attention[/caption] MMA enables the model to explicitly attend to different types of information in different heads, and thus improves the expressive capacity of multi-head self attention. The local, forward and backward attention functions are implemented by masks which restrict the attention to the desired scope.
In the work of Cuit et al., the different attention heads are aggregated by concatenation. Note that other strategies are possible. For example, Xu et al. aggregate the local and global attention heads with a gating scalar, which allows the model to quantify the contribution of the local and global representations.
Results
Cui et al. only evaluate the impact of MMA in terms of BLEU score. Globally the improvement over the baseline multi-head approach is about 1 BLEU point. However, the improvement is different depending on the sentence length: it is lower for short sentences (10 words or less) and higher for larger sentences. The explanation is that in small sentences, the difference between the mixed attention functions is reduced. With larger sentences, there are more distinct features to be captured. This result also gives evidence of the practical difficulty for the Transformer to model long-range dependencies, while in theory it is well suited for this task.
The effect of the scope of the local attention is also investigated. The scope is the number of words on each side of the current word to which the local attention function can attend. The best results are obtained for a scope of 1 word. This is the case for which the local attention is most different from the global attention.