Issue #87 - YiSi - A Unified Semantic MT Quality Evaluation and Estimation Metric
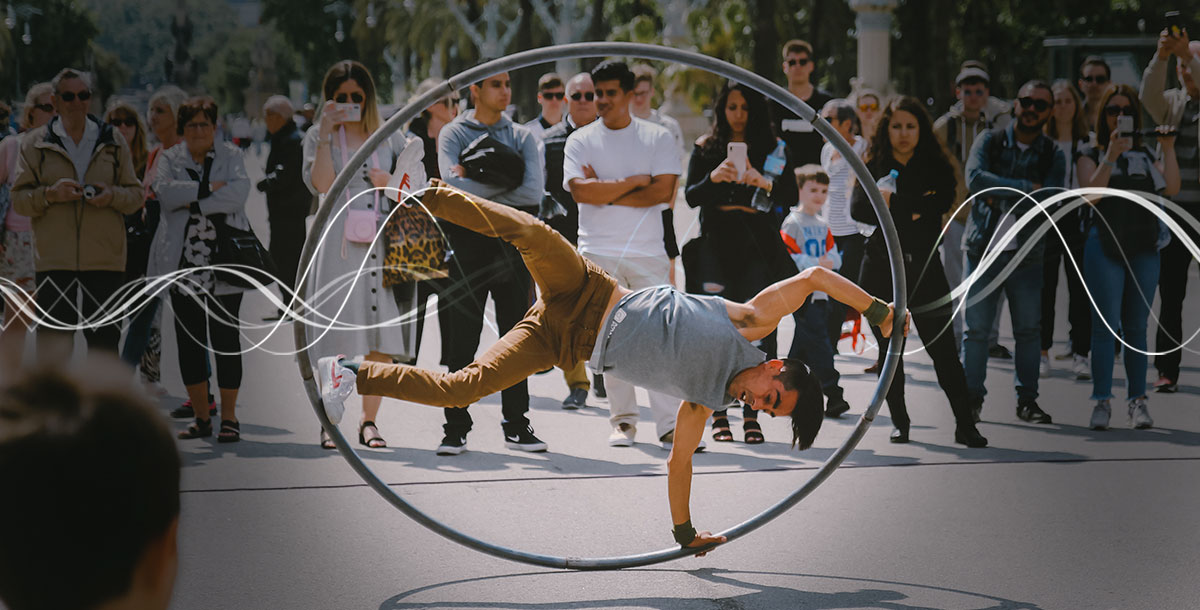
Introduction
Automatic evaluation is an issue that has long troubled machine translation (MT): how do we evaluate how good the MT output is? Traditionally, BLEU has been the “go to”, as it is simple to use across language pairs. However, it is overly simplistic, evaluating string matches to a single reference translation. More sophisticated metrics have come on the scene, including chfF (Popović, 2015), TER (Snover et al, 2006), and METEOR (Banerjee and Lavie, 2005). None of them attempt to evaluate the extent to which the meaning of the source is transferred to the target text. The first real attempt to incorporate a semantic element into automatic evaluation was MEANT (Lo and Wu, 2011). However, the fact that it requires additional linguistic resources made it less easy to use widely. YiSi (Lo, 2019) builds on that work, offering a range of flavours based on the level of resources available for that language pair. Interestingly, this includes a quality estimation component too, which allows us to measure the quality of the output without the need for a reference (a version previously translated by a human). In today’s post we examine how this metric, YiSi, measures the semantic quality of the output and we look at their results.
At a most basic level, a translation should transfer the meaning of the source text to the target text. A good MT quality metric should be able to measure the extent to which it does that. YiSi does this using a shallow semantic parser, which derives semantic frames and role fillers from the source and target texts. In other words, it extracts entities and their roles in a sentence from the source and target text, and compares them in a range of ways:
- Derives the logical form with shallow semantic parser,
- Aligns semantic frames extracted from source and target by comparing the lexical similarity of the predicates,
- Aligns the arguments by comparing the lexical similarity of the arguments (entities) in source and target texts,
- Computes the F-score of these aligned roles and entities, where:
- w(e) = lexical weight of e s(e,f) = lexical similarity of e and f
- where the definition of lexical similarity and weights depends on the version of YiSi used (see below).
Variants
YiSi-0:
- Requires no additional resources and as such can be deployed to any language pair
- Measures lexical similarity via longest common character substring
- Compares MT output and human reference
- Since both are same language, this is the inverse document frequency of the words from each of them in the reference document
YiSi-1:
- Requires an embedding model
- Optionally also a semantic role labeler in output language
- Measures the similarity between the MT and a reference by aggregating the lexical semantic similarity of the embeddings
- Where available it can incorporate shallow semantic structures to evaluate structural semantic similarity
YiSi-2:
- Requires a crosslingual embedding model
- Optionally requires a semantic role labeler in both input and output language
- Evaluates the crosslingual lexical semantic similarity of the source text with the MT output using bilingual embeddings
- Can also estimate the quality of the MT output without any reference- attempting to directly evaluate whether the MT output reflects the semantics of the source text.
- Can also be used for parallel corpus filtering