Understanding bias in machine learning, media and mind
28 Aug 2024
5 mins

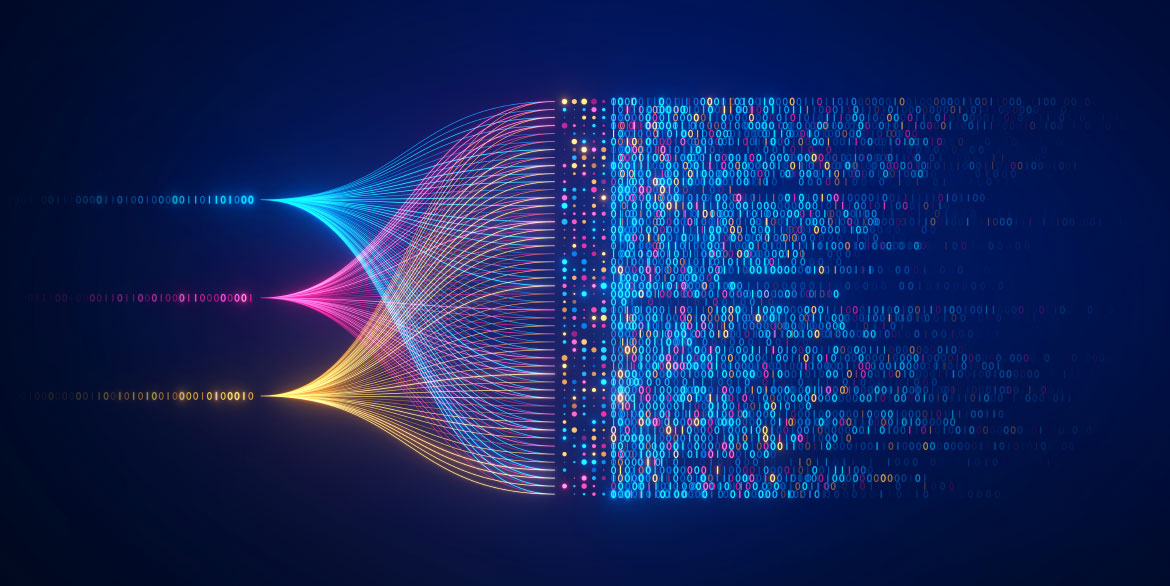
In my daily encounters with human and artificial intelligence, I’ve come across the term 'bias' in three distinct meanings that are often conflated. Let’s get them straight.
Bias in machine learning
In the narrow domain of machine learning, the term 'bias' refers to the measured distance between the predictions of an AI model and the factual data that the model is trained on. As a concrete example, imagine we train an AI system to forecast the weather, and it consistently predicts temperatures that are on average 10 degrees lower than reality. Such a model has bias for predicting lower temperature values. This bias is measured against the real data we have from existing temperature observations. It refers to a systematic statistical deviation measured against the existing data.
Bias in the media and AI ethics
In the current discourse on AI, journalists, marketers, and the burgeoning AI ethics community use the word 'bias' to refer to the deviation between the model predictions and a coveted perfect world. It’s measured not against existing data but against imaginary data of an ideal world we aspire to live in: a world free from prejudices, inequality and discrimination. A world where AI algorithms treat every person as equal no matter race, gender and background. Humanity is not there yet, but we want AI to be.
When AI predictions exhibit bias, this inevitably gives rise to indignation, fear, and oftentimes public outcry. Consider a scenario where a qualified job candidate is rejected merely because of their gender. When such injustice stems from human decision-making, it feels unfair. When the decision is produced by an AI algorithm, it feels unbearably unjust. The reason for this asymmetrical reaction is simple: humans can accept that other humans may be unfair, but they expect machines to always be objective and show no bias. Paradoxically, the human propensity for trusting machine objectivity more than the one of humans is called automation bias and is just one example of an uncomfortably long list of cognitive biases that characterize the human species.
Bias in psychology
These cognitive biases are adaptive features that evolved to help us make better and faster decisions through mental shortcuts and effective sampling of the available information. As useful as they can be, such biases distort our perception and cause us to make non-objective decisions. For instance, salience bias misleads us to allocate money to solving the most prominent problems and ignore more unremarkable, but equally important, issues. Availability bias makes us overestimate the danger of flying on a plane and underestimate the risks of using cars, even though the latter kill far more people annually. And, ironically perhaps, we have a bias called the bias blind spot which makes us perceive ourselves as having fewer cognitive biases than the people around us.
In addition to the cognitive mental shortcuts used to speed up decision making, humans have socio-cultural biases generated by cultural stereotypes and personal experiences. Examples of biases in this category are gender bias and stereotyping. AI models trained on data containing socio-cultural biases can inadvertently replicate them, leading to unfair and discriminatory outcomes.
Should training data be debiased?
Cognitive and socio-cultural biases can infiltrate training data in numerous ways. Firstly, human annotation may introduce bias. Secondly, the data collection methodology can be flawed and lead to an unbalanced, biased dataset. Thirdly, the data may contain historical bias, reflecting outdated societal values.
AI systems trained on such biased data can amplify these biases. This raises an important question: should AI datasets mirror our imperfect reality, or should we create training datasets that adjust for human psychological biases and rectify societal injustices? Most people would agree that the latter approach is preferable: training data must be debiased. However, two factors make this task very challenging.
Bias can be subjective
Socio-cultural bias is not an absolute phenomenon. Bias is perceived differently by various individuals and across different time periods. What may be considered innocuous in one culture may be offensive in another. A decision deemed acceptable today might trigger public outrage in the future. Bias perception also varies among individuals from the same culture due to differences in personal experiences and sensitivities. Even within the same individual, sensitivity to bias can differ depending on their physical and psychological state at any given moment.
Lack of explainability in AI
The situation is further complicated by the lack of AI explainability in AI, which refers to the difficulty in understanding how AI systems arrive at their decisions. Current deep-learning AI systems are sub-symbolic and operate as black boxes, concealing the logic of their learning processes. There are numerous instances where AI behaves predictably until it is probed to reveal startlingly divergent decision-making process than the one humans would follow.
A notable example comes from an AI-powered recruitment tool that was discovered to discriminate against women for technical positions such as software engineering. Even though all gender information was meticulously removed from the training data, the algorithm systematically downgraded women's resumes for such jobs. It did so by identifying subtle language patterns in female and male language usage and favoring male resumes where words like 'executed' or 'captured' were more prevalent, thus signaling recruitment success.
This comes to show how an AI algorithm can unintentionally learn bias from a variety of different sources. Everything from the data used to train it, to the people who are using it, and even seemingly unrelated factors, can all contribute to AI bias.
The emergence of AI ethics
Amidst this complexity, the field of AI ethics emerges to tackle the ethical implications of AI deployment. As technology brings new opportunities, it also necessitates vigilance against unintended consequences. Failure to debias as well as overcompensating for bias can bring equally harmful consequences, exemplified by Google Gemini's well-intended diversity feature that inadvertently misrepresented the race of historical figures. Over-debiasing can become a form of bias itself.
People are biased. Training data is biased in part because people are biased. AI algorithms are biased when they are trained on biased data. Only by confronting these biases—both within ourselves and within our machines—can we steer AI towards a future aligned with our aspirations for a fair and just world.
Read our blogs relating to ethical AI training and development, and learn how you can reduce bias in your future AI innovations.