Issue #83 - Selective Attention for Context-aware Neural Machine Translation

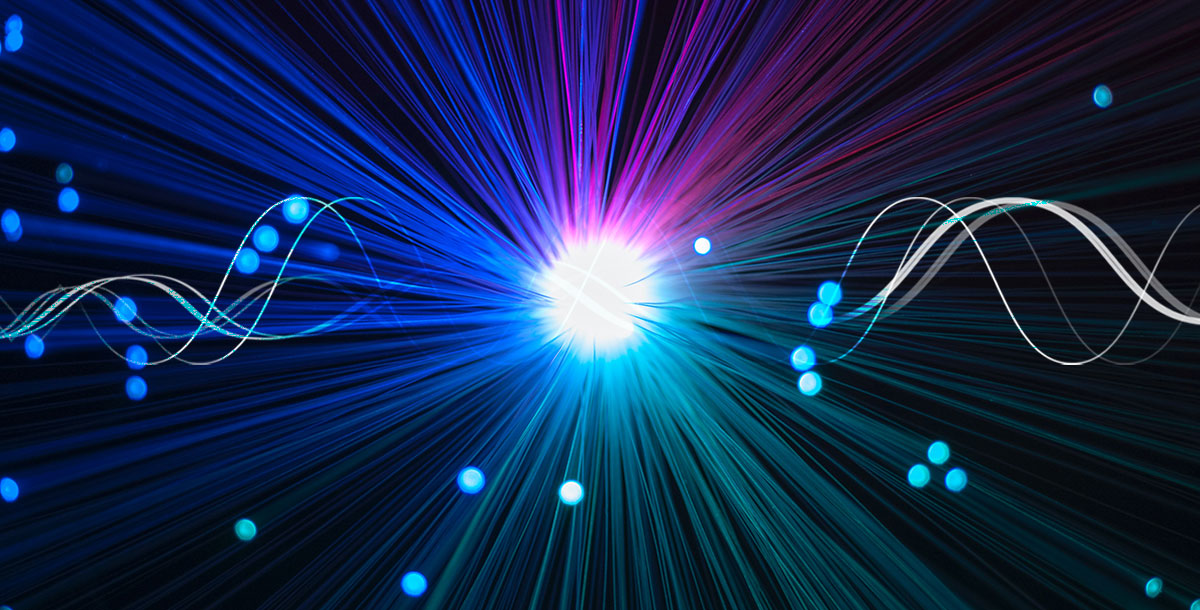
Introduction
One of the next frontiers for Neural Machine Translation (NMT) is moving beyond the sentence-by-sentence translation that currently is the norm, to a context-aware, document level translation. Including extra-sentential context means that discourse elements (such as expressions referring back to previously-mentioned entities) can be integrated, resulting in better translation of references, for example. Currently the engine has no awareness of something referred to in a previous sentence, which results in a great deal of errors, for example around the gender of referring expressions (mistranslations of ‘it’, ‘he’, ‘she’).
While there has been some work in context-aware or document level MT, integrating various aspects of discourse into machine translation, this is still not the dominant paradigm. Work on context-aware translation has been largely restricted to a few sentences (Miculicich et al 2018). There is an approach that is fully document level, although the context is static, computed once for the sentence being translated (Maruf & Haffari 2018). This research builds on that work. For a full survey on Document level MT see Maruf et al 2019.
Sparse Attention
In this blog post we look at a very interesting approach taken by Maruf et al 2019 which uses selective attention to focus on relevant sentences in the document as a whole, then attends to just the key words in those sentences. They do this by experimenting with a different attention transformation, sparsemax (Martins & Astudillo 2016), to identify the key sentences, which has the effect of dropping low probability sentences (while also being efficient).
Training the document level NMT model in this approach comprises two steps: Firstly, pre-training a standard sentence level NMT model. Secondly, optimising the parameters of the whole model, document and sentence-level. Their translation process also incorporates two steps: a draft translation using the sentence based model, then updates using the context aware one.
They experiment with modelling the document level context in a couple of different ways: a hierarchical attention module or flat attention one. The hierarchical attention model extends the traditional attention by adding another layer. To compute the weights they use sparsemax (Martins & Astudillo 2016) (which can output sparse distributions) instead of softmax, selectively attending to key sentences in an efficient manner. For word level attention they experiment with both soft and sparsemax. The flat attention model integrates the document context in a modification of the normal attention.
This document level context is then integrated into either the encoder or decoder of the NMT model:
- Either as monolingual context into the encoder, where the context is from the integration of source text words or sentences derived from the pre-trained sentence level model.
- Or else as bilingual context integration in the decoder, which integrates both source side context and target side context.
Results
Results both over context agnostic baselines and over context-aware baseline are better in terms of BLEU score, whether the document context was integrated into the encoder or decoder, with variations depending on the test set. Interestingly, the hierarchical attention model is always not significantly better than the flat attention one under standard evaluation methods. Given how unsuitable BLEU score is for measuring intersentential discourse phenomena, they also evaluate on contrastive pronoun test set, which specifically measures the accuracy of translating the English pronoun it to one of ‘es’,’er’ or ‘sie’ in German (Mülller et al 2018). Here their model surpassed the baselines, particularly as antecedent distance increased beyond 3 (ie. the pronoun refers to an entity 3 sentences previous), when their hierarchical model supersedes the flat one. Finally they include subjective evaluation where native German speakers were asked to judge adequacy and fluency of the 18 translated documents, finding that their model improves on the Transformer in both document level adequacy and fluency. In terms of qualitative analysis, we can see from the example below where the context-enabled model has improved pronoun translation: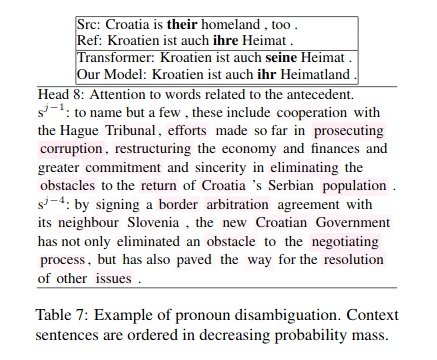