Issue #141 - All that glitters is not gold: revisiting scientific credibility

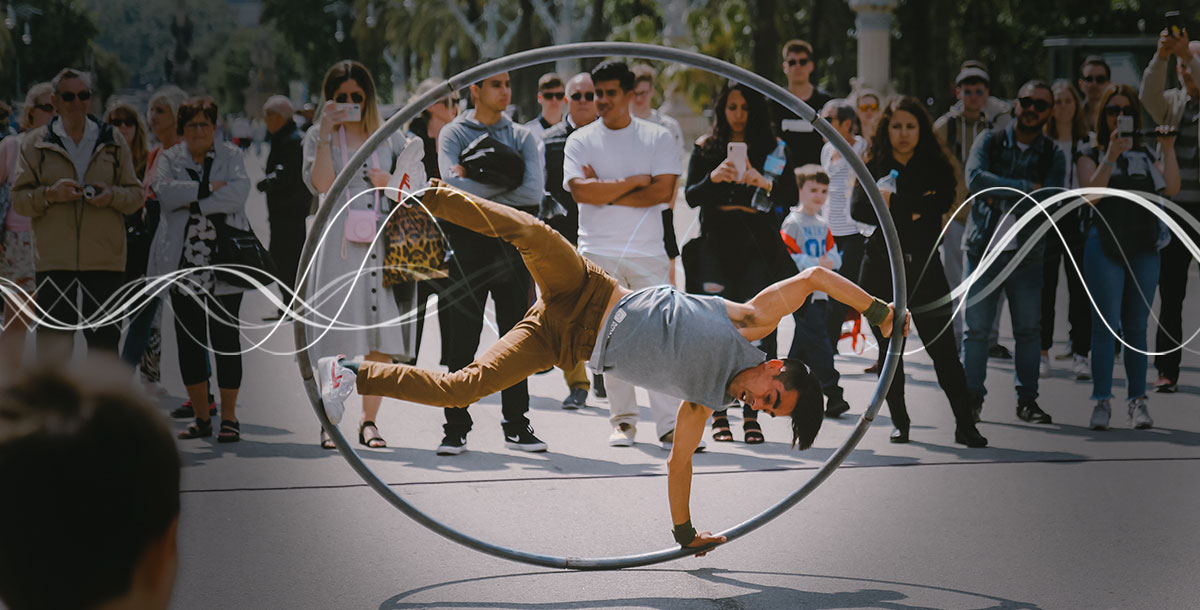
Introduction
The Meta-Evaluation
- What metrics are used to evaluate the MT quality? Variants of the same metric were excluded, as well as metrics only measuring specific aspects of a translation (e.g. the translation of pronouns).
- Does the paper include a human evaluation of the MT output? Here, they only considered this to be the case if the evaluation was done holistically. If there was a human evaluation just checking one aspect of the translation (e.g. pronoun translations, or right term translations), it was considered that no human evaluation was performed.
- Do the authors perform any kind of statistical significance testing of the difference between metric scores? If this was not explicitly stated in the paper, it was deemed that no statistical significance testing was performed.
- Are comparisons with previous work done by blindly copying automatic scores? That is: did the authors re-compute the scores from previous work or simply copied what was reported in a paper?
- Was SacreBLEU used? If it was not stated, they assumed it was not.
- Did the authors confirm that the systems compared used exactly the same pre-processed training, validating and testing data? This question was applied for papers that copied results rather than reproducing previous work.
Trends detected (and potential issues with them!)
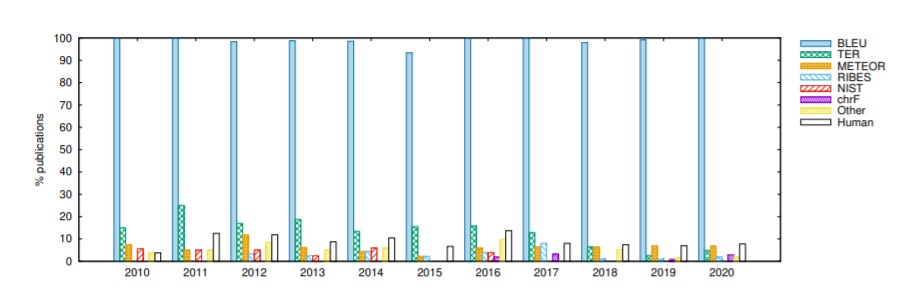
This is a surprising tendency, given the criticism towards the metric, particularly in the advent of NMT. As the authors point out, just in the last decade alone, 108 new metrics have been proposed to evaluate MT results, and some of those not only correlate better with human judgements, they are also simpler to compute. The danger of relying entirely on BLEU scores (specially if no statistical significance testing or human evaluation is additionally performed), is that the best system will not necessarily rank first. The authors illustrate this by running a simple evaluation of WMT20 submissions using SacreBLEU and chrF. Depending on which metric you look at, a different system is ranked first.
2. The absence of statistical significance testing. Statistical significance is computed to ensure that experimental results are not a happy coincidence. Traditionally, MT research groups would use statistical significance testing to assess whether differences observed across the scores of various systems are not coincidental. Sadly, while in 2011 up to 65% of the papers reported the statistical significance of the results obtained, Marie et al. observed a sharp decrease in its use since 2016. This is a risk, as performing this test indicates whether a particular difference between two scores is trustworthy or not, irrespective of how big or small such a difference is. Figure 2 shows this trend.
Figure 2: Percentage of papers testing statistical significance of differences between metric scores.
3. The comparison of incomparable results from previous work. It is in the nature of research to compare results against previous work. This allows researchers to save time and money (and we should add CO2 to the environment!). However, one can opt for taking the output of MT systems and running their own evaluation script, or blindly copying results reported from a paper. The risk of copying results is that the evaluation method may not be comparable, as most metrics, BLEU included, have various parameters and also depend on pre-processing techniques to both the source and the reference text. An attempt to standardize the way BLEU is computed was proposed by Post (2018), who provided the scientific community with a tool, SacreBLEU, to compute BLEU scores in a standardized way. Moreover, the tool provides a stamp with the specific configuration used to compute the scores that allows for the reproducibility of the evaluations performed. The authors show that since its appearance, SacreBLEU seems to have gotten traction, but that even in 2020 papers that copied BLEU scores did not always use it. Figure 3 illustrates this.
Figure 3: Percentage of papers copying scores from previous work ("Copied scores"), using SacreBLEU ("SacreBLEU"), and copying scores without using SacreBLEU ("Copied w/o SacreBLEU").
To illustrate the risk of not using standardized ways of computing automatic metrics, the authors do a small experiment using different processing techniques on the source and the target, such as lowercasing and normalizing punctuation marks. Depending on the way the metric is computed, different systems will be ranked differently and hence the scores are not truly comparable.
4. The reliance on comparison between MT systems that do not exploit exactly the same data. The last issue that the authors identified is the fact that even when working on the same language pair, and maybe even starting off with the same data sets, the training set, the tuning/validating set and the test set may be set up differently in an experiment. Each of these sets could be considered independent variables that affect the outcome of the automated metrics. The risk is that changing datasets, even if it is just a small change (e.g. a different tuning or test set is used) will yield different results and hence the differences observed in metric scores may be due to the change in the dataset itself, the method/algorithm used to train the engine, or a combination of both. The authors further analyzed which papers were comparing systems that had used datasets that could not be considered identical, and the results are surprising, as we can see in Figure 4. In fact, almost 40% of the papers from 2020 compared results across systems that used different datasets.
Figure 4: Percentage of papers that compared MT systems using data that are not identical.
As they did with the previous trends and problems, in the paper the authors illustrate the impact of this by running their own MT experiments and showing a comparison that cannot be considered like for like.
Proposal for improving MT meta-evaluations moving forward
As we have seen, Marie et al. (2020) did a thorough analysis of 769 papers and identified four main risks for the MT research and its credibility. To alleviate such risks and help revert the trends they observed, they suggest the following 4 questions to be posed to researchers, paper authors and reviewers, each targeting one of the trends observed:
- Is a metric that better correlates with human judgment than BLEU used or is a human evaluation performed?
- Is statistical significance testing performed?
- Are the automatic metric scores computed for the paper and not copied from other work? If copied, are all the copied and compared scores computed through tools that guarantee their comparability (e.g., SacreBLEU)?
- If comparisons between MT systems are performed to demonstrate the superiority of a method or an algorithm that is independent from the datasets exploited and their pre-processing, are all the compared MT systems exploiting exactly the same pre-processed data for training, validating, and testing? (Marie et al., 2020)
To ensure credibility, all four questions should be answered with a ‘Yes’.
In summary
Marie et al. (2020) offer a very thorough meta-evaluation of the way in which MT evaluation has been performed in MT research over the last decade (2019-2020). Their research is eye-opening, as it confirms in a scientific manner trends that have been observed in the industry and research arena over the years. BLEU is still the de-facto standard in our industry, but we need to question how it was computed and encourage the use of other metrics and, if possible, human evaluations, to reach credible conclusions. When comparing results across research papers, it is important to bear in mind that differences may be due to the issues highlighted above. As readers of MT research and developers of MT engines, we know how important it is to not only rely on BLEU and to have a critical eye when reading research papers and comparing systems. In fact, to provide the best possible services to our clients, we at Language Weaver always use a set of various metrics and include human evaluation as part of our standard processes. After all, all that glitters is not gold!