LOC TALK: Why NMT post-editing matters
30 Sep 2019
7 min read

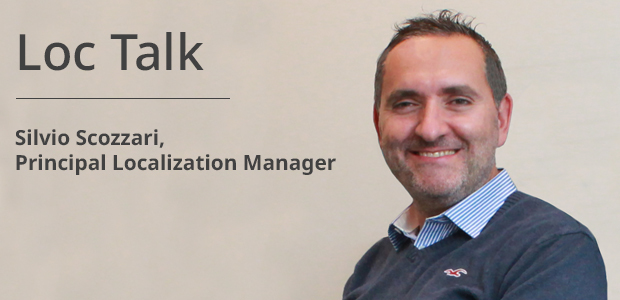
In this second instalment of our Loc Talk blog series, RWS Localization Manager Silvio Scozzari talks with industry veteran and long-time RWS employee Rodrigo Fuentes Corradi about the development and growth of machine translation and how it’s changing the future of the localization industry.
Silvio: Thank you for taking the time to talk to me Rodrigo about artificial intelligence and, specifically, machine learning. Can you tell us a little about yourself and what your role is within RWS?
Rodrigo: As you know, I have been with RWS for 16 years now. I progressed through the Language Services organization and then moved to the Machine Translation (MT) part of the company in 2007. My main focus was to make MT Post-Editing (MTPE) operational in terms of a quality-oriented deployment process and, thus, commercially viable.
RWS has grown from translating around 40 million words of MT when I started to over 300 million last year. Also when I started, RWS was using a form of MT that was rules-based and focused on trying to replicate language rules. Later we switched to statistical MT, as did the rest of the industry. This technology was based on statistical models that reduced the ramp-up time for MTPE deployment. The latest incarnation of MT technology is based on artificial neural networks, which pushes our industry deeper into the realms of AI and Machine Learning. So basically, I have overseen much of the transformation of the language service provider (LSP) part of RWS, and it is just like many other parts of the business - no two years have been the same!!!
Silvio: It’s certainly interesting to be speaking to an industry expert with such extensive experience in MT – and to hear that last year RWS translated over 300 million words with MT is pretty impressive! Many of us are aware that in recent times MT has become an essential resource to help businesses deliver an effective global customer experience, reach worldwide audiences faster and at a lower cost than human translation. However for those readers who aren’t overly familiar, can you explain simply what MT actually is?
Rodrigo: To start with, it’s worth stating that when we refer to MT we are talking about the underlying technology. We can use MT technology for many use cases but Language Service Providers (LSPs) predominantly use the MT Post-Edit use case. Put simply, MT Post-Editing is designed to make professional translators more productive. RWS creates MT models (still often called engines) by taking bilingual data, such as Translation Memories, and training iterative MT models to get to the best MT solution. In terms of how this works with the production workflow, it is pretty straightforward. Customer files are processed via computer assisted translation (CAT) tools or workflow tools like translation management systems (TMS) or WorldServer. The file is leveraged with full and partial matches and new words, normally those under 75% matches, are now leveraged with MT output.
Silvio: Thinking back to when I first started in the localization services, over 21 years ago now, MT has come a very long way. Can you explain the different types of MT available and how MT technology has evolved over the years?
Rodrigo: The challenge of automated translation has been around for a long time. The first incarnations of MT were rules-based systems, based on actual language rules and dictionaries. Developers tried encoding syntactical information, but due to the many rules and exceptions for each language, these systems were fragile, took a long time to develop and did not scale.
Rules-based systems were later replaced by statistical MT systems that used bilingual corpora and algorithms to produce translations. This signified a shift towards introducing machine learning (ML) to solve the problem of language, rather than humans creating systems based on language rules.
Now, we are experiencing the change from statistical to Neural Machine Translation (NMT). Instead of writing algorithms or rules to make decisions or trying to program a computer to “be intelligent”, the NMT approach teaches computer systems to make decisions by insights, or extracting information from large data sets.
Silvio: You’ve just touched on a buzzword there, Rodrigo. We’re hearing a lot about NMT, which is fast becoming known as the new powerful MT algorithm. How does the NMT approach differ from rule or statistical-based MT methods you’ve just explained and what unique benefits does NMT bring over and above anything else we’ve seen before?
Rodrigo: Like you say, there has been a massive focus on NMT. RWS rightly pointed out from the start that NMT has a unique architecture and properties that define it as a revolution rather than an evolution. With statistical MT, you design algorithms so that the system learns translation rules from existing content, usually an aligned translation corpus.
NMT instead learns meaning from a translation corpus. When we talk about Machine Translation and Neural MT, we need to understand that MT and NMT are part of the much wider arena of Artificial intelligence (AI). Machine Translation itself is a subset of Natural Language Processing (NLP), which is one of the hardest problems to solve within the AI framework.
Neural networks are developed to mimic the human brain, which is something that applies to all areas of Machine Learning. An NMT system learns from observing correlations between source and target texts and modifies itself to increase the likelihood of correct translations. For example, this means that you start with a network of nodes, or neurons, and the connections between the nodes. The nodes start with default values so when you input text from the training material, it is fed through all the default settings and results in output text. This output is then compared to the correct translation in the training material. First time round, it probably won’t be a perfect match, so the default settings are adapted and the process is repeated until the ideal settings are created. The key message to emphasize is that the Neural MT system modifies itself to reach the ideal settings without human intervention. Isn’t this amazing?
Silvio: It certainly is amazing and incredible to see how far MT technology has come in a relatively short space of time. One question I get asked a lot by my internal customers is around the suitability of MT for different content types and target languages. With the advances in NMT, can we now consider all target languages and content types to be suitable?
Rodrigo: One of the main benefits of NMT is that it can deal more effectively with more challenging content and languages than statistical MT. Another important benefit is that NMT can process whole sentences rather than breaking a source sentence into smaller chunks, then translating and reconstructing the output sentence like a jigsaw puzzle. This processing of the whole sentence captures greater context and meaning, leading to a more fluent and precise translation. For languages where the benefits of statistical MT were limited, this ability to process language more completely is particularly important. Good examples are languages with different grammatical sentence structures like German and Dutch.
In the diagram below you can see that NMT quality improvements now allow for post-editing of content types that were previously considered unsuitable. On the other side of the scale, quality improvements also mean that more content may be considered for raw MT without human involvement.
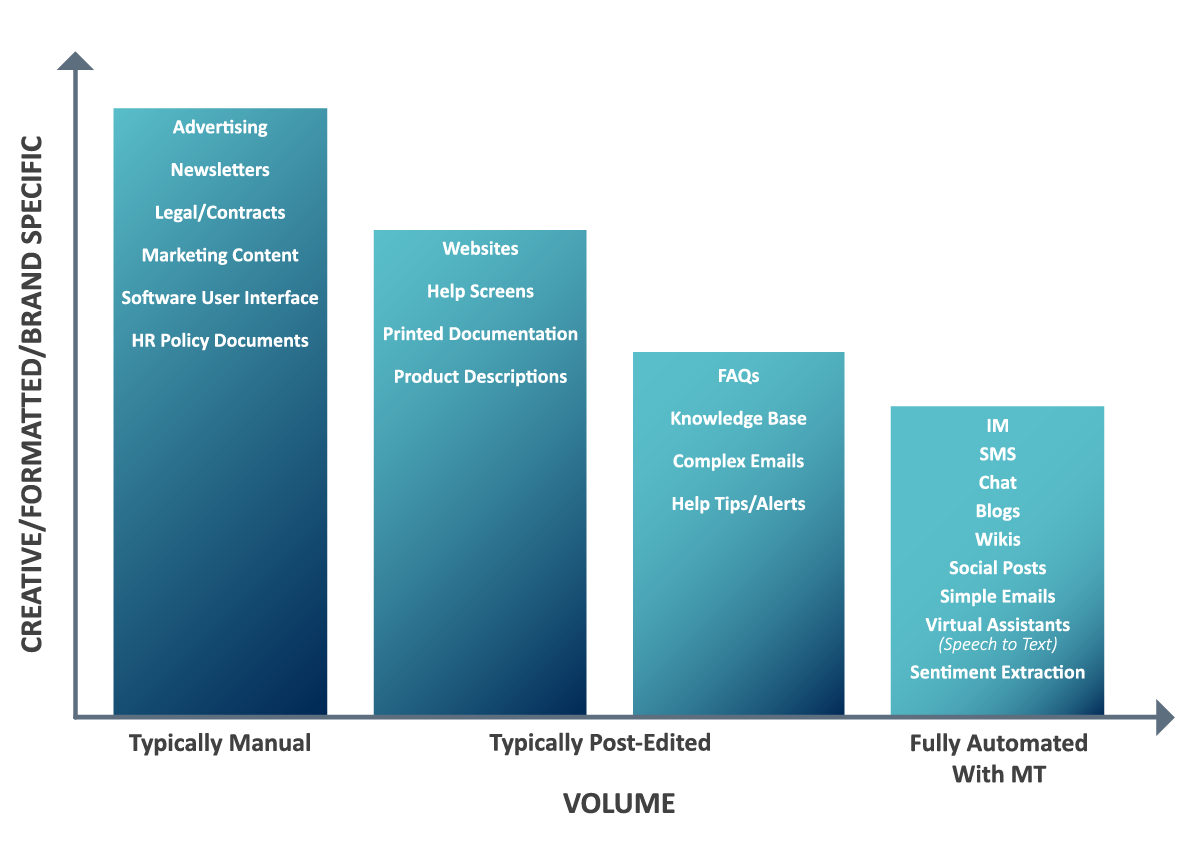
Silvio: As you know MT has become a hot topic of conversation and many businesses are now considering getting on the MT bandwagon to translate more content faster and at a lower cost. But what reassurances can you give to the trusted Translator, who may feel that they are becoming an endangered species as people point to MT as a replacement for their skills?
Rodrigo: I think this an important question because at the heart of the conundrum lies content. What content needs to be developed, what content needs to be translated, what its purpose is… The point is that our customers need to deliver their content to consumers the best way possible, and this includes cost efficiency.
Content is constantly changing in today’s fast-moving markets. What does this mean for translators? Faced with the growing use of and need for MT, translators need to continue to adapt and keep up with the latest innovations, in particular NMT. NMT really is a game changer when it comes to output quality, even for language pairs that were once considered to be tricky.
It’s important to emphasize that MT is not a threat to the profession of translator, but rather an opportunity to learn and grow. Yes, MT means change, but not in a bad way - Technology promotes new and different ways of working and gives rise to new linguistic roles and profiles.
Silvio: That's certainly reassuring to hear. From what you’ve explained, it’s clear that Translators should turn what some perceive as a threat into a new opportunity to widen their skills, particularly through post-editing work. Let’s now talk about RWS customers. There may be some reading this blog who haven’t considered using MT to translate their content until now. For those who are keen to use MT, how straightforward is it to get started?
Rodrigo: RWS has been fine-tuning and streamlining the MTPE process for many years now, making MT increasingly accessible for our customers. Using our experience and methodologies developed by our in-house R&D team of computational linguists, content can be evaluated for MTPE suitability very efficiently. Then, with pre-sales consultancy and technical account management, we can create a realistic roadmap for customers.
Most importantly, we can audit all of a customer’s enterprise content. As I said earlier, MTPE is just one use case for the technology. By looking at all content as a whole, we can create a strong, secure and personalized content strategy, hopefully with embedded MT technology for their global employee and customer base.
As we know, when employees use MT freeware, there can be huge security risks. Our latest Language Weaver product looks to eliminate these risks with its intuitive interface and the ability to adapt terminology and render our NMT output with even more accuracy.
Silvio: Having been through the MT on-boarding process myself for RWS content, I can vouch that getting started isn’t as daunting as it may first appear. As you well know Rodrigo we’re fortunate that RWS has some of the brightest minds in the industry when it comes to MT. Saying that, some readers may be concerned that before they can start to apply MT they may not have sufficient data or linguistic assets to build a new customized machine translation engine. Is there any way around this?
Rodrigo: RWS regularly increases the scope of our generic MT solutions by harvesting and optimizing data, so this is no longer a barrier to entry. This, combined with the extra potency of NMT, means that an increasing amount of content is now suitable to build an engine. RWS can also harvest data for a specific customer, if desired. The most important thing is to have an open conversation and set realistic milestones and goals.
Silvio: So what I’m hearing, Rodrigo, is that with the right content type, target languages and engines, it is possible to achieve good quality translations with MT. But since quality is all-important, how do you ensure that the content you plan to translate with MT will meet quality expectations?
Rodrigo: That’s an important question. It is key to evaluate the potential future performance of MT when it comes to the MT post-edit use case. RWS MTPE testing must demonstrate a credible gain over human translation to motivate skilled translators to accept PE assignments. The tests must be secure and produce easily understood, transparent and credible results. RWS's integrated testing environment and tools create valid and representative test beds using real customer content to easily determine the productivity of post-editing MT output online. This environment also provides detailed analytics, such as the speed of manual translation versus post-editing.
To underpin our integrated platform, skilled computational linguists are used to perform tests. As project and domain experts, they know what quality levels are required for the final deliverable. They also oversee the process, analyze the data and deliver relevant and predictive observations on the MT performance. Honed over a number of years, our thorough methodology brings together a strong combination of RWS subject matter experts.
Silvio: Over the years I’ve worked with several of these computational linguists that you’ve just talked about and I agree that they are true experts and are there to guide customers through the MT testing process while providing credible recommendations based on their evaluation and testing findings. Finally, one last question, Rodrigo. How has RWS’s history and early investment in MT changed things for the better, not only for our company but for the industry?
Rodrigo: Recognizing the importance of Machine Translation for localization very early on gave RWS a real head start on understanding and developing the technology. Ongoing investment in our proprietary MT solution has enabled RWS to provide thought leadership and training that includes an online Post-Editing Certification program for in-house and external translation teams. We regularly attend localization conferences and help academic institutions future-proof their translation programs.
RWS has been a trailblazer in Machine Translation for almost 20 years now, always working with the latest technology and now showcasing our proprietary Neural MT solution. Our technology has been refined in collaboration with our in-house translation teams, who provide direct and insightful feedback that guides the development team. As a result, RWS’s MT technology is fully integrated into the translation experience and includes a wide range of features designed for translators to effectively post-edit.
Thank you for helping us understand more about the history and development of MT, Rodrigo! And if any readers out there would like to learn more, please visit. Stay tuned for the next instalment in my Loc Talk blog series.