Solving customer challenges with data services for AI
23 Nov 2021
6 mins

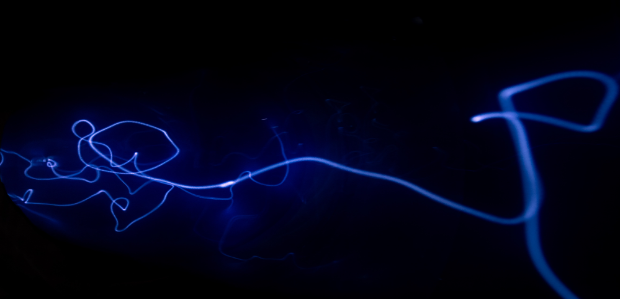
Although AI is currently one of the most discussed topics, it is not always entirely clear what is behind it. For some, AI is a technology shrouded in mystery. Others expect AI to solve all business challenges without the need for costly human resources. However, when you look deeper, you realize that AI is a technology-based science that has been researched for years, but only recently has it been successfully put to productive use.
In a nutshell, it is about using algorithms and lots and lots of data to enable an AI system to analyze new or lots of data, to recognize content and correlations, and to support decision-making processes or perform defined actions autonomously.
And this is where we come to the topic of our interview. We welcome Alejandro Garcia. He is Program Director at RWS and has been involved with Data Services for AI for many years.
Alejandro, perhaps you could briefly introduce yourself?
Hello and great to meet you today! My name is Alejandro Garcia, I am a Systems Engineer and live in Rosario, Argentina. I started my career in the IT industry 20+ years ago in different Software Development factories as a programmer, then team leader and business analyst. I joined RWS 11 years ago where I had the opportunity to grow professionally and expand my knowledge in different areas , like Machine Learning where my focus has been ever since.
Can you please define Data Services for AI at RWS? Can you describe it more precisely or narrow it down?
Data Services for AI means for me a great opportunity to be part of the biggest digital transformation we ever had, where I can provide tangible value helping clients improve the global consumer experience, as well as to create platforms and products more visible and accessible to all.
What are typical requirements that clients formulate for data services and how do you go about it?
There is a lot of variation in the tasks scope and requirements as it really depends on the AI model, it´s goals, and how to train it with data. However, I have found common that clients come to us with requests that fall in Data Collection, Data Annotation or Data Validation groups of services. Clients typically share set of languages, number of resources, resources profile, task definition, volumes and format of the files, expected outputs and productivity (when possible), legal and / or technology requirements. In any case, sometimes, as the experts, we also help the clients define the requirements based on general project information they provide.
What are the first steps when you want to start an AI project?
The first steps definitely revolve around getting to know the client and their goals as much as possible. Some of our clients can find themselves in the early stages of their machine learning deployment journey and we can provide significant added value on top of providing just annotated data – whether it’s through providing end-to-end services or simply consulting on system design. After all, it’s very easy to get a whole lot of right answers for the wrong question, or to design a system that is prone to various biases or blind spots. With more experienced clients, this conversation would be more about technical aspects and optimization of effectiveness and accuracy.
Knowing the goals and context means that we can now effectively prepare the production pipeline for annotating the data set. This phase involves several steps, such as instruction preparation, cleaning the data (if necessary) and setting up the labelling ontology, all of which ensure that the AI will understand the information it is being trained on and will be able to recognize meaningful patterns in the data.
So if I understand that correctly, it's kind of about preparing the learning material for the AI. So in this sense, RWS would be something like the creators of learning material for AI?
That’s exactly right! We are the tutors to the machine learning model and the trained data set is the textbook it is learning from. Our responsibility is to make sure that it gets good grades in the tests ?These metaphors do not fully represent the complexity and the limitations of machine learning but are still useful to understand the fundamentals of data annotation and its role in machine learning.
Does it actually make a difference in which languages the training data is prepared?
There are some exceptions to the rule, but a machine learning model is typically only useful for the one specific language it has been trained on. There are tasks and models which you could consider language agnostic (usually involving audio or image inputs), but natural language processing is one of the hot spots for AI and it is definitely one of the areas we specialize in, given the vast experience and market position in localization.
How will requirements change in the future and how is RWS adapting to them?
I think many companies will expand (some of them already doing it) their Data Services needs from English only or just a few common languages, to long tail languages and even dialects. This will facilitate reaching more markets and providing services to users who currently miss the benefits of AI depending on where they are located. There will also be standardization and automations to collect, annotate, validate data, as well as more systematic approach to define and measure quality for Data Services tasks.
Thank you for this interesting insight into the world of Data Services! I am sure that this area will become even more important in the future.