Practical Uses for AI Beyond Machine Translation
30 Apr 2019

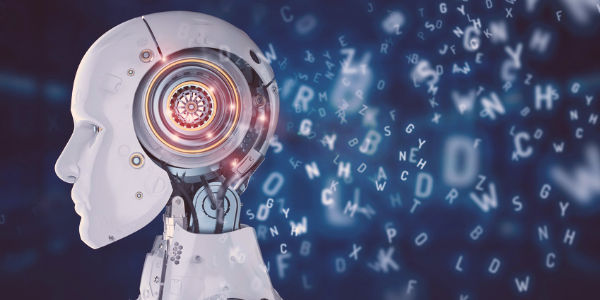
How artificial intelligence is shaping the localization industry is a subject we approach from many different angles.
Generally, though, we’ve found that those interested in AI tend to fall into three camps: those who are suspicious and fearful of it (Will AI replace humans?), those who directly develop the technology (How can we improve AI?) and those who are optimistic about its potential even if they don’t know what that looks like (How and when should I deploy AI, and what for?).
If you’re in camp three, we have some insight we think you’ll find useful. Jim Compton, Manager of the Partnerships Program at RWS Moravia, recently wrote an article for MultiLingual Magazine about the “killer applications” of AI that can solve practical problems in several areas of localization—not just machine translation. Below is a summary of his ideas (and your free copy of the full article).
AI: The story so far
First, some context: what has AI already helped us achieve? AI has always been part of the localization culture. Beyond machine translation (MT)—which we’ve been using since the 1960s—we’ve been using Translation Memory, concordance searching, terminology recognition, optical character recognition and other applications of AI for some time. The question now is how to apply AI to even more localization challenges, which of course have evolved just as quickly as AI itself. Our goal of crushing language barriers hasn’t changed. But the continued shrinking of the world and the rise of digital communications and other global megatrends have made it harder to do—and many agree we need AI to get there. Jim likens the use of AI in loc to having extra hands on deck. “What would you do with 150 extra engineers?” he asks, or in other words: how would you solve these challenges if you didn’t have human resources limitations, whether they be in translators, project managers, copywriters, solution architects or account managers?Rethinking how to apply AI
It’s best to approach this question by looking at specific situations you’ve found difficult to solve. When have you been forced to waste resources? When did you have to compromise on a solution because of human-based limitations? One of these scenarios might sound familiar:- You archive valuable transaction data after completing a project, only to have to re-learn the same lessons in later, similar projects.
- You estimate the price of the project with a “finger in the wind” approach—and sometimes find it to be inconsistent with a similar, previous project.
- After developing a solution to what appears to be a “unique” client challenge, you find out that another team already developed another (better) solution to a similar challenge months ago.