Issue #106 - Informative Manual Evaluation of Machine Translation Output

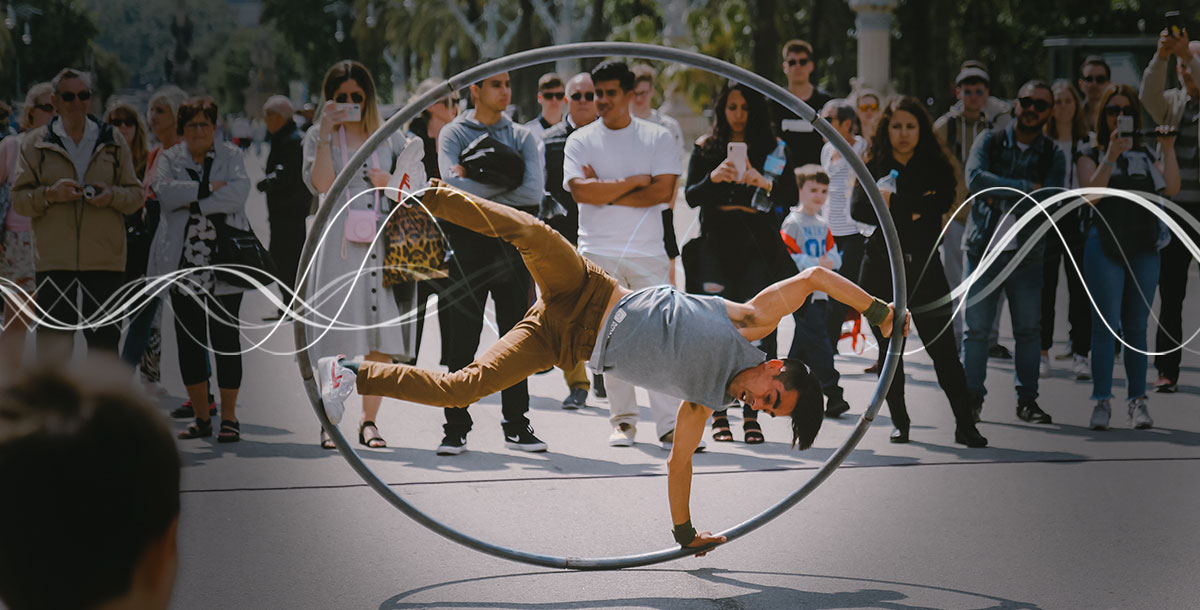
Introduction
With regards to manual evaluation of machine translation (MT) output, there is a continuous search for balance between the time and effort required with manual evaluation, and the significant results it achieves. As MT technology continues to improve and evolve, the need for human evaluation increases, an element often disregarded due to its demanding nature. This need is heightened by the prevailing tendency of automatic metrics to underestimate the quality of Neural MT (NMT) output (Shterionov et al., 2018). NMT evaluation is not a newcomer to our blog series and has been featured in a number of Neural MT Weekly issues e.g. #104, #92, & #90, to name just the most recent ones! But you can also check our #100 issue, where we summarised all of them. In today’s post, we focus on a human evaluation method recently proposed by Maja Popović and to be presented at the upcoming COLING 2020 conference in December. This tiered approach centers solely on the identification of problematic areas within MT output, which can be supplemented by an error analysis of highlighted issues.
Approaches
Human MT evaluative metrics are positioned on a broad scale, categorised by factors such as temporal effort, cost effectiveness and practicality of results. On one end of the spectrum sit established metrics including comparative ranking, direct assessment, adequacy and fluency among many others. On the other end of the spectrum sit methods such as post-editing and error classification. The downfall of the latter metrics however, is the extensive amount of both time and effort they require. Popović proposes an approach that potentially exists as a middle ground, requiring no additional effort than general assessment metrics yet providing informative results on a similar scale to coarse-grained error analysis.
Within this paper, the metric, applicable to all domains and text types, is guided by the evaluative pillars of comprehensibility and adequacy. English to Croatian and English to Serbian translations of IMDb movie and Amazon product reviews from Google Translate, Bing and Amazon Translate were evaluated by 13 computational linguistics students and researchers. Each text was evaluated by two annotators. Annotators were asked to differentiate between major and minor issues when assessing comprehensibility on source alone and adequacy with reference to both source and target. A summary of guidelines as given to annotators are as follows: Comprehensibility -
- Red - not understandable
- Blue - grammatical or stylistic errors
- XXX - omission (in corresponding color)
- Red - different meaning than source
- Blue - grammatical errors or sub-optimal lexical choices
- XXX - omission (in corresponding color), English correspondent in yellow
- Green - source error, corresponding translation in red or blue
Insights
Popović argues that her proposed methodology can potentially increase inter-annotator agreement (IAA) and computes an F-score and a normalised edit distance as a way of computing IAA which shows some promising results: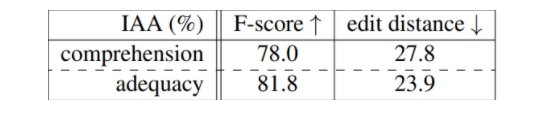
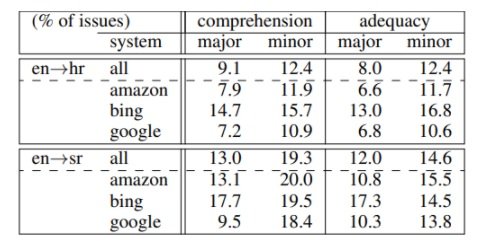