Your guide to using artificial intelligence for business growth
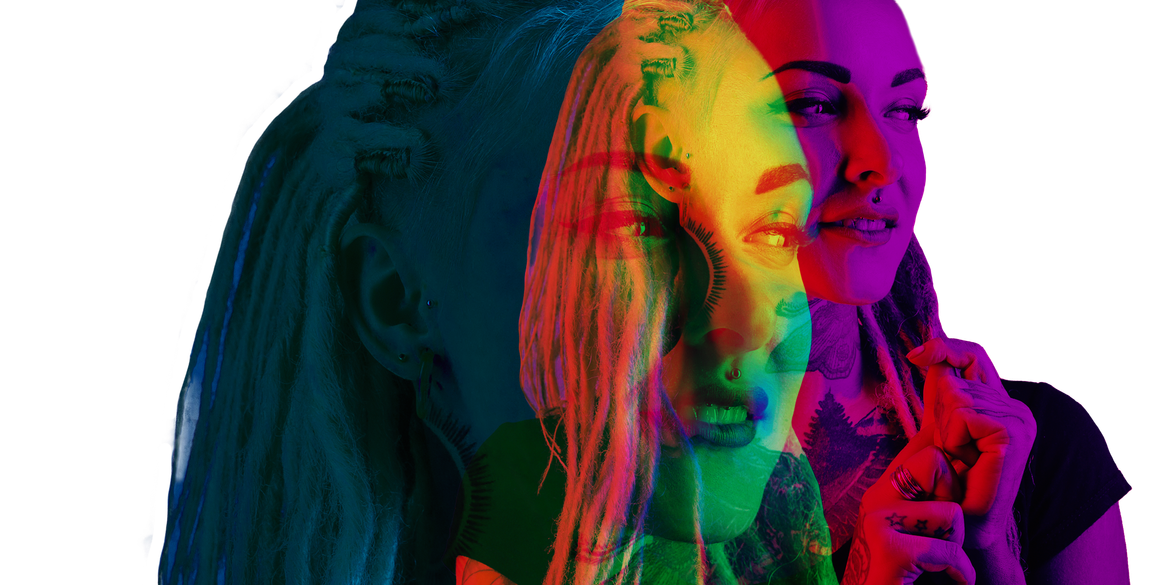
With its ability to automate tasks and provide valuable insights not always obvious to humans, it’s no surprise that business leaders are turning to artificial intelligence (AI) to drive innovation and business advantage. But the AI market is so complex, it can be hard to know where to start or how to avoid the many pitfalls that lie in wait if you try to run before you can walk.
Hence this guide to using artificial intelligence for business growth. We cover everything from fundamental AI concepts to the most recent advancements in AI, as well as all stages in the AI journey from exploring and building AI to common use cases that can propel your business forwards.
Why listen to RWS on this subject? Because we’re not new to AI. Our roots in machine translation stretch back to the 2000s, and today we also develop AI solutions for other businesses and support the world’s leading AI companies with trusted AI training data services. Long before ChatGPT got everyone buzzing about AI, we’d been integrating AI into our own technologies – and we continue to do so as the AI landscape rapidly changes. From strategy to application, there’s no area of AI that we’re not involved in.
So let’s dive in with an introduction to some AI concepts and some different types of AI.
What is artificial intelligence?
AI is a broad term used for a range of technologies that mimic various human cognitive functions, able to perform tasks that would typically require human intelligence. An AI model can perform tasks without explicit step-by-step instructions, working with uncertain or incomplete information and generalizing from known scenarios or data to previously unseen scenarios or data.
Machine learning
Machine learning is a pathway to AI – a method of developing an AI model. Machine learning models, a subset of AI systems, use statistical techniques to learn what to do for themselves, instead of being told what to do through explicit programming. They learn by being trained on large volumes of data, mainly through a mix of supervised, unsupervised and reinforcement learning. Read our blog on how AI is trained for more on this topic.
Deep learning
Deep learning is a subset of machine learning methods powered by multi-layered artificial neural networks – that's the 'deep' component – inspired by the human brain's own biological neural networks. The layers enable the model to train on a set of features and then use that knowledge to recognize, or 'classify', new inputs. Deep learning methods are applied in a wide range of AI applications, including facial recognition and natural language processing.
Natural language processing
Natural language processing (NLP) combines computational linguistics with statistical and machine learning models (especially deep learning) to process text or voice data and deal appropriately with its semantics (or meaning), including its sentiment. This is what gives machines the ability to derive meaning from human language in a valuable and structured way – and the ability to create text or speech.
Generative AI
Another application of deep learning, generative AI (GenAI) models can create new data such as text, images or music based on patterns in their training data.
Exploding into our consciousness with the release of ChatGPT, these models are quickly being adopted by businesses for a wide range of applications. This rapid adoption has raised concerns about GenAI, including its security and privacy, tendency to hallucinate, and the way its reliability or usefulness can be affected by bias and other data quality issues. To maintain the trust of their stakeholders, businesses developing and using GenAI need to take the principles of responsible AI seriously.
Large language models
A type of GenAI focused on human language, large language models (LLMs) respond to text prompts in a human-like way. OpenAI's GPT, Google’s Gemini and Meta’s Llama are well-known examples, with many more emerging as demand grows.
LLMs can write essays (or poetry, or any content you like) and answer questions. Though they learn and use language very differently from us, their ability to mimic our interactions makes LLMs valuable tools for organizations looking to improve customer service and engagement at scale. You can build LLM-powered chatbots or virtual assistants (something we’ve done, for example, to provide product support for our Trados portfolio) to answer queries, schedule appointments, place orders, generate reports and much more.
But as a type of GenAI, LLMs have the same vulnerabilities, and need expert human oversight to ensure their responsible use.
Getting started with AI
That’s the basics of artificial intelligence wrapped up. These technologies offer potentially transformative capabilities to solve complex problems, optimize operations and create more personal and engaging experiences for customers. But before you can take advantage of them, you need to know where to start. The first step in deciding where and how to use artificial intelligence is to explore and understand which areas of your business will benefit from AI automation and insights.
Look for areas that are data- or processing-heavy, such as customer service, marketing automation, fraud detection, predictive analytics or inventory management. Focus on tasks that are particularly time-consuming or prone to human error – these are prime opportunities for AI implementation.
The next step is to develop an AI strategy. This involves designing a plan with clear goals and objectives, as well as actionable steps to reach them. Your plan should also take into account the types of data you have (or need) and the resources available for development.
Through this entire process – from exploring opportunities to building a plan – it’s important not to pursue innovation for innovation’s sake. It’s hugely tempting to jump on the AI bandwagon simply to be seen as progressive, or because the hype makes it seem oh-so-easy to realize the benefits. This is almost never the case. Urgent, unchecked AI advancement risks exposing your organization or your customers to immature solutions that may do real damage to your reputation. To make the most of AI’s potential, it’s important to distinguish between hyper-acceleration and purposeful innovation – and always pursue the latter.
Building your AI model
With a plan in place, it’s time to start building your AI model. If you have an in-house AI team, they may be able to handle the work. But if not, there are tools and services available to help you get started, including cloud-based machine learning platforms and pre-built AI models – commercially available or open-source LLMs, for example – that you can fine-tune using your organization’s data.
Or – as with any software requirement – you can outsource AI development entirely. Look for a machine learning engineering team with a successful record of AI development, and the strategic outlook to advise you on what AI realistically can and can’t achieve for you, in the context of your specific needs.
The central role of data
Data is the foundation of every AI application. Being trained with specific data is what enables your AI-powered systems to recognize the right inputs, deliver the right responses and progressively support new and more complex use cases.
It takes huge amounts of data to train an AI model – especially if it’s a large language model (the clue is in the word ‘large’) – and the quality of the data is critical to the ultimate success of the model. Never has the adage ‘garbage in, garbage out’ been more true, than when it comes to AI training and data quality. If your AI application has learned what to do based on poor-quality data, or data that’s not quite fit for your particular purpose, it won’t perform well when it matters.
In fact, preparing quality data for AI training is so important, it can consume up to 80% of AI project time. Whether you’re doing it yourself or outsourcing to a data partner, it’s a significant part of the process that needs to be properly budgeted for. For a detailed overview of what AI training data is, how it’s prepared and used in the AI training process, and what good quality training data looks like, read our blogs: How AI is trained: the critical role of AI training data and Ensuring high-quality AI data: the foundation of AI success.
Ensuring that AI is ethical and trustworthy
Quality data is critical for reducing the chance of inaccurate, irrelevant or biased outputs arising from issues in the training data. Bias – both in the data and in the AI model’s output – is particularly insidious and difficult to detect and mitigate, so it’s a major source of mistrust in AI.
Bias is not the only AI challenge that has ethical implications. Other common AI challenges that reflect important societal concerns are privacy, transparency and sustainability. For insight on how to tackle these, along with more practical AI training challenges such as data acquisition and keeping pace with change, see our blog: Seven common AI training challenges and how to address them.
The prevalence of ethical challenges in AI development and use is why there has been so much focus given to the topic of responsible AI, and why it’s important to pay attention to the work of the organizations developing standards to ensure that AI applications can be trusted. In December 2023, for example, the world’s first AI management system standard, ISO/IEC 42001, was published for this purpose.
Using AI to expand your global reach
There are as many ways to use AI for business growth as there are examples of AI applications. Any improvement in efficiency or insight delivered by AI can ultimately be seen as contributing to growth, even if indirectly, by saving time or money that can be invested in growth-driving activities.
But one of the most direct and obvious ways to grow your business with the help of AI is to use it to communicate and engage with new audiences when you expand your business to new markets.
Unlocking global understanding with AI
We know that consumers want to buy from brands that respect their native language and culture. Years before the advent of LLMs, AI-powered machine translation (MT) was already good enough to help businesses translate content more efficiently for their global audiences. Today, the latest advancements in artificial intelligence are being brought to bear to make it even easier for businesses to communicate with customers and others in their preferred languages.
For example, we’re already combining the power of neural machine translation (NMT) with human-trained, AI-powered MT quality estimation (MTQE) and LLMs to take translation efficiency to the next level. This means that the businesses relying on our translation services can get the quality they expect more quickly and cost-effectively – enabling them to redirect time and money to cover more languages, or translate more types of content, or do other things that achieve their growth ambitions.
Businesses translating their own content won’t be left behind by this revolution, either, as long as the translation tools they’re using are enhanced with appropriate AI-powered capabilities.
Doing it intelligently and securely
Beyond the core task of translation, there are other ways to use AI in the end-to-end translation process to improve productivity and help organizations create multilingual content at the pace and scale that their businesses demand. We believe strongly in AI as an empowering tool that will change how people work, but we also believe that human involvement remains critical on many levels. To realize the true value of AI, we need to combine human and artificial intelligence – using the strengths and compensating for the weaknesses of both – to create a true collaboration that results in what we call Genuine Intelligence.
It’s also important to ensure that, whatever AI service or AI-driven tool you use, it doesn’t compromise information security or privacy. It’s no different from choosing a traditional machine translation service. You wouldn’t use a publicly available MT option if there’s a risk that confidential information relating to your business, employees or customers could be captured by the service as AI data and potentially exposed. You should give the same thought to your use of any newer form of AI.
Using AI for better customer service
Besides engaging with people in the language they prefer, businesses have a real challenge to help customers and other stakeholders find the information they need, quickly and reliably.
Even before LLMs entered the fray, businesses were generating digital content at an accelerating pace to meet the needs of an increasingly connected, omnichannel world. The problem is that the more content you have, the more difficult it is to ensure that it’s always up-to-date and accurate, and that just the right information can easily be found when needed.
One solution is to use the right type of content management system – and when you combine this with thoughtfully applied AI innovation, you can supercharge information findability.
For example, for some years we’ve been building semantic AI into Tridion, our intelligent content management platform, to help organizations provide more relevant search results and personalized recommendations to their customers. With the advent of LLMs, we’ve quickly been able to use a technique called ‘retrieval augmented generation’ to enhance information findability even more, enabling you to build a trustable chat function that will reliably draw on approved content rather than hallucinating a possible answer.
LLM-powered content creation capabilities can also be used to help authors – the people in your business who actually write the content that customers rely on – to make content more accessible by being more readable. Once again, the secret to success lies in collaboration between humans and AI, ultimately leading to a better customer experience that enables you to differentiate and grow your business.
Unlock your AI potential
Wherever you are on your AI journey, we can help you go further. We’re leaders in language and in information findability, and pioneers in AI. Learn more here.