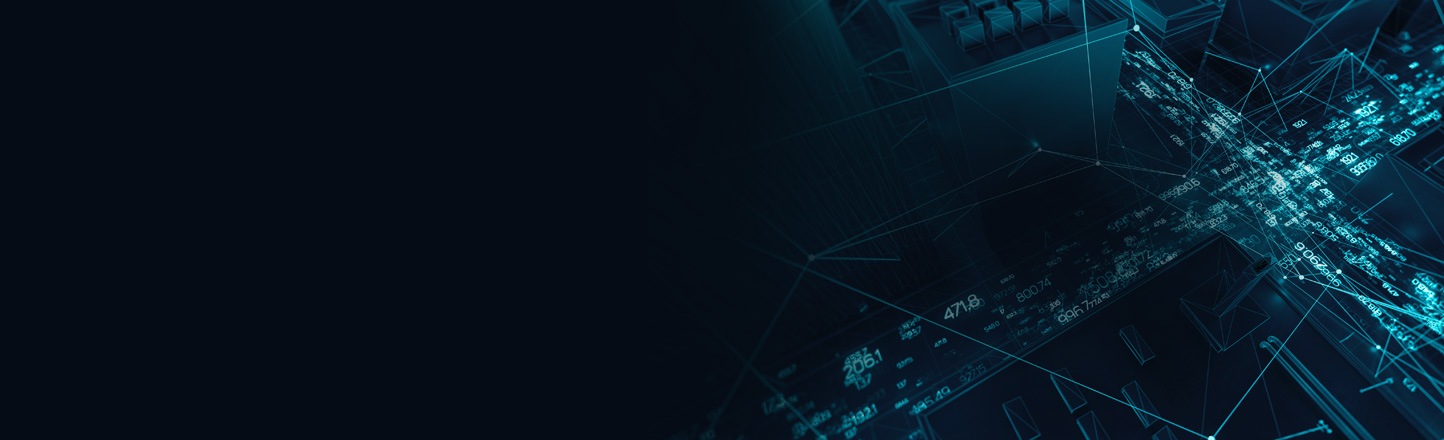
The RWS Blog
A place to keep updated on the latest industry trends and developments.
Filter by:
Filter by:
Categories
Tags
{{totalItems}} Results
No blogs could be found