Do massively multilingual machine translation models help business functions get massively multilingual faster?
20 Nov 2020
8 min read

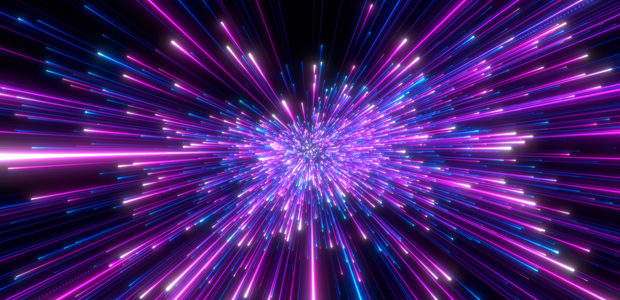
Technology is in a constant stage of change. This is especially true for Artificial Intelligence (AI) where every day we see the impossible transform to the every-day. Within machine translation (MT), a form of AI, researchers are continually looking for ways to make automatic translation faster, more accurate, and broader.
SDL has been at the forefront of innovation, pushing the evolution of machine translation from rule-based systems, to statistical models, and now to neural machine networks. We have seen the dramatic improvement in accuracy and fluency with the development and adoption of Neural Machine Translation (NMT) 2.0. Recently, I came across a research article touting a new approach for machine translation based on Massively Multilingual Translation (MMT). This approach is being developed by the Facebook AI team and while it’s an interesting approach, it has drawbacks and limitations that may cause it to be a less-than-optimal fit for those looking at machine translation as an enterprise software.
What is Massively Multilingual Translation?
Typically, translation models are trained for one language pair at a time to ensure the best accuracy and fluency; this is the prevalent machine translation methodology in the market today. Massively Multilingual Translation uses a large amount of training data across many languages to produce a single model that can be applied to any language pair. Those that are working with this claim that the results are just as good if not better than the bilingual baseline approach, especially for low resource languages. The theory is that the method is able to apply learnings across languages rather than being focused on a single language pair. While this is an interesting development and may with time open up new possibilities, it is not a methodology that would materially improve the outcome for enterprise machine adoption. And there are some drawbacks to this methodology that may not be readily apparent.
Massively Multilingual Translation (MMT) begins with a premise that there are vast amounts of training data for any and all language pair combinations. That is, there is just as much content to train models that can instantly translate from Uzbek to Hebrew as there would be to train French to English (or English to French). That may be true if the content you need to translate is common—chats about food, entertainment, current events, short bits of information – the content that would be relevant and useful to a Facebook user. However, that same approach may not work if the body of content you need to translate is technically complex—contracts, patents, documentation, corporate policies. While the researchers note that training high and low resource languages together help the model benefit from what can be describes as economies of scale—that may not actually apply to language nuances. The vernacular and technical vocabulary may be vastly different and mixing the languages may produce less useful results.
Why Massively Multilingual Translation isn't the answer for enterprise environments
MMT proponents note that the method has a positive impact on BLEU scores. For low resource languages—languages that aren’t frequently translated as a pair and don’t have a lot of unique training content—a five point improvement in BLEU scores isn’t unusual. For a consumer application, that may be a significant achievement since the accuracy bar may be fairly low. For a business application, a BLEU score isn’t enough to capture the ROI and a five point improvement may not be worth the added complexity of implementation that would be introduced with a single model for all, versus expertly trained bilingual models.
For example:
- A single model makes it harder to isolate issues in individual language pairs and adjust them without impacting all operations. A language pair-specific approach means that errors and re-training can focus on one model without affecting the rest of the business. This also allows customers to test changes on isolated languages and ensure success before changing others.
- Many enterprise customers require custom-trained models, in order to ensure that the MT engine preserves their brand voice and their translation guidelines. (This is why SDL introduced Adaptable Language Pairs in June 2019). Adaptable Language Pairs create customer models by taking the generic model and then using customer data to adapt it. If the generic model is an MMT, adaptation becomes much more difficult and computationally expensive.
- One of the promises of AI is that of self-learning systems. For Machine Translation, this indicates the ability of a translation engine to assimilate feedback, and immediately change its behavior in ways that make it more consistent with that feedback. SDL introduced this feature in SDL Machine Translation Edge 8.5. Such real-time adaptation from relatively little feedback is much more challenging to obtain for a large MMT model with billions of parameters.
The main issue that MMT solves is breadth of languages. Training languages one pair at a time can appear daunting if the goal is to achieve the breadth equal to what MMT can achieve with a single run. However, there are ways to achieve breadth that addresses low resource languages without resorting to MMT.
Get Involved
Language pair chaining is a method whereby language pairs are chained together to add breadth. SDL implemented language pair chaining in its SDL Machine Translation Edge deployment in January 2020. Customers are able to chain language pairs together and deploy new models easily. This same capability is now part of SDL Machine Translation Cloud where users are able to automatically apply chained models to achieve a breadth of language pairs well beyond 2,000 combinations.
There is a 14 day free trial available for SDL Machine Translation Cloud where those interested can try this, and other features included in our award-winning neural machine translation software.