Unlocking organizational knowledge: Why structured content is essential for effective AI
01 Apr 2025
6 mins

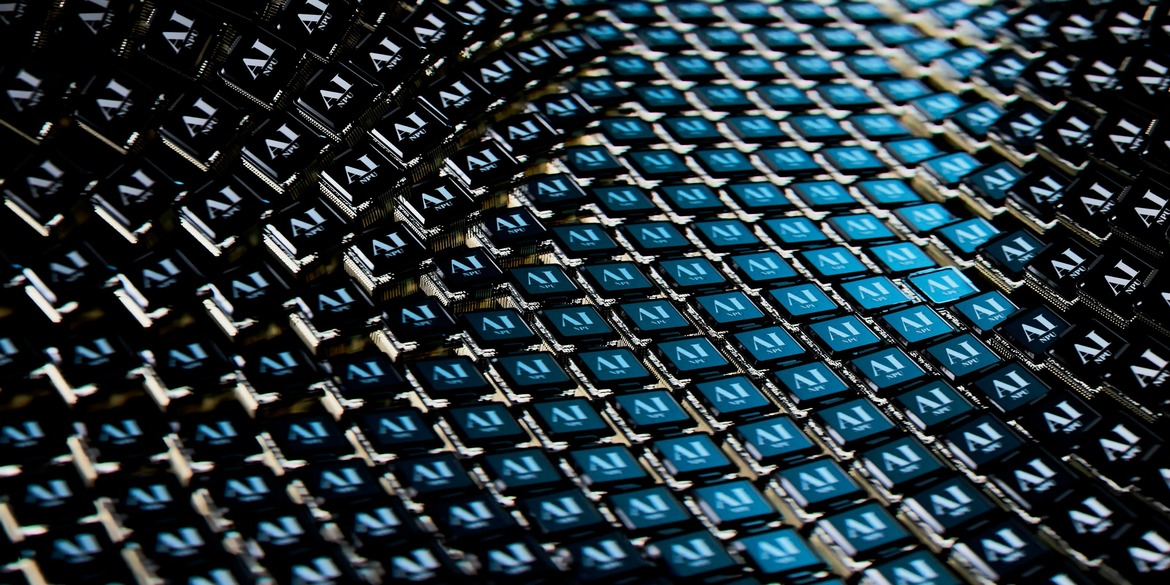
I will never forget sitting in a meeting several years ago with one of the Big Four accounting firms. Let’s call them Company X. When one of their senior executives started the meeting, they displayed a slide that read: “If only Company X knew what Company X knows.” The point of course was that the firm sat on vast stores of knowledge, but making that knowledge available for their teams at the point of need was an unsolved problem.
This meeting took place before the emergence of AI based on large language models (LLMs), and you might think that an enterprise LLM implementation, perhaps based on a Retrieval-Augmented Generation (RAG) model, would have solved Company X’s problems. Now that they can equip teams with the tools to ‘converse’ with their content, they must know. Problem solved.
But it turns out that the truth is far more nuanced.
RAG reality check: when ‘just add AI’ fails to deliver
Company X was an early mover with LLMs and in fact one of the first to bring a private LLM instance in-house. They were also very early to turn to RAG as the most promising approach for unlocking the vast potential of their internal knowledge.
Their data store was document-oriented and composed of unstructured Microsoft Word documents and PDFs. Company X quickly discovered that RAG produced responses that were good-seeming (in other words, fluent, confident, etc.), but not actually good enough for high-stakes audit and accounting decision-making.
The story so far sounds like the golden ring just out of reach: a magic answer machine that confidently returns answers you can’t quite trust. Back to the drawing board? Not quite. Company X already had the building blocks of a fix in place, it just needed to go back to the source.
By ‘source’ in this case, I mean the data store they were using as their knowledge base -- the one made up of Word documents and PDFs. Fortunately, this company had invested in an XML-based structured content authoring and management system that was the true system of record for all those files.
This brings us to the punchline. By using XML-based content (in this case based on the DITA standard) as the data source for its RAG-based system, the company has been able to nearly eliminate inaccuracies due to outdated and ungoverned content, and the AI context challenges of working with content at the monolithic document level.
Let’s dive into how and why this change of source material made all the difference.
The issue with unstructured content
First, consider the challenges associated with using unstructured content (raw PDFs, Word files) as source content in a RAG system. These challenges are largely centered on the content preparation phase, including:
- Arbitrary or inexact chunking and loss of context: RAG systems typically process data by breaking it into smaller chunks for embedding and retrieval. With unstructured content, this chunking can be arbitrary, splitting critical information that should be grouped and severing contextual relationships. The result is that the LLM receives fragmented pieces of information, making it difficult to generate coherent and accurate answers.
- Challenges with precise understanding: Unstructured content lacks markers of explicit semantic meaning. While LLMs excel at understanding individual phrases, they struggle to discern what blocks of content are ‘about’ and the relationships between different blocks of content. This leads to irrelevant or incomplete retrieval, as the system struggles to match the user's query with the intended information.
- Limited metadata and search capabilities: Unstructured documents usually lack consistent and rich metadata. This makes it challenging to filter, refine, and target the retrieval process effectively. Without meaningful metadata, the RAG system has limited ability to understand what the content is about, the content's purpose, audience, or relevance to specific queries.
- Challenges in content governance and maintenance: Managing and updating unstructured content within a RAG system can be cumbersome. Version control, identifying outdated information, and ensuring consistency across a large corpus of unstructured documents become significant hurdles.
The power of structured content to the rescue
Embracing structured content authoring, in this case an XML-based architecture using DITA, offers a compelling solution to these challenges and lays a solid foundation for high-performing RAG systems.
Topic-based modularity: DITA drives the creation of content as small, self-contained, and self-described topics. This modularity makes chunking for RAG more effective. The content is ‘chunked by design’ as each content module represents a complete and coherent piece of information with an explicitly described purpose.
Semantic structure and predictability: XML formats like DITA allow (and often require) authors to tag content with semantic meaning. Elements like <task>, <concept>, and <reference> clearly indicate the type of information being presented. This semantic predictability allows the RAG system to understand the content's purpose and retrieve relevant information based on the user's intent.
Rich metadata capabilities: Structured content models inherently support the inclusion of rich and granular metadata at various levels – topic, section, or even specific elements. This metadata can include information about the product or service in question as well as its audience, version, keywords, and relationships to other content. This significantly enhances the precision and effectiveness of the retrieval process.
Facilitating knowledge graph integration: Structured content, especially XML-based formats like DITA, can be easily transformed into knowledge graphs. Knowledge graphs represent entities and their relationships in a structured format, enabling more sophisticated retrieval and reasoning capabilities within the RAG system.
Improved content governance and auditability: Authoring in a structured XML format and storing that content in the right kinds of knowledge systems naturally leads to better content governance, auditability, version control, and maintainability.
A blueprint for AI success
Company X’s foresight in investing in an XML-based approach to structured content management paid off. They could quickly switch from using a content set designed for human readability (PDFs and Word documents) to a content set designed from the outset to be consumed by machines – what we now call ‘agents.’ This allowed the company to move into production with their RAG-based knowledge system confident that they were delivering enhanced knowledge retrieval quality, reduced hallucinations, improved efficiency, and better content governance.
The lesson for other organizations is clear. While adopting structured authoring practices and tools might require an initial investment, the long-term payoff is undeniable. Preparing your content to deliver on the full promise of a RAG-based AI system is the foundation for a smarter, more efficient, and more knowledgeable organization for the future.