Issue #109 - COMET- the Crosslingual Optimised Metric for Evaluation of Translation

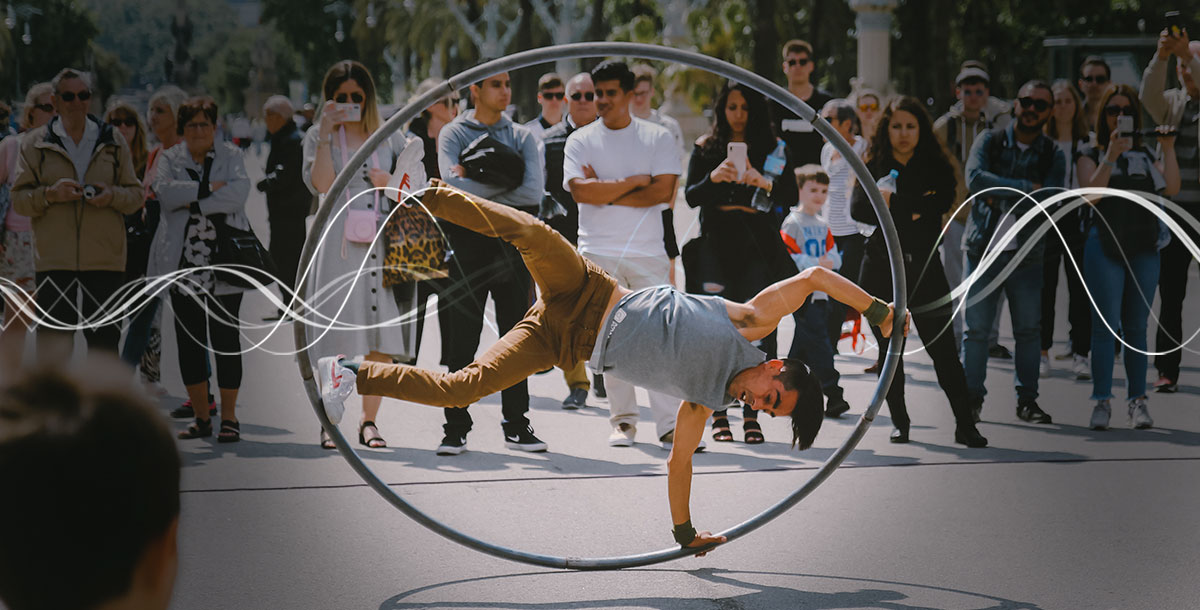
Introduction
In today’s blog post we take a look at COMET, one of the frontrunners this year at the annual WMT metrics competition (when looking across all language pairs) (Mathur et al., 2020).
Historically, Machine Translation (MT) quality is evaluated by comparing the MT output with a human translated reference, and using metrics which increasingly are becoming outdated (Rei et al., 2020) (see also our recent blog posts #106, #104, and #99 on evaluation).
Rei et al. (2020) point out that the two major challenges highlighted last year at WMT19 were a failure to accurately correlate human judgements at segment level, and a failure to differentiate the best MT system. They set out to address these challenges in their work. Given recent work in Quality Estimation achieving high levels of correlation with human judgement, Rei et al. (2020) incorporate the source language as input to their MT evaluation models, and evaluate its effectiveness.
Human judgements of MT quality are generally Direct Assessments (DA), Human-mediated Translation Error Rate (HTER), or Multidimensional Quality Metric (MQM) (detailed in Rei et al., 2020). They use data from three different corpora to train 3 models that estimate different types of human judgements: the QT21 corpus, DARR corpus, and their own MQM annotated corpus (all detailed in Rei et al., 2020).
COMET
The COMET framework (Rei et al., 2020) incorporates 2 architectures:- An Estimator Model (left hand side below)- trained to regress directly on a quality score, minimising the MSE (Mean Squared Error) between the predicted scores and the quality assessments, whether these be DA, HTER or MQM.
- A Translation Ranking Model (right hand side below)- trained to minimise the distance in embedding space between 2 different hypotheses (i.e. potential MT outputs) and both the reference and original source.
Common to both is the Crosslingual Encoder - which forms the primary building block and incorporates a pretrained crosslingual model, XLM-RoBERTa (Conneau et al., 2019). It is used to produce an embedding for each token and each layer, for source, MT output and reference, mapping them into a shared feature space.
Given the different linguistic information that the different layers can capture, both architectures include a pooling layer, which pools information from the most important layers within the network and into a single embedding for each token using a layer-wise attention mechanism. Averaged pooling is applied to derive a sentence embedding for each segment.
Estimator Model
This model integrates combined features from the sentence embeddings (output from the encoder and pooling layer) such as element-wise source and reference product, and source and reference difference. These are concatenated to the reference embedding and hypothesis embedding, then passed to a feed-forward regressor. They train 2 Estimator models, COMET-HTER and COMET-MQM.Translation Ranking Model
This model receives an input tuple consisting of source, reference and 2 hypotheses of different rankings. The tuple is passed through the encoder and pooling layer resulting in a sentence embedding for each segment. They then compute the loss in relation to source and target (detailed description in Rei et al., 2020). Finally, the distance is converted to a similarity score between 0 and 1. To investigate the value of incorporating source as input, they trained 2 versions of the Ranker model, one with reference only, the other with reference and source.Results
The results on WMT19 Metrics DARR corpus for all 3 models are displayed in the tables below, reporting Kendall’s Tau correlations with language pairs where English is the source. Here, all three COMET models outperform other metrics across the board.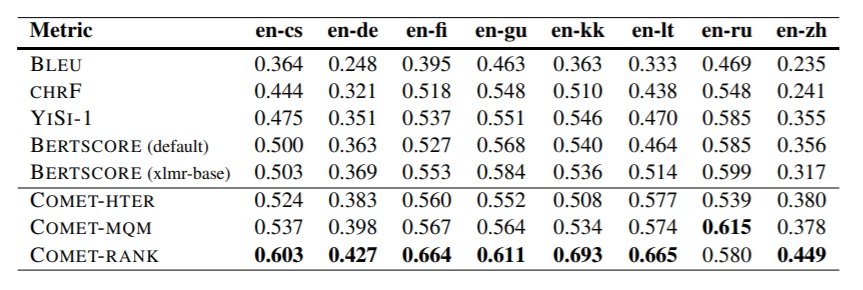
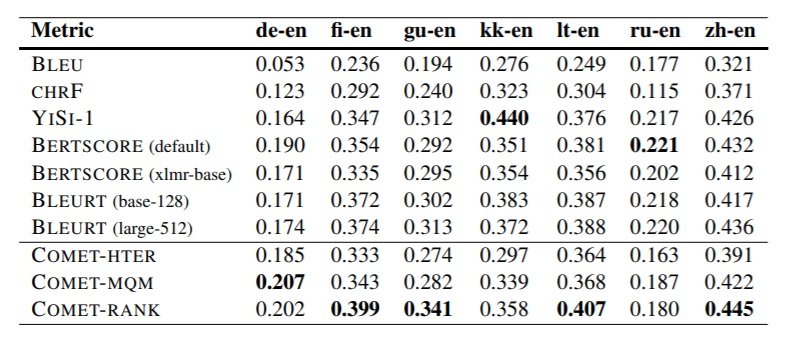