Redefining self-service experiences
12 Nov 2021
5 mins
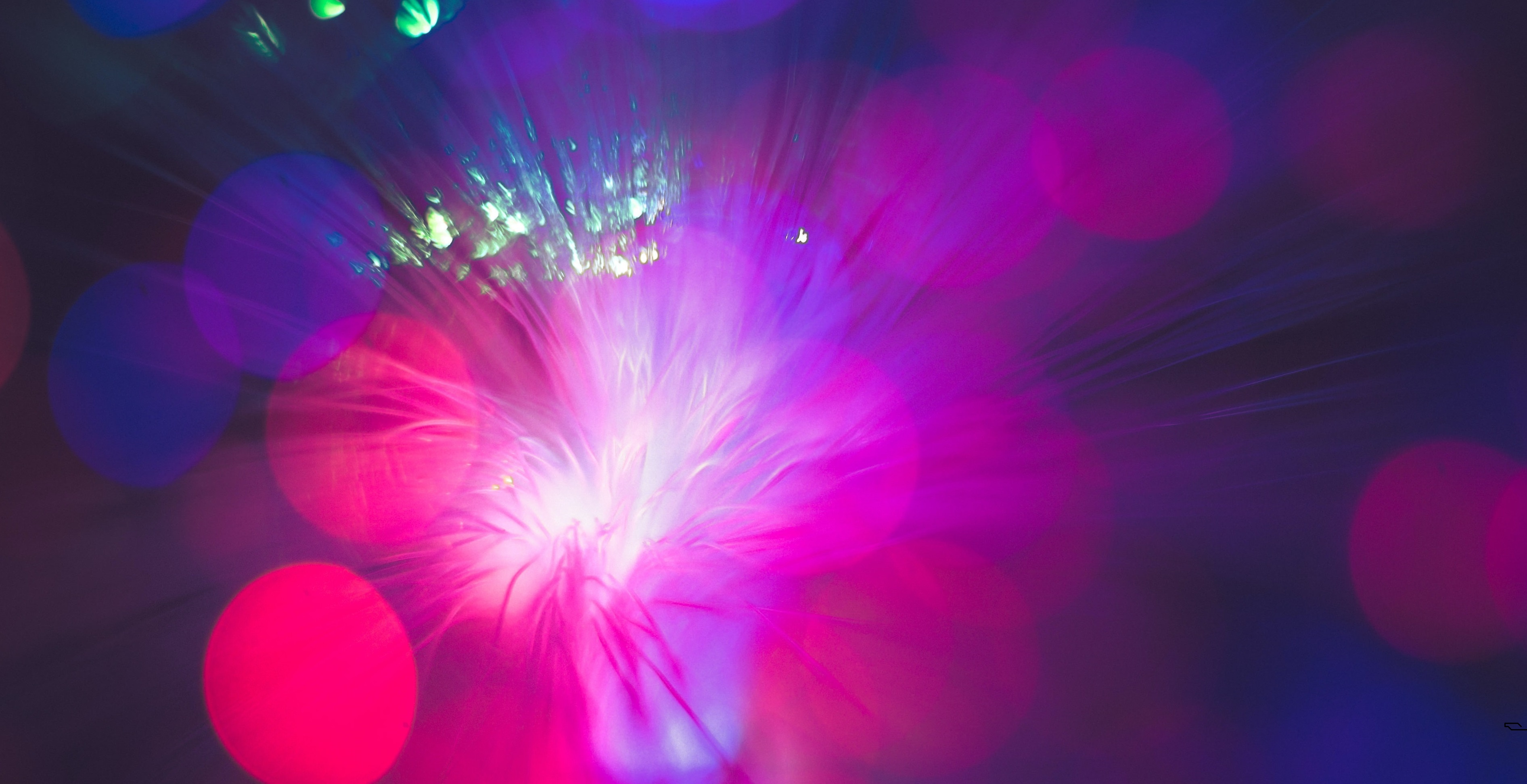
In the face of continually rising customer expectations, a focus on user intent in digital spaces is becoming paramount. When customers search for products or information, they want accurate, relevant answers – quickly – irrespective of the digital touchpoint. Search engines work with this mindset, and it's expected from business websites and applications – both for self-service and agent-assisted support.
Customer service as a business differentiator
Customer-centricity is ever more critical in these times of remote work and increased online interactions. Often it's the only true differentiator, as commoditization advances rapidly. With products and services becoming easier to replicate, scale and distribute, customer service becomes the way to stand out.
It's an area more than ready for progress, because bad customer service experiences are so common. Customer emails take too long to be addressed, customer issues remain unresolved, chatbots fail to respond to the question asked or give an unrelated answer – all leaving the customer feeling unimportant and frustrated.
'All' customers want is an experience that feels like a great human interaction – even when no human is involved. They're looking for something personal, demonstrating knowledge, adaptability and nuanced communication, maybe even empathy or creativity.
So how do you create a great human-like experience for customers when no human is involved? How do you mimic the personalization and meaningfulness of a human-to-human engagement through self-service interactions?
User intent and semantic AI
Understanding user intent is a decisive factor when it comes to designing modern customer self-service strategies for enterprises.
It starts with a solid knowledge base, containing the content you've identified as being relevant to customers' most common questions. Naturally the more content you capture, the easier it will be for a self-service tool such as a search engine, chatbot or conversational UI to extract a relevant response when faced with a customer query. But if the knowledge base and self-service tool are not very sophisticated, there's a high likelihood of failure to understand the user intent: the very reason why so many self-service experiences are so disappointing. Too often, for example, they rely on exact matches between query and answer, and something as simple as a synonymous search query fails to elicit a relevant response.
To take things up a notch and get closer to understanding user intent, you can use a feedback loop and machine learning (ML). The ML algorithm will learn from the actions of users – what they do and don't click on, for example – and the feedback they provide about how useful (or not) the answer was. This will enable it to deliver more relevant responses in future, improving the effectiveness of self-service by learning what users want – what their intent is – when they ask specific things.
But ML alone isn't enough to get to a human-like 'understanding' of user intent. It's very reliable in some circumstances but falls short in too many. It will fail whenever there isn't enough past behaviour to make good predictions, nor can it guess at the equivalence of synonyms or avoid other pitfalls of language. It doesn't really understand anything at all.
That's why we recommend the addition of a knowledge management model, specifically a knowledge graph, to the self-service mix. A knowledge graph allows enterprises to tag their content in ways that expose meaning (capturing synonyms and other nuances of language) and all sorts of relationships between different types of information. With the right kind of knowledge graph system, you'll also improve the quality of your knowledge base through taxonomy governance and automated classification suggestions.
All of this turns your knowledge base into a sophisticated asset that you can use to step up self-service from being enhanced only by ML, to being powered by semantic AI.
What do we mean by semantic AI in this context? We mean that when a self-service AI-powered search engine, chatbot or conversational UI turns to a knowledge graph to find an answer to a user question, it finds enough context to resolve ambiguous language, and enough direction to move beyond the most obvious starting point to find a more relevant, or complete, answer.
In other words, it comes as close as an AI can get to 'understanding' what the user's question meant, or what their search intent was.
Using semantic AI to transform customer service
Because semantic AI is key to understanding user intent, it's fundamental to delivering an excellent customer self-service experience.
It's hardly surprising, then, that according to IDC's Analytics, AI, and RPA Services Survey (May 2020), the most significant percentage of analytics, AI and RPA service buyers said they had already deployed knowledge graphs (36%), with another 14% piloting knowledge graphs to help manage relationships between data elements.
The creation of a good knowledge graph can be significantly facilitated by a component content management system (CCMS): a CMS that holds content at a granular level, as small components that are combined when we want to create a traditional document. This is because the more granular, or specific, content is, the more precise the knowledge graph can be, and the more likely it is to expose relationships that can be used to create new inferences and insights.
With a comprehensive and precise knowledge graph as your foundation, all it takes to deliver the human-like self-service interactions that customers want is to add AI. Semantic AI can use the understanding and insight enabled by the knowledge graph, together with its own learning abilities, to deliver personalized recommendations and answers during self-service interactions. IDC predicts that by 2026, 20% of all AI solutions will combine deep learning with symbolic methods (such as a knowledge graph) to create robust human-like decision-making.
To get started yourselves, it helps to have a solution that combines CCMS and semantic AI capabilities in one. Recognizing this, RWS has been making the semantic AI capabilities of our CCMS a focus for development. In fact, an important new semantic AI release is imminent, but even without it, IDC has recognized us as a leader in content management systems for today's digital workplace, with its growing focus on process automation, chatbots and self-service digital channels.