Issue #80 - Evaluating Human-Machine Parity in Language Translation

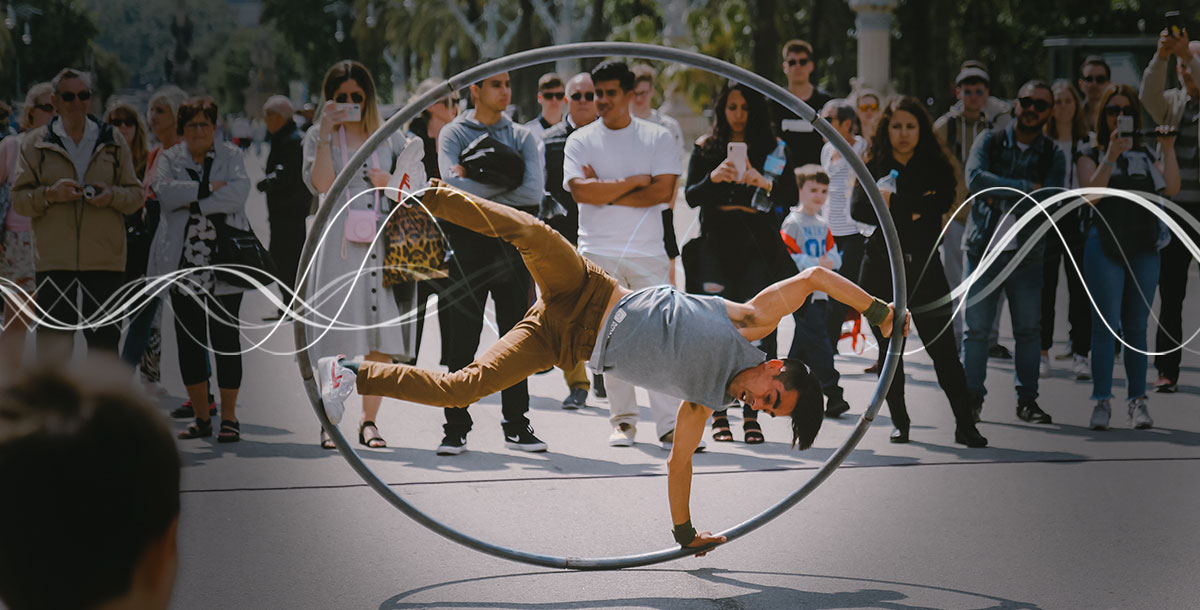
Introduction
Recent leaps in MT, including the evolution of neural MT (NMT), mean that translation quality is higher now than ever before. Recent studies - such as Hassan et al. (2018) - have gone as far as to suggest that the quality of this translation has achieved the level of - or even greater than - human translation: so-called “human parity”.
However, in order to consistently and effectively measure the progress of this technology, we must also ensure that the evaluation design is of an equally high standard. Läubli et al. (2020) examines three elements of language translation evaluation- choice of raters, linguistic context and reference translations - and recommends changes that could be implemented to provide more accurate assessments.
Choice of Raters
In their experiments, experts (professional human translators) and non-experts (crowd workers and MT researchers) were asked to rate three methods of translating Chinese sentences into English - one human, and two machine translations - relative to one another.
The original sentence is shown for reference, along with the previous and next sentence in the source text. This step was carried out to provide local inter-sentential context, in an attempt to address linguistic context. A translated sentence on its own may appear to be appropriate but, when viewed within the context of its source document, is not cohesive or coherent. Human translators take into account the context of a text when translating a sentence, and so an effective evaluation of MT systems should also include this.
Human translation was preferred over MT in terms of adequacy and fluency by both groups, although the experts exhibited a significantly greater emphasis in ranking score than the non-expert group. This suggests that less suitable raters were more tolerant to mistakes in output.
Linguistic Context
When investigating the effect of linguistic context on MT evaluation, raters scored machine and human translation on isolated sentences and sentences within their original document.
The results showed that preference was generally tied between human and machine translation with regards to adequacy, in the case of isolated sentences.
Interestingly, these scores shifted in favour of human translation when the test sentence was shown within its entire document. This highlights how context matters when it comes to good quality translation, and should be considered in any meaningful evaluation.
Occasionally, MT struggles with naming entities, as well as reordering and omissions. These errors are almost unnoticeable when viewed in isolated sentences, but given the context of previous sentences in a text it is clear they are incorrect. Human translation is again favoured for fluency over MT, in both isolated sentences and full documents.
Reference Translations
Non-native speakers may have issues with understanding text to be translated, particularly in unfamiliar domains. Their ability to generate fluent translations may also compromise the standard against which MT output is compared.
Limited resources must also be considered where humans are involved; fatigue, motivation and attention to detail are all variables that may affect the consistency of translation.
When fluency becomes priority over adequacy, human translation is not favoured over MT. Human translation is shown to drop important context words when proofreading MT output, in an attempt to make it look more natural.
Languages that have been back translated (e.g, English translated into Chinese and back into English) allows MT systems to achieve higher scores. "Translationese" is noted to be simpler and less varied than original source text. While this may be the only option for low-resource languages, it does not provide a fair basis for real-world evaluation.
Recommendations
- USE PRO RATERS: Professional translators appreciate the nuances of a translation, and thus are less likely to ignore the shortcomings of MT output.
- USE WHOLE DOCUMENT: As shown through experimentation, the context of a segment has a significant influence over the suitability of its translation.
- EVALUATE FLUENCY ALONG WITH ADEQUACY: Sentence-level adequacy between machine and human translation is largely similar, although MT falls short in grammatical aspects.
- AVOID HEAVILY EDITED REFERENCE TRANSLATIONS FOR FLUENCY: increasing fluency in post editing has been shown to further reduce adequacy, as important points of information are lost to accommodate stylistic changes.
- USE SOURCE TEXT: The use of translationese text places an unfair bias towards MT, as this is generally less complex.
In summary
Further experiments with more raters, language pairs and domains are necessary to strengthen the conclusions drawn from this research. The authors also note that the implementation of these recommendations will come at a high cost, particularly due to the hiring of professional human translators as raters and the longer evaluation time due to viewing translations within entire documents.