Issue #137 - Comparing Agreement in Sentence and Document Level MT Evaluation
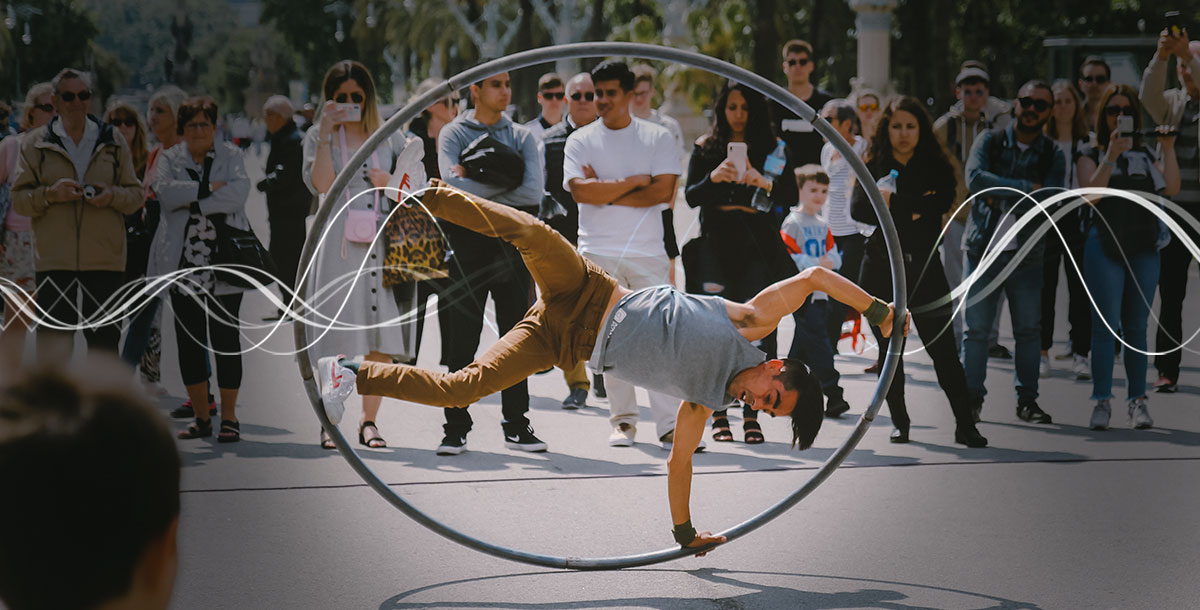
Introduction
To date Machine Translation has not made the transition to document level, and most systems translate one sentence at a time. This fails to take account of the surrounding context and the discourse issues involved, such as references to previously mentioned entities. However there has been some interesting research towards incorporating wider context, which we have investigated in previous blog posts (#15 and #98). Evaluating the ability of an MT engine to handle discourse issues typical of a wider context is something that has not been widely researched. Yet if document level MT is to progress, it is important to see the strengths and failings of different systems. In our blog post today we examine work by Castilho (2020) to compare inter annotator agreement (IAA) between quality assessments at sentence and document level. This matters because the IAA is generally regarded as reflecting the reliability of human judgements.
Document level context
Work by Castilho (2021) which assessed 300 sentences in 3 domains to determine the context span required to translate a sentence, found that over 33% of these sentences required context beyond the sentence level in order to disambiguate issues such as gender agreement. The illustration below taken from Castilho (2021) indicates why the context is important:
Figure 1: Examples of context span needed to solve gender and number issues in sentence 104 and 105. The parts in pink relate to the gender of the speaker, red parts relate to the number of "you" (singular/plural), orange parts relate to the gender of "you", and the green parts relate to the resolution of "it".
Previous work has highlighted that extra-sentential context improves assessments, as does employing professional translators (Läubli et al. (2018), Toral et al. (2018)). However the work we examine today directly focuses on differences in assessor agreement between sentence and document-level evaluation. This builds on findings by Läubli et al. (2018) for pairwise comparison, but this is the first comparison of IAA at document and sentence levels using fluency and adequacy scales.
Experiments
The experiment involved 5 professional English to Brazilian Portuguese translators, and focused on fluency, adequacy, error annotation and pair-wise ranking.
They evaluated 2 scenarios:
- sentence level (randomised sentences)
- document level, one doc at time, one score per doc
The datasets comprised the English corpora from WMT newstest2019, divided into 2 Test Sets of 32 documents per scenario, some of which are very short. The corpus was translated with Google Translate and DeepL for comparison. Each translator evaluated 500 sentences per scenario.
IAA was computed with Cohen’s Kappa, both weighted and non-weighted (where W accounts for degree of disagreement). They also computed (IRR) Inter-rater Reliability, and Pearson correlation between translators (details in paper).
Adequacy was assessed by considering how much of the meaning in the source appeared in the translation. Translators judged on a Likert scale of 1-4. Results (see table below) showed that IAA scores were higher at sentence level than at document level.
Fluency was similarly judged on Likert scale 1-4. Here IAA was again higher in the document-level scenario than sentence-level.
Error annotation was simplified to facilitate comparison of IAA, using 4 categories: Mistranslation, Untranslated, Word Form, and Word order. The results were assessed as binary (indicating whether or not there was an error) and type of error. If results were regarded as binary (i.e. whether or not there is an error), agreement was high, since the full text likely includes some kind of error. However, annotating error at document level is perhaps not granular enough for meaningful comparison.
Pair-wise ranking of the output from the 2 systems was performed. Output was randomly mixed and annotators asked to rate their preferred translation. Again, the sentence level scenario saw higher IAA. The authors point out that each system will have its own characteristics which may influence the preference of the translator.
Given that human evaluation involves humans (including in this instance, doubts over one annotator and the need to increment the number from 4 to 5), there is a difference in assessments across translators who will have subjective preferences that impact the IAA. Nevertheless, some tentative conclusions can be drawn. At document level evaluation this variation could be due to the fact that a document comprises many sentences which may be of varied quality. At sentence level it may be related to judgements due to ambiguity, or in terms of what constitutes an error. As the authors point out, we are still largely translating documents with sentence-level NMT systems. It would seem that to progress we need to find a reliable way of evaluating beyond sentence level, in order to see where the shortcomings are.
In summary
The work by Castilho (2020) represents a welcome (albeit small scale) attempt to start trying to pinpoint issues of agreement in document level evaluation, compared to sentence level evaluation, which has been the norm so far. It finds that document level evaluation leads to lower IAA for adequacy, ranking and error markup, although could be useful on fluency, if sufficiently defined. This is likely due to the fact that a document comprises many sentences which may be of varying quality. The challenge will be in reaching meaningful evaluation of different criteria with agreement as high as reasonably possible.