Issue #81 - Evaluating Human-Machine Parity in Language Translation: part 2
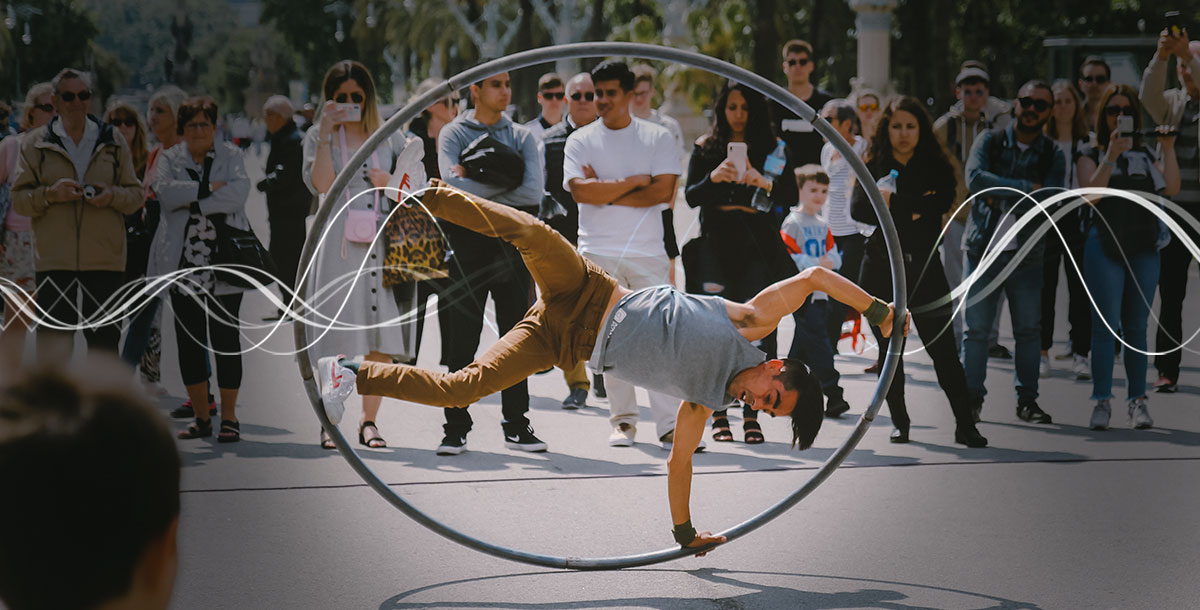
This is the second in a 2-part post addressing machine translation quality evaluation – an overarching topic regardless of the underlying algorithms. Following our own summary last week, this week we are delighted to have one of the paper’s authors, Dr. Sheila Castilho, give her take on the paper, their motivations for writing it, and where we go from here.
In the machine translation (MT) field, there is always great excitement and anticipation for each new wave of MT. In recent years, we have seen impressive claims by a few MT providers, such as Google (2016): “bridging the gap between human and machine translation [quality]”; Microsoft (2018): “achieved human parity" on news translation from Chinese to English; SDL (2018): “cracked" Russian-to-English NMT with “near perfect“ translation quality. The truth is that, not rarely, there is a great discrepancy between the high expectation of what MT should accomplish and what it is actually able to deliver.
Given the hype around NMT and the big claims that came with it, two independent studies (Toral, Castilho, Hu and Way (2018); and Läubli, Sennrich and Volk (2018)) re-assessed the claims of NMT reaching ‘human parity’ by Microsoft (MS) in Hassan et al (2018). It is worth noting that MS used the state-of-the-art (SOTA) human evaluation procedures and they were the only provider to open their evaluation and provide us with the data for the experiments.
What is “human parity”?
Human parity is a problematic term that has been widely used recently in MT evaluation. The problem with the term is that it implies that there is a single human translation (HT) for a given sentence, which we know is not true as humans can produce several translated versions for the same sentence (equally good or equally bad). Hassan et al’s definition of human parity is when a human judges the quality of a translation produced by a human to be equivalent to one produced by a machine; in other words, when the difference between the MT and the HT is not statistically significant, MT has reached human parity. But the problem with this definition is that, if the MT is proven to be “not different” from a reference (let us assume it is indeed a HT used as reference here), it only means that that MT system is comparable to that specific reference being used for testing, not to all possible HTs for that given sentence/text.Issues with SOTA human evaluation of MT
Both Toral et al (2018) and Läubli et al (2018) found a similar issue with the human evaluation of the MS system, namely, the lack of linguistic context, where raters prefer HT more consistently when given a wider context span, or even the full document. In addition, both studies disproved that the MT output was indistinguishable from the HT. Some other discoveries were also reported, such as the choice of raters – where we show that professional translators are able to assess the subtle differences between different translation outputs; and quality of the reference translations – where we show that translationese found in the WMT (Conference on Machine Translation) references was favouring MT, not to mention that some of the references were poorly translated.A set of recommendations for human evaluation of MT
During EMNLP 2018 (Conference on Empirical Methods in Natural Language Processing) where we were all presenting these findings, we met to discuss our research and decided to write a set of recommendations for a new SOTA MT human evaluation (but not without first running a few more experiments!). The result is the paper “A Set of Recommendations for Assessing Human-Machine Parity in Language Translation”, where we list a few recommendations in order to try to minimise the bias in the evaluation (for either MT or HT):- Choose professional translators as raters: They should translate the test sets from scratch to ensure high quality and independence from any MT engine, and conduct the human evaluation with fine-grained translation nuances taken into account.
- Evaluate documents, not sentences: When evaluating sentences in random order, professional translators judge machine translation more favourably as they cannot identify errors related to textual coherence and cohesion, such as different translations of the same product name.
- Evaluate fluency in addition to adequacy: Raters who judge target language fluency without access to the source texts show a stronger preference for human translation than raters with access to the source texts. Moreover, raters prefer human translation in terms of fluency while they find no significant difference between human and machine translation in sentence-level adequacy.
- Do not heavily edit reference translations for fluency: Aggressive revision can make translations more fluent but less accurate, to the degree that they become indistinguishable from MT in terms of accuracy.
- Use original source texts: Our results show further evidence that translated texts tend to be simpler than original texts, and in turn easier to translate with MT.